An Unseen Features-Enriched Lifelong Machine Learning Framework.
ICCSA (2)(2023)
摘要
The dialect of a machine learning model is comprised of the features encountered during training. Nonetheless, as time passes, a deployed machine learning model may encounter certain features for the first time. In conventional machine learning approaches, newly observed features are typically discarded during testing data sample preprocessing. In lifelong machine learning, newly observed features may have appeared in the feature space of previously learned tasks; consequently, the knowledge associated with those features present in the knowledge base is incorporated to handle these features. However, there may be some features that have yet to appear in the knowledge base; lifelong machine learning also discards such features. Features that were not seen before are called unseen features. In this paper, we propose an enhanced lifelong machine learning framework for handling unseen features during the testing phase that incorporates relative knowledge. To extract relative knowledge, we retrieve semantically similar features using a language model. In addition, semantically similar features are examined in the knowledge base, and the knowledge of those present in the knowledge base is incorporated in order to deal with unseen features. Experiments conducted on the Amazon review dataset indicate that the proposed method outperforms three baselines and is competitive with state-of-the-art methods.
更多查看译文
关键词
machine learning,features-enriched
AI 理解论文
溯源树
样例
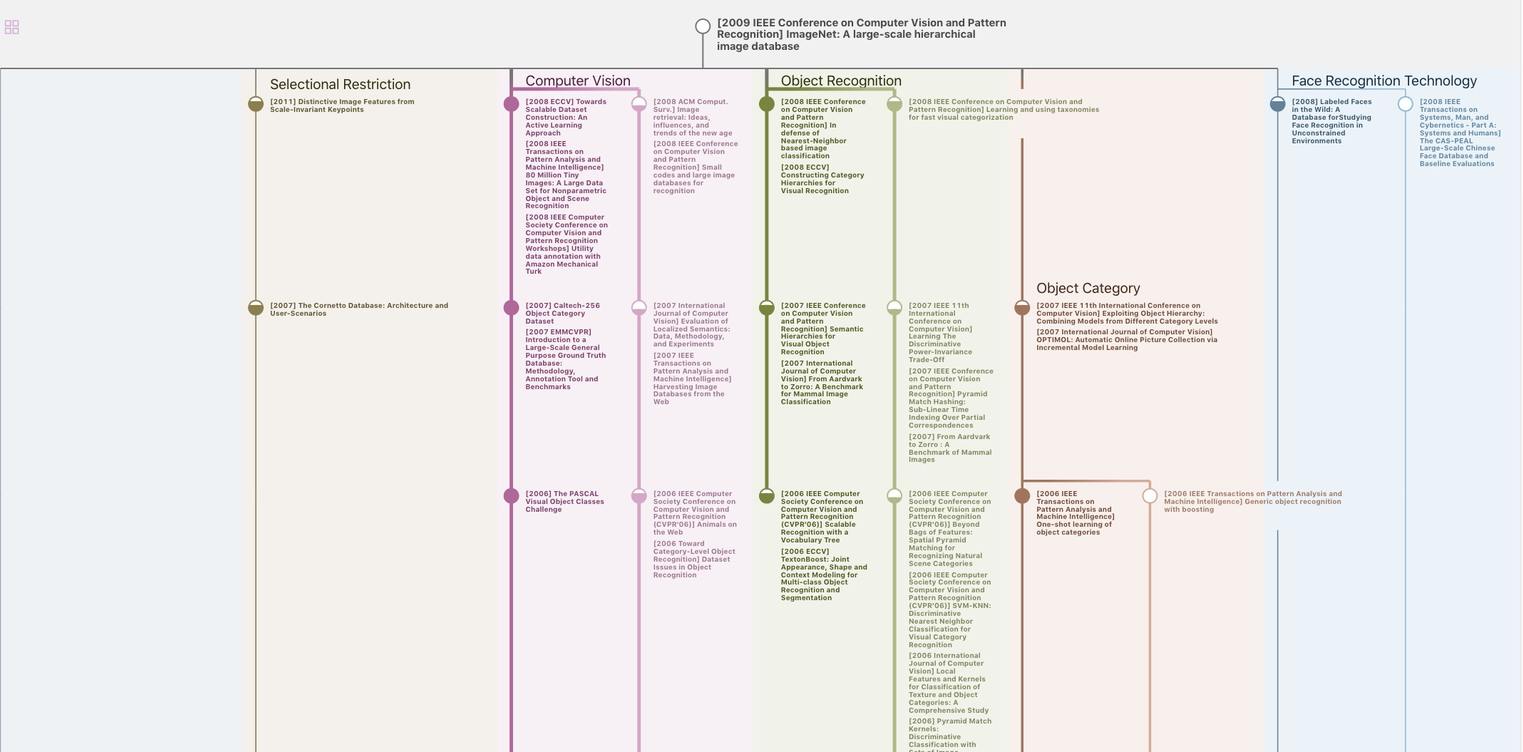
生成溯源树,研究论文发展脉络
Chat Paper
正在生成论文摘要