MIMO YOLO - A Multiple Input Multiple Output Model for Automatic Cell Counting
2023 IEEE 36TH INTERNATIONAL SYMPOSIUM ON COMPUTER-BASED MEDICAL SYSTEMS, CBMS(2023)
摘要
Across basic research studies, cell counting requires significant human time and expertise. Trained experts use thin focal plane scanning to count (click) cells in stained biological tissue. This computer-assisted process (optical disector) requires a well-trained human to select a unique best z-plane of focus for counting cells of interest. Though accurate, this approach typically requires an hour per case and is prone to inter- and intra-rater errors. Our group has previously proposed deep learning (DL)-based methods to automate these counts using cell segmentation at high magnification. Here we propose a novel You Only Look Once (YOLO) model that performs cell detection on multi-channel z-plane images (disector stack). This automated Multiple Input Multiple Output (MIMO) version of the optical disector method uses an entire z-stack of microscopy images as its input, and outputs cell detections (counts) with a bounding box of each cell and class corresponding to the z-plane where the cell appears in best focus. Compared to the previous segmentation methods, the proposed method does not require time- and labor-intensive ground truth segmentation masks for training, while producing comparable accuracy to current segmentation-based automatic counts. The MIMO-YOLO method was evaluated on systematic-random samples of NeuN-stained tissue sections through the neocortex of mouse brains (n=7). Using a cross validation scheme, this method showed the ability to correctly count total neuron numbers with accuracy close to human experts and with 100% repeatability (Test-Retest).
更多查看译文
关键词
Deep Learning,Neuron Counts,YOLO,Microscopy,Stereology
AI 理解论文
溯源树
样例
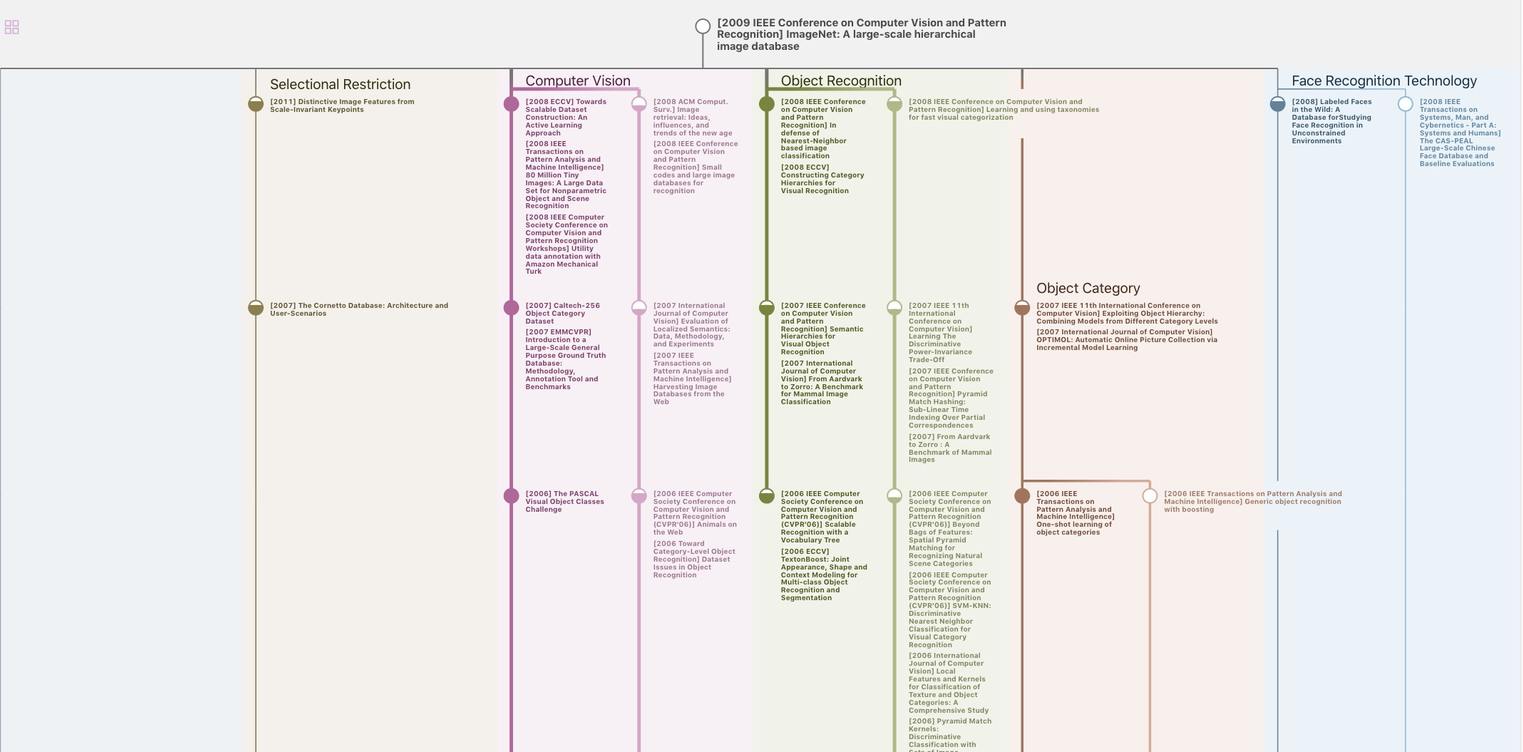
生成溯源树,研究论文发展脉络
Chat Paper
正在生成论文摘要