Multi-compartment structure optimizes the deep learning network from the biophysical perspective.
I2MTC(2023)
摘要
Artificial intelligence makes significant progress attributing to the deep learning adopting neurophysiological strategies. The learning process in deep learning is resemble to that in the brain. However, the traditional nodes with a uniform structure form are much simpler than the biological neurons with branches in the brain. To investigate whether realistic neurons with multi-compartment structures could optimize the deep learning algorithm, a three-layer deep learning network with multi-compartment neurons is designed. The results show that three-compartment neuronal structure with apical dendrites makes the network perform a better learning effect compared with the two-compartment and single-compartment neuronal structure on coding MNIST database. The apical dendrites separate the feedforward and feedback signals, which provides a way for solving credit assignment problem. In addition, the introduction of apical dendrites increases the correlation between the loss of hidden and output layers. The effect of axial conductance from the apical dendrite to soma and the conductance related to spike frequency adaptation (SFA) current is quantified. The results show that greater axial conductance generates higher accuracy. And the adaptation conductance reaches the highest accuracy at around 97.2% when the interval is in 1mS/cm(2)similar to 3.4mS/cm(2). This work contributes to a better understanding of the function of multi-compartment structure in deep learning algorithms and provides insight into constructing a deep learning network with biophysical characteristics.
更多查看译文
关键词
Multi-compartment neuron,Deep learning,Correlation,Artificial neural networks
AI 理解论文
溯源树
样例
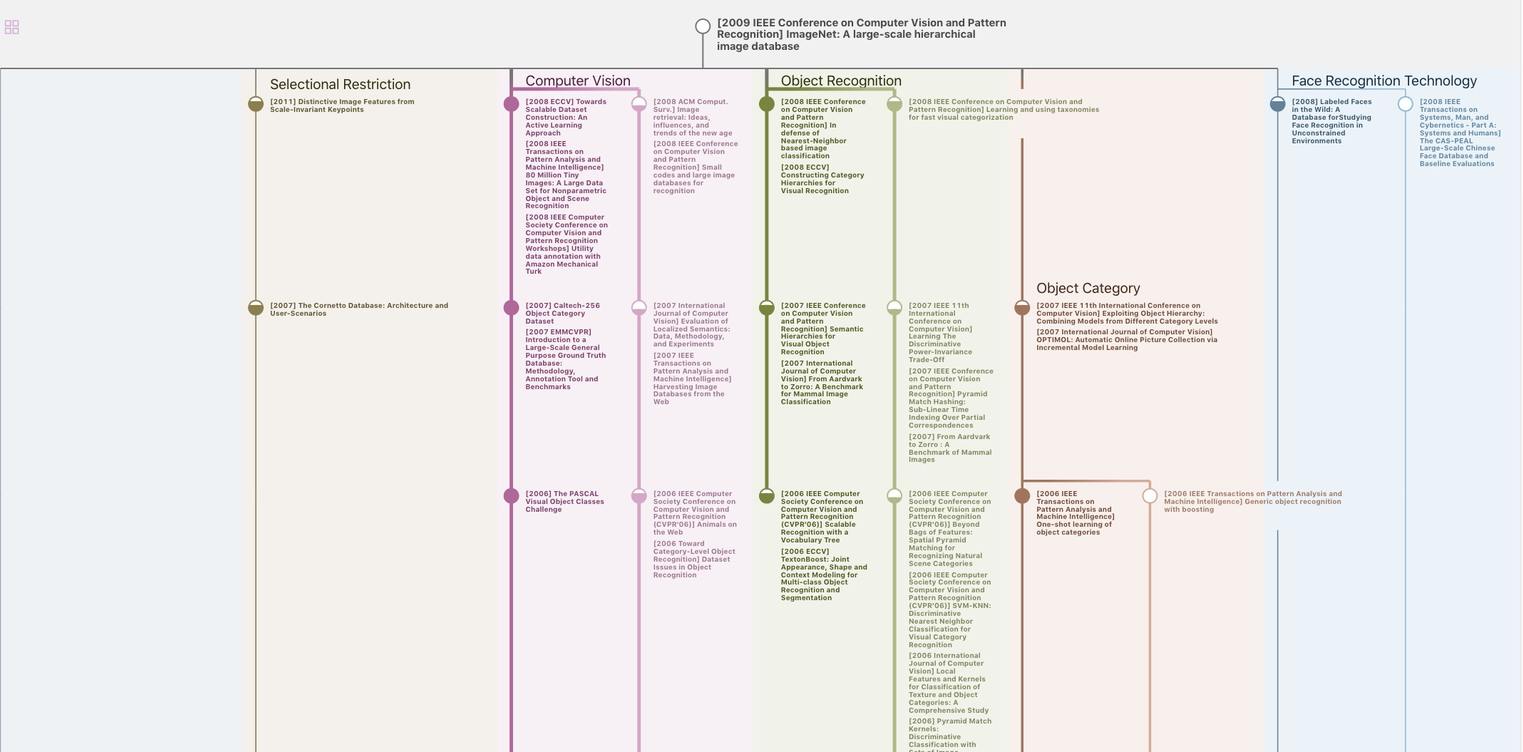
生成溯源树,研究论文发展脉络
Chat Paper
正在生成论文摘要