SAS-UNet: Modified encoder-decoder network for the segmentation of obscenity in images.
CCGridW(2023)
摘要
Obscene content on online platforms can be harmful to society in several ways. Firstly, it can negatively impact the mental health of individuals who view or are exposed to it, particularly children and vulnerable individuals. Secondly, it can lead to the spread of illegal content such as child pornography or revenge porn, which can cause significant harm to the victims involved. Lastly, it can create a toxic and unsafe online environment, where cyberbullying, harassment, and hate speech thrive, ultimately leading to social division and harm to the community. As a result, it is important to promote the responsible and respectful use of online platforms to prevent such harm. Therefore, it is important to segment such obscene regions present in images across social media platforms. To deal with the issue, we have proposed a modified encoder-decoder-based technique entitled sandglass-block encoder with attention skip and swin-based bottleneck (SAS-UNet) for the segmentation of obscenity in nude/obscene images. Our proposed method SASUNet incorporates the usage of a sandglass block in the encoder section of U-Net, a swin transformer in the bottleneck, and CBAM inclusion at the skip connection. The proposed model is trained and validated with the segmented obscene image (SOI) dataset. The collected obscene images were segmented precisely to build a binary mask of all the obscene images. The proposed SAS-UNet achieved a precision of 94.30%, 93.22% recall, 92.31% of mDC value, and 91.42% of mIOU value which outperformed the existing algorithms.
更多查看译文
关键词
Obscenity, deep learning, image classification, U-Net, swin transformer
AI 理解论文
溯源树
样例
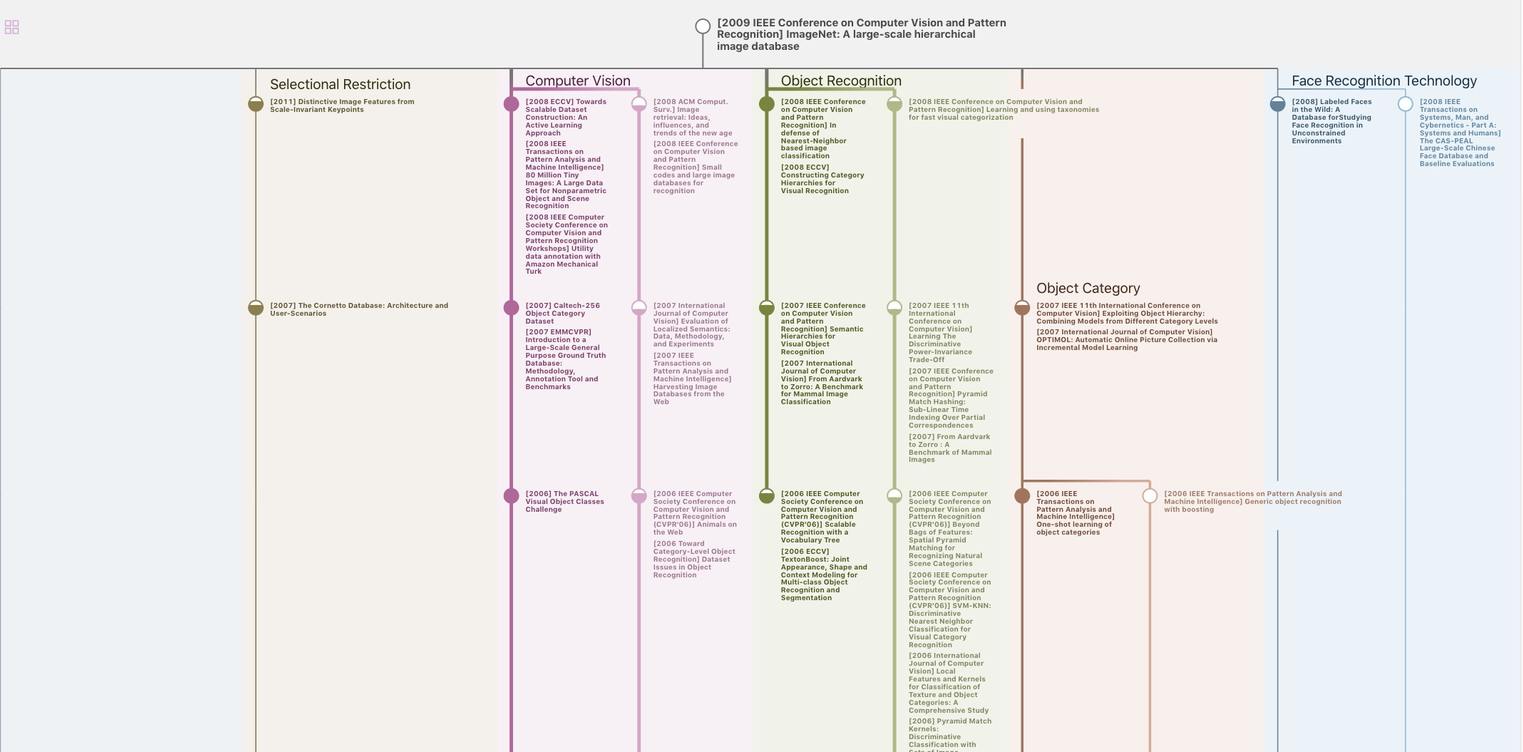
生成溯源树,研究论文发展脉络
Chat Paper
正在生成论文摘要