Battery Remaining Useful Life Prediction Supported by Long Short-Term Memory Neural Network.
I2MTC(2023)
摘要
The rise of renewable energy and electric vehicles has led to enormous growth and development in the field of lithium-ion batteries. Ensuring long-life and safe operation of these batteries requires accurate estimation of key parameters such as state of charge, state of health (SoH), and remaining useful life (RUL). In this paper, a long short-term memory neural network (LSTM NN) is presented for RUL prediction. Furthermore, the predictors used are discussed in detail, and a comparison between the two models is presented. The network has been trained and tested on a substantial dataset of 124 batteries, aged under various fast charging conditions, and published by the Toyota Research Institute in collaboration with MIT and Stanford. Despite their vastly different cycle lives, the proposed LSTM NN structure has performed very accurate RUL prediction for all tested cells.
更多查看译文
关键词
lithium-ion batteries,machine learning,remaining useful life (RUL),neural network,aging
AI 理解论文
溯源树
样例
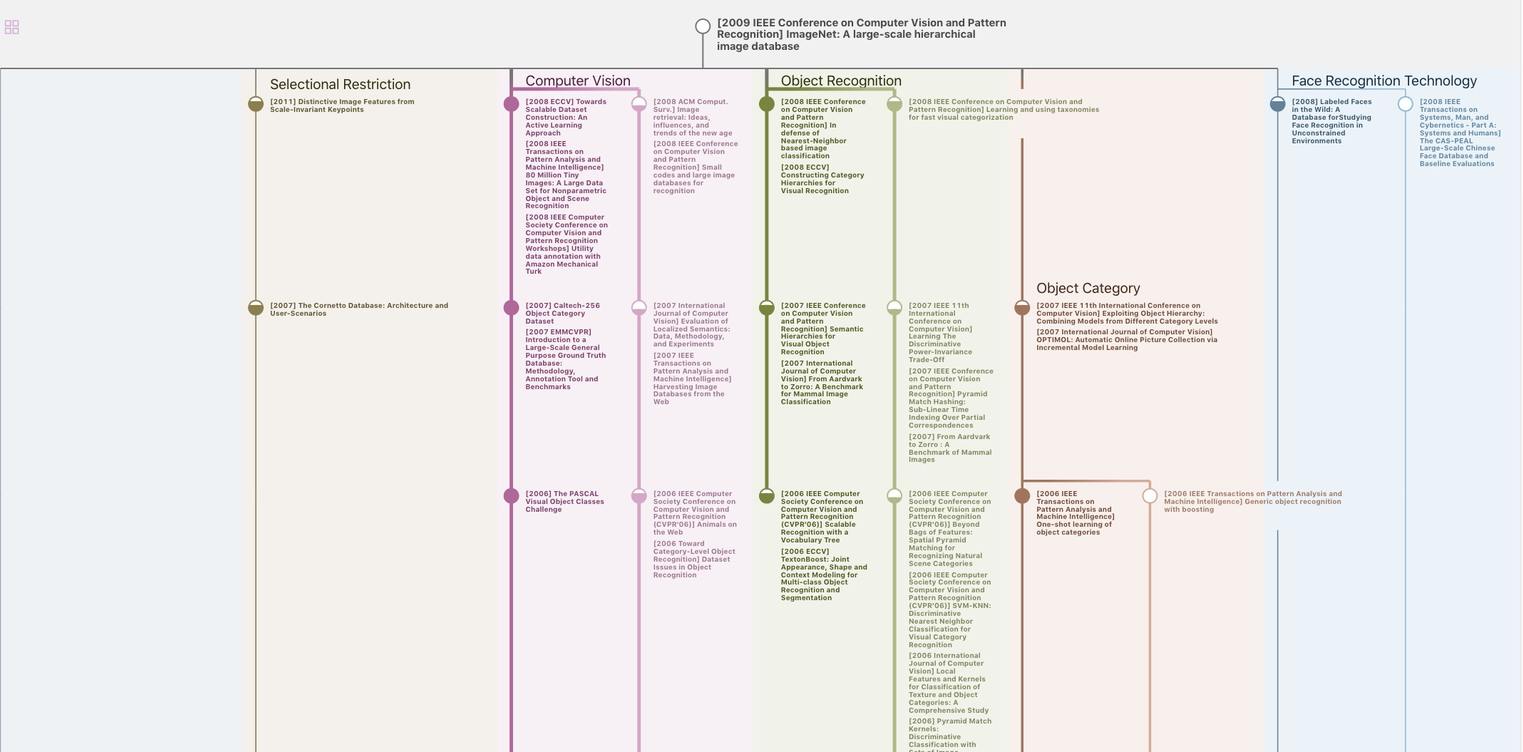
生成溯源树,研究论文发展脉络
Chat Paper
正在生成论文摘要