Multiscale Deep Attention Reinforcement Learning for Imbalanced Fault Diagnosis of Gearbox under Multi-Working Conditions.
2023 IEEE International Instrumentation and Measurement Technology Conference (I2MTC)(2023)
摘要
Multi-operating conditions and skewed class data distribution bring great challenges to gearbox fault diagnosis. This paper presents a new multiscale deep attention reinforcement learning (MDARL) approach for imbalanced fault diagnosis of gearbox. Specifically, class deviation degree is defined to build the environment reward strategy, and then an imbalanced classification Markov decision process (ICMDP) is established to realize the learning of fault diagnosis policy. Based on the deep Q network (DQN) algorithm, a multiscale convolutional attention network (MCAN) is designed as the network structure of the DQN agent by using multiscale convolution, channel attention, and residual network, to enhance the model's feature learning ability. Finally, imbalanced fault diagnosis of gearbox is effectively realized via the interactions between the agent and data environment, and the interaction obeys the ICMDP. Experiment results show that the presented approach can achieve an accuracy of over 99.0%, and has strong stability for imbalanced gearbox fault diagnosis under multi-working conditions.
更多查看译文
关键词
deep reinforcement learning,data imbalance,gearbox,fault diagnosis,multiscale convolution
AI 理解论文
溯源树
样例
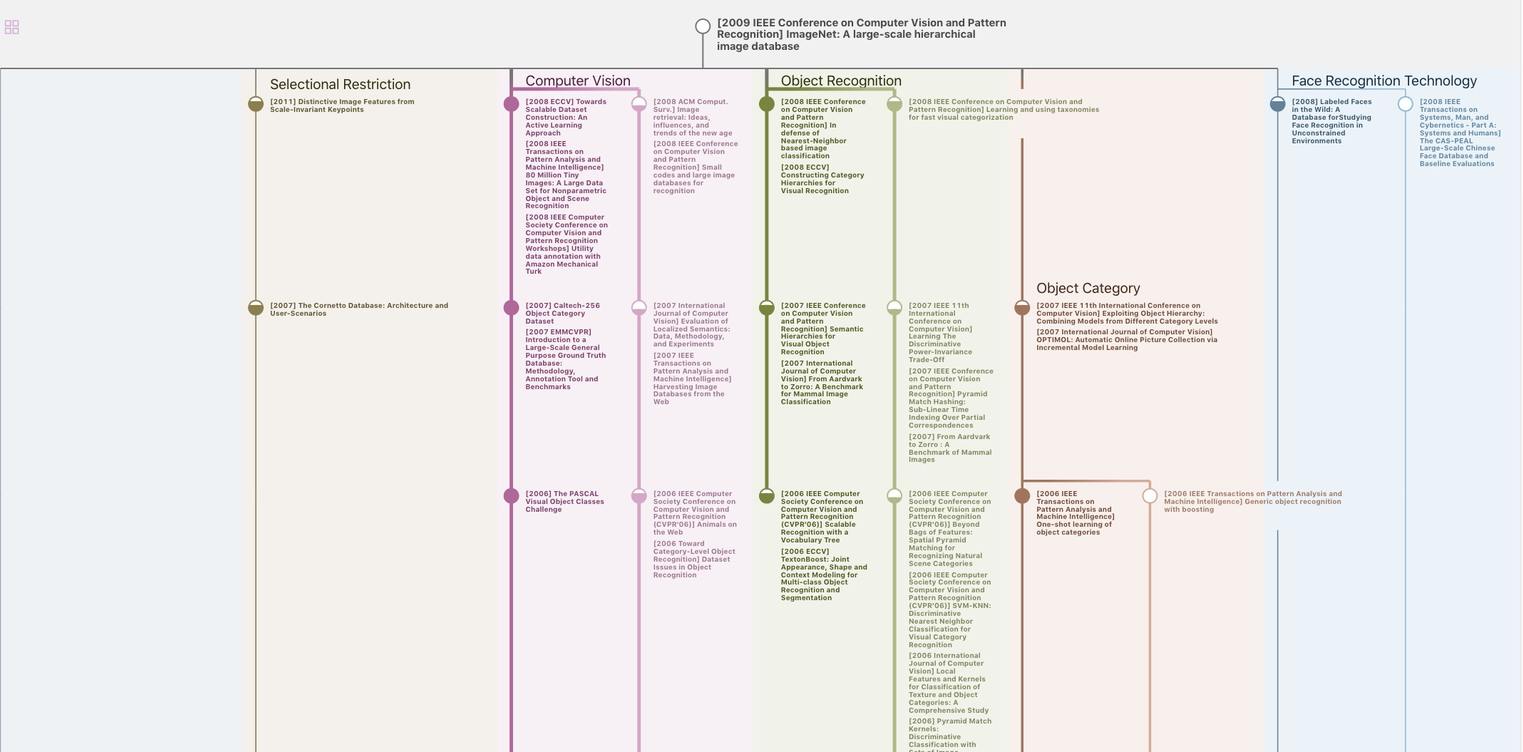
生成溯源树,研究论文发展脉络
Chat Paper
正在生成论文摘要