OPFsemble: An Ensemble Pruning Approach via Optimum-Path Forest.
IWSSIP(2023)
摘要
One of the main drawbacks of classification and machine learning algorithms is selecting the learning models that best fit the problem domain. A common approach to tackle this issue comprises ensemble learning, i.e., several different models are employed to solve a given task, and the output consists of a pool of these models’ outcomes. Nevertheless, such an approach is computationally costly and demands a strategy to prune similar models and keep the variability in the results. A general solution comprises clustering algorithms, which, on the other hand, usually require prior knowledge of the problem to estimate the number of clusters. This paper proposes the OPFsemble, an Optimum-Path Forest (OPF) ensemble pruning approach that uses the unsupervised OPF to select the most representative classifiers while maintaining diversity. It also proposes five variants of pruning to select the most representative classifiers and combine the final predictions. The proposed approach is compared against several aggregation methods for the ensemble process. Experiments conducted over twelve datasets show the OPFsemble provides the best scores and even statistical similarity with the baseline ensemble approaches.
更多查看译文
关键词
Ensemble Pruning,Optimum-Path Forest,Ensemble Model,Machine Learning
AI 理解论文
溯源树
样例
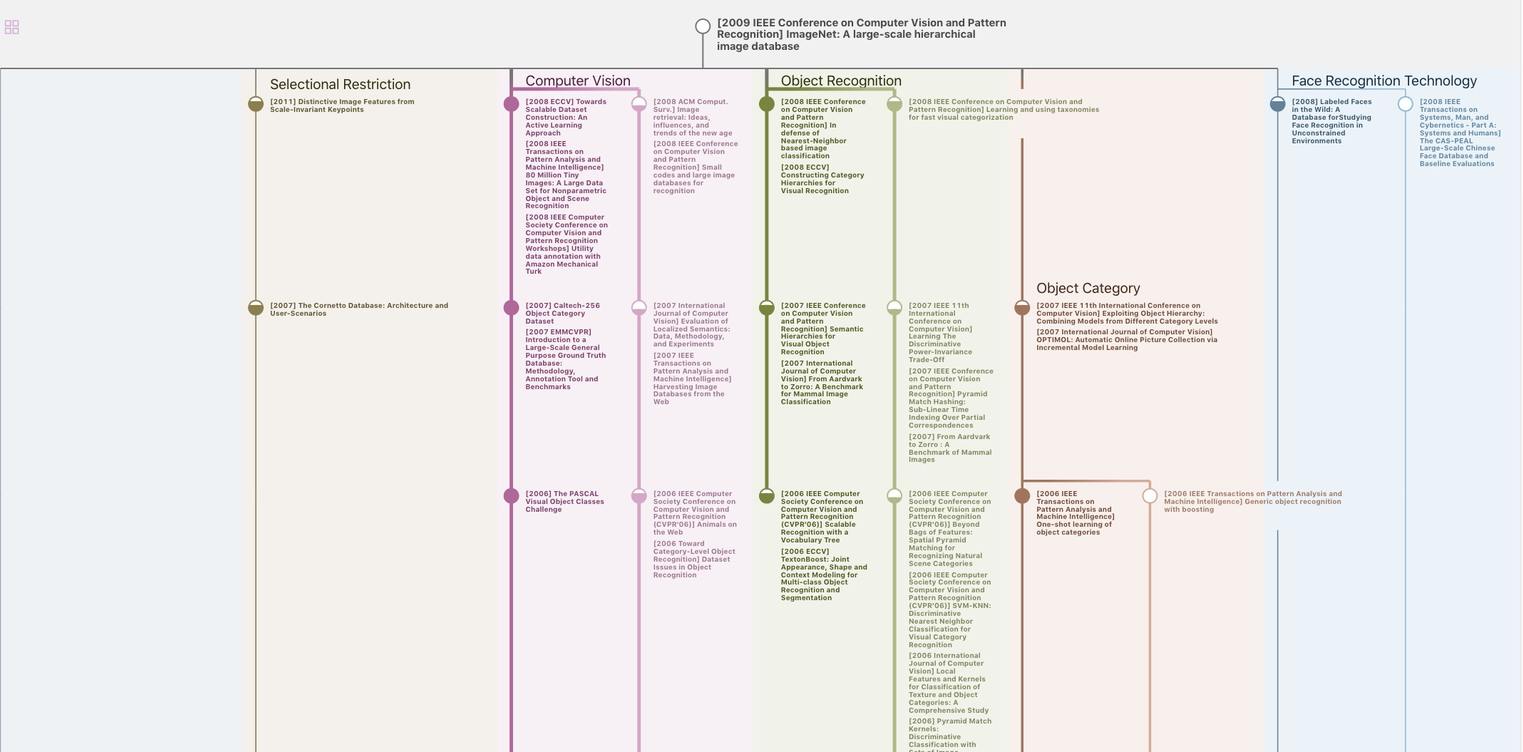
生成溯源树,研究论文发展脉络
Chat Paper
正在生成论文摘要