Mitigating Traffic Congestion in Smart and Sustainable Cities Using Machine Learning: A Review.
ICCSA (2)(2023)
摘要
Machine Learning (ML) algorithms can analyze large amounts of traffic data, learn from patterns and past behaviors, and provide insights into the current and future traffic flow. ML can also optimize traffic management, including traffic signal control, route optimization, and demand forecasting. Traffic prediction is a key application of ML in traffic management, with studies showing that ML outperforms traditional methods in predicting traffic congestion. ML is an effective tool for managing traffic, particularly for projecting traffic demand, predicting traffic congestion, and optimising routes. Studies have revealed that ML is more efficient than conventional techniques in these areas, leading to decreased journey times, improved traffic flow, and better traffic management in general. As the demand for efficient and sustainable transportation systems rises, ML integration in traffic management is expected to be vital in addressing these requirements. Nevertheless, there are obstacles and restrictions that must be overcome, such as shortcomings in the reliability of data and model interpretability. Despite these challenges, ML has the potential to mitigate traffic congestion and enhance urban mobility in smart and sustainable cities. Further research is needed to address these challenges and fully realize the potential of ML in traffic management.
更多查看译文
关键词
traffic congestion,sustainable cities,machine learning
AI 理解论文
溯源树
样例
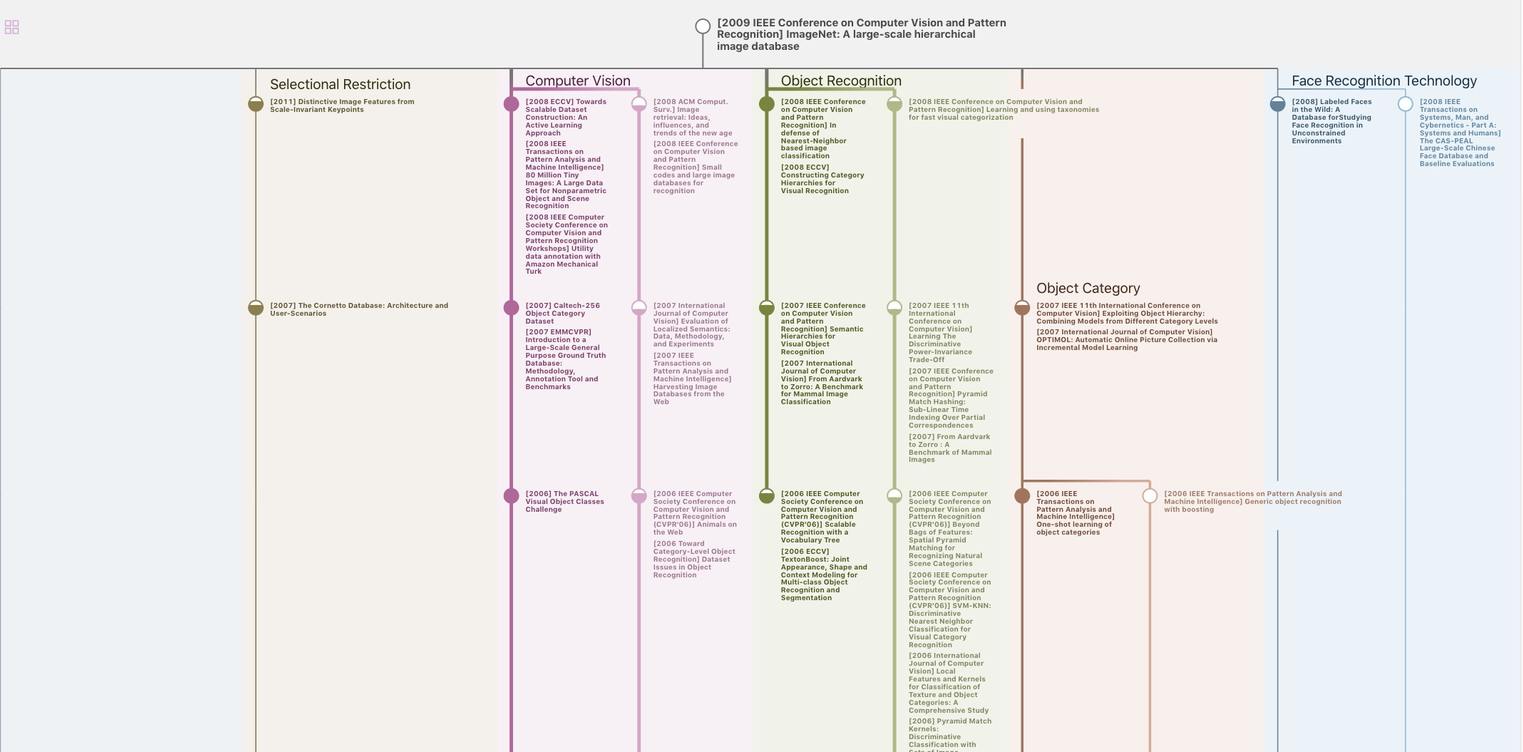
生成溯源树,研究论文发展脉络
Chat Paper
正在生成论文摘要