PrivNUD: Effective Range Query Processing under Local Differential Privacy.
ICDE(2023)
摘要
Local differential privacy (LDP) has been established as a strong privacy standard for collecting sensitive information from users. Although it has attracted much research attention in recent years, the majority of existing works focus on applying LDP to frequency distribution estimation for each individual value in a discrete domain. This paper concerns the important range queries involving multiple discrete values. Till now, only a few works target this problem. They all rely on the B-ary tree to construct a uniform and hierarchical decomposition, so as to decrease the error when answering large range queries. However, the uniform splitting manner ignores the properties of decomposed sub-domains and processes them equally without preferences, which leads to significant performance penalty.In this paper, we tackle the problem head on: our proposal, privNUD, is a novel domain hierarchical decomposition mechanism. It dynamically decomposes each domain with a tailored granularity into some sub-domains, which sensitively considers the potential chances to answer one range query. The issue of granularity is carefully analyzed for better performance. It also can smartly prune the sub-domains with small frequencies. Besides, an adaptive user allocation technique is designed to dynamically decide the scale of users that are involved in each sub-domain’s frequency estimation. Extensive experiments using real and synthetic datasets demonstrate that privNUD achieves significantly higher result accuracy compared to the up-to-date solutions.
更多查看译文
关键词
local differential privacy,range query,hierarchical decomposition
AI 理解论文
溯源树
样例
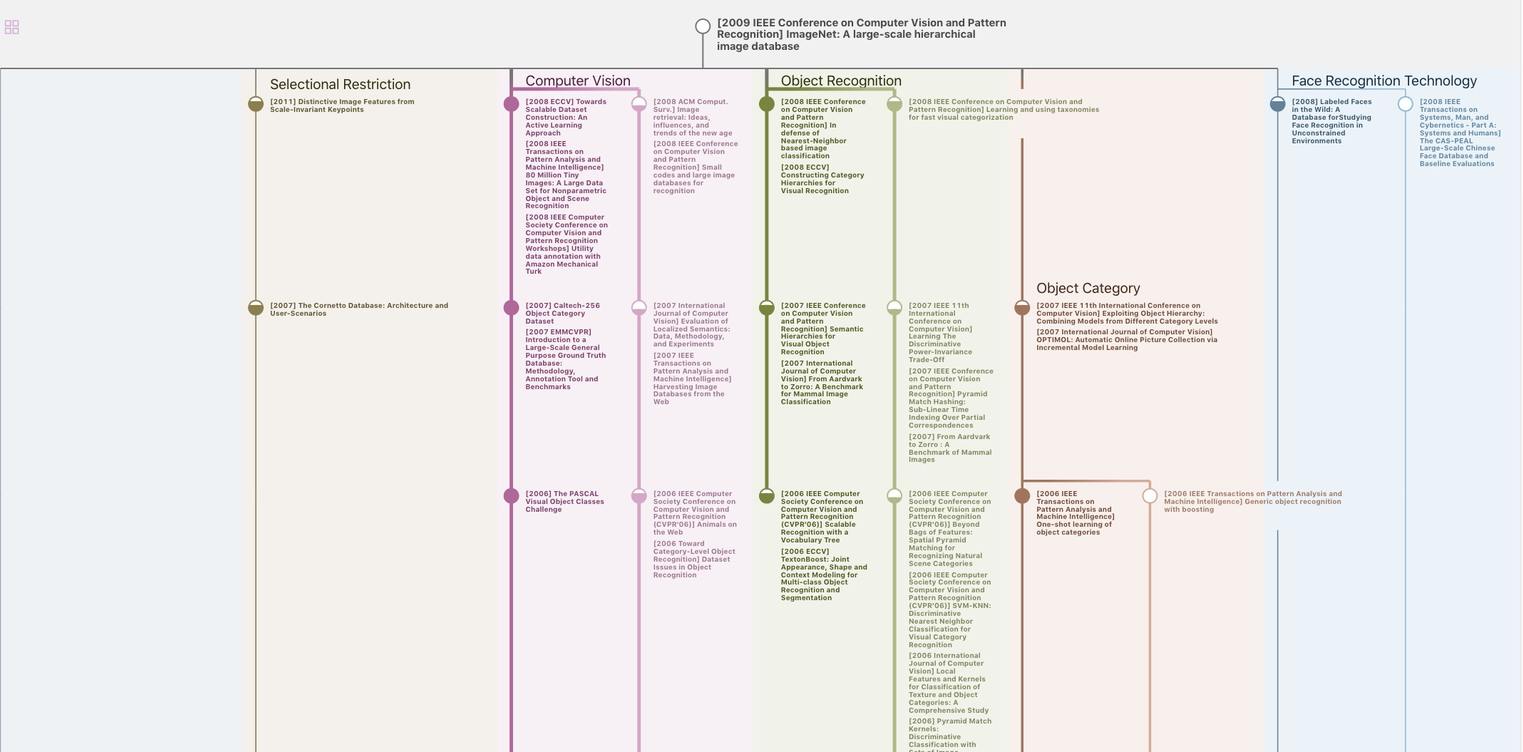
生成溯源树,研究论文发展脉络
Chat Paper
正在生成论文摘要