TECD: A Transformer Encoder Convolutional Decoder for High-Dimensional Biomedical Data.
ICCSA (Workshops 1)(2023)
摘要
In recent years, machine learning and deep learning methods have been increasingly explored in a variety of application domains, including healthcare. Despite the rapid advances in this field, several challenges still need to be addressed to properly model complex biomedical datasets, such as genomic datasets or physiological signals from wearable sensors, that exhibit a very high dimensionality, i.e., a high number of variables or features which can be mutually related. As evidenced by the literature, the induction of reliable predictive models becomes intrinsically harder as the data dimensionality increases. To give a contribution to this field, this paper explores a new deep learning approach that leverages the emerging paradigm of Transformers , which can capture long-range dependencies among the input features and combines them with a Convolutional Neural Network , which is suited for capturing local patterns and dependencies. The resulting architecture has shown very promising results on six biomedical datasets with high dimensionality (several thousands of features), paving the way for further research in this area.
更多查看译文
关键词
transformer encoder convolutional decoder,data,high-dimensional
AI 理解论文
溯源树
样例
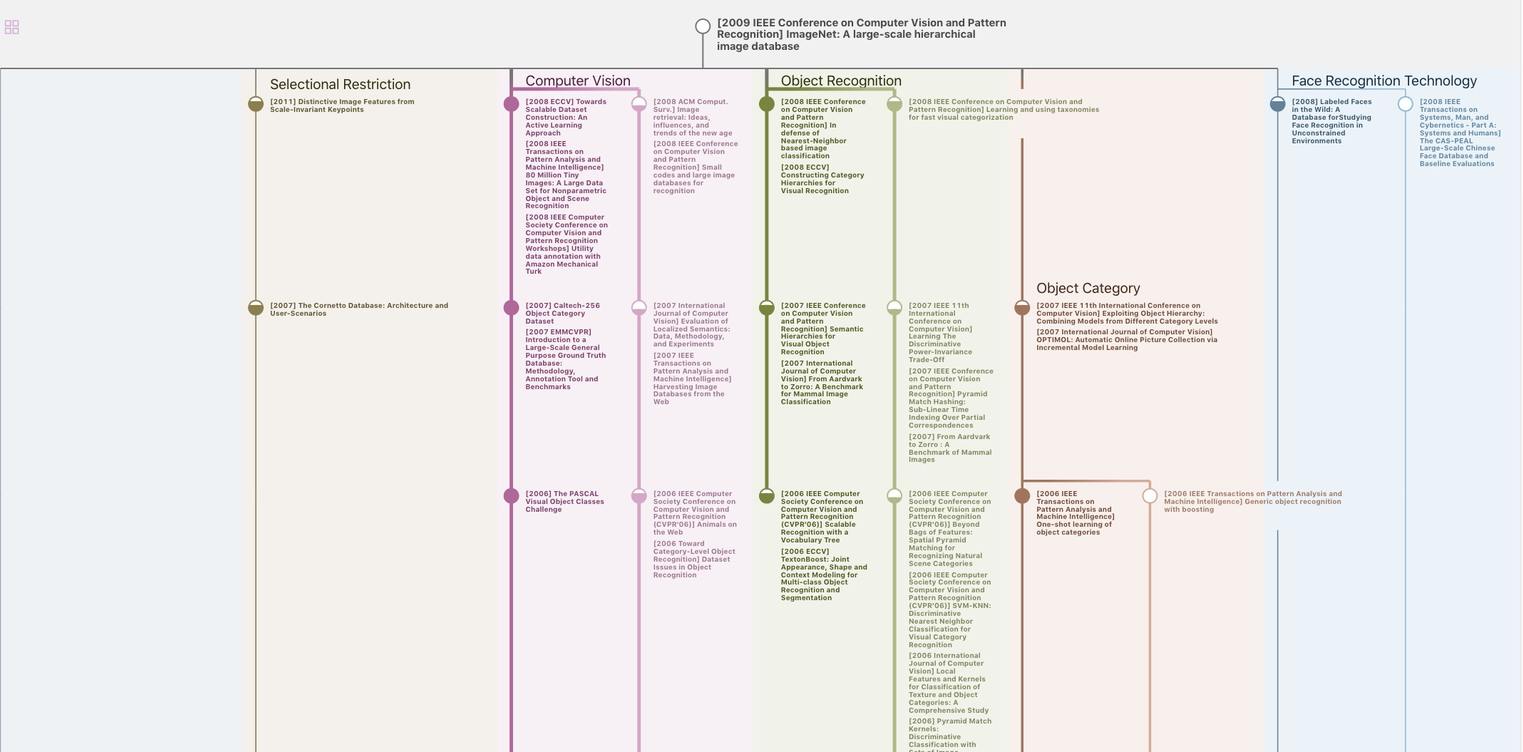
生成溯源树,研究论文发展脉络
Chat Paper
正在生成论文摘要