Information Fusion-based Cybersecurity Threat Detection for Intelligent Transportation System.
CCGridW(2023)
摘要
Intelligent Transportation Systems (ITS) are sophisticated systems that leverage various technologies to increase the safety, efficiency, and sustainability of transportation. By relying on wireless communication and data collected from diverse sensors, ITS is vulnerable to cybersecurity threats. With the increasing number of attacks on ITS worldwide, detecting and addressing cybersecurity threats has become critically important. This need will only intensify with the impending arrival of autonomous vehicles. One of the primary challenges is identifying critical ITS assets that require protection and understanding the vulnerabilities that cyber attackers can exploit. Additionally, creating a standard profile for ITS is challenging due to the dynamic traffic pattern, which exhibits changes in the movement of vehicles over time. To address these challenges, this paper proposes an information fusion-based cybersecurity threat detection method. Specffically, we employ the Kalman filter for noise reduction, Dempster-Shafer decision theory and Shannon’s entropy for assessing the probabilities of traffic conditions being normal, intruded, and uncertain. We utilised Simulation of Urban Mobility (SUMO) to simulate the Melbourne CBD map and historical traffic data from the Victorian transport authority. Our simulation results reveal that information fusion with three sensor data is more effective in detecting normal traffic conditions. On the other hand, for detecting anomalies, information fusion with two sensor data is more efficient.
更多查看译文
关键词
Information fusion,Cybersecurity,Intelligent Transport Systems,Threat Detection
AI 理解论文
溯源树
样例
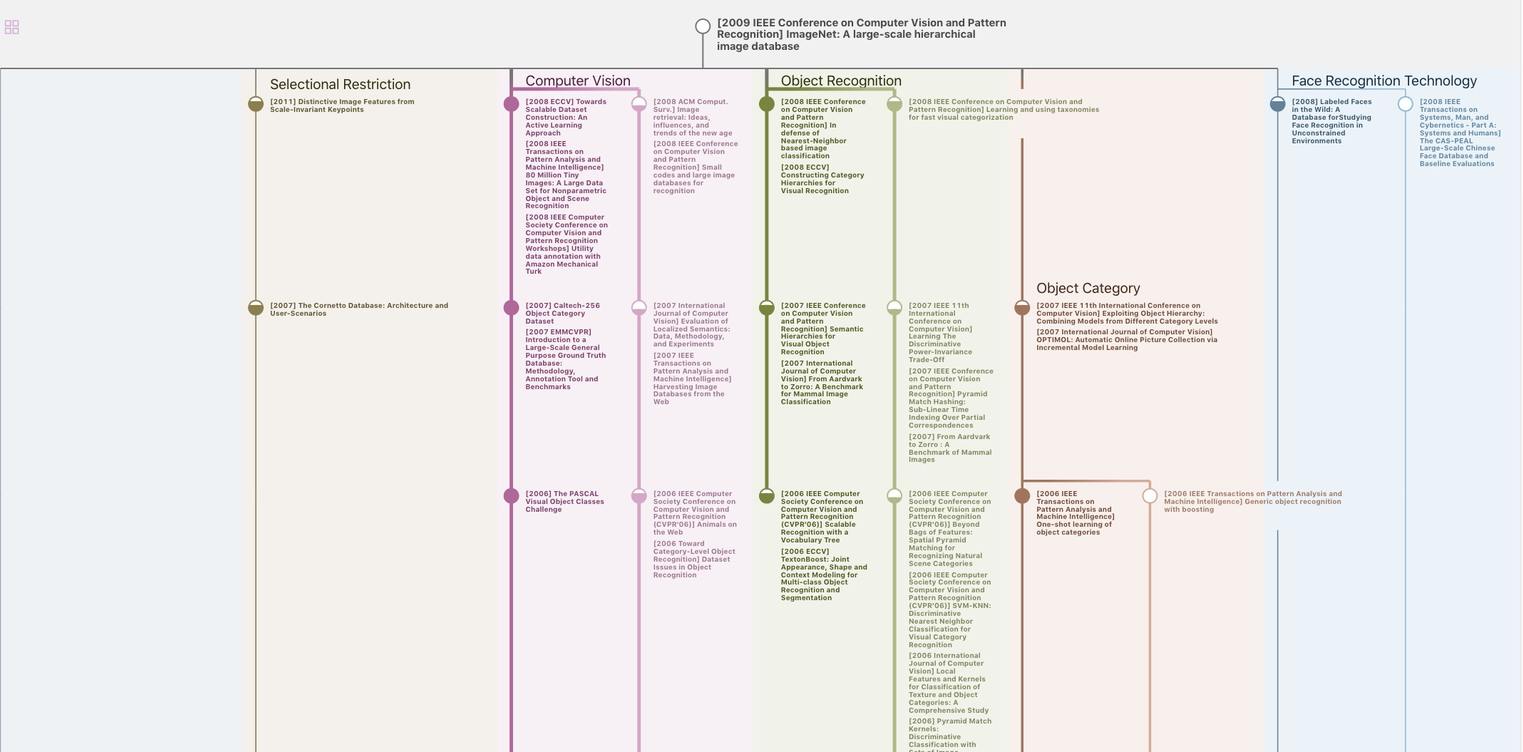
生成溯源树,研究论文发展脉络
Chat Paper
正在生成论文摘要