Cross-Domain Disentangled Learning for E-Commerce Live Streaming Recommendation.
ICDE(2023)
摘要
E-commerce live streaming as an increasingly popular sales model has generated a significant amount of gross merchandise value (GMV) for e-commerce platforms. Live streaming recommendation systems (LSRS) of e-commerce aim to recommend the most appropriate live channels for users to motivate them to buy products. Existing LSRS methods focus only on the user’s interaction behaviors on the live channel (live domain) while ignoring the user’s behaviors and intentions on the e-commerce product (product domain). As a result, the user’s consistent purchase intentions in the cross-domain are not being fully captured, especially when user present differentiated purchase intentions in the cross-domain. How to disentangle user’s consistent intentions and domain-specific intentions in the cross-domain poses a challenge to the LSRS of e-commerce platforms. In this paper, we present a live channel recommendation method, named eLiveRec, developed for Taobao, one of the largest e-commerce platform in the world. Specifically, eLiveRec employs the disentangled encoder module to learn user’s cross-domain consistent intentions and domain-specific intentions. Then, an adaptive multi-task learning framework is developed to jointly optimize the multiple objectives (e.g., stay time, click goods bag, and click products after entering channel) related to live streaming recommendation. In this way, the performance of live streaming recommendation can be further improved and con-form to standard industry RS paradigms. Extensive experiments are conducted on a large-scale industry dataset collected from Taobao Live platform have been performed. Both online and offline experimental results indicate that eLiveRec consistently outperforms existing state-of-the-art baseline methods.
更多查看译文
关键词
E-commerce,Live streaming,Recommendation systems
AI 理解论文
溯源树
样例
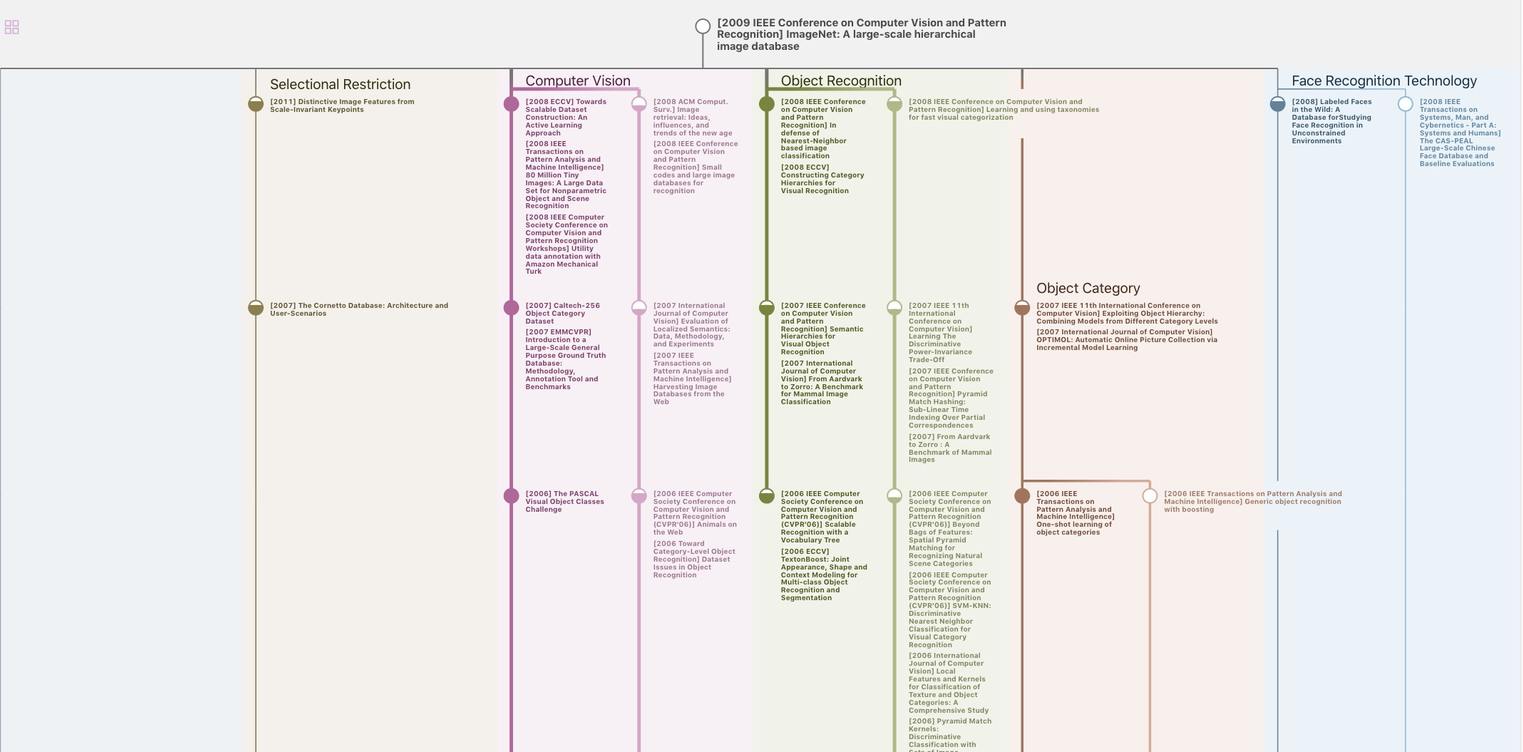
生成溯源树,研究论文发展脉络
Chat Paper
正在生成论文摘要