TMPNN: High-Order Polynomial Regression Based on Taylor Map Factorization
AAAI 2024(2024)
摘要
The paper presents Taylor Map Polynomial Neural Network (TMPNN), a novel form of very high-order polynomial regression, in which the same coefficients for a lower-to-moderate-order polynomial regression are iteratively reapplied so as to achieve a higher-order model without the number of coefficients to be fit exploding in the usual curse-of-dimensionality way. This method naturally implements multi-target regression and can capture internal relationships between targets. We also introduce an approach for model interpretation in the form of systems of differential equations. By benchmarking on Feynman regression, UCI, Friedman-1, and real-life industrial datasets, we demonstrate that the proposed method performs comparably to the state-of-the-art regression methods and outperforms them on specific tasks.
更多查看译文
关键词
ML: Classification and Regression,ML: Transparent, Interpretable, Explainable ML
AI 理解论文
溯源树
样例
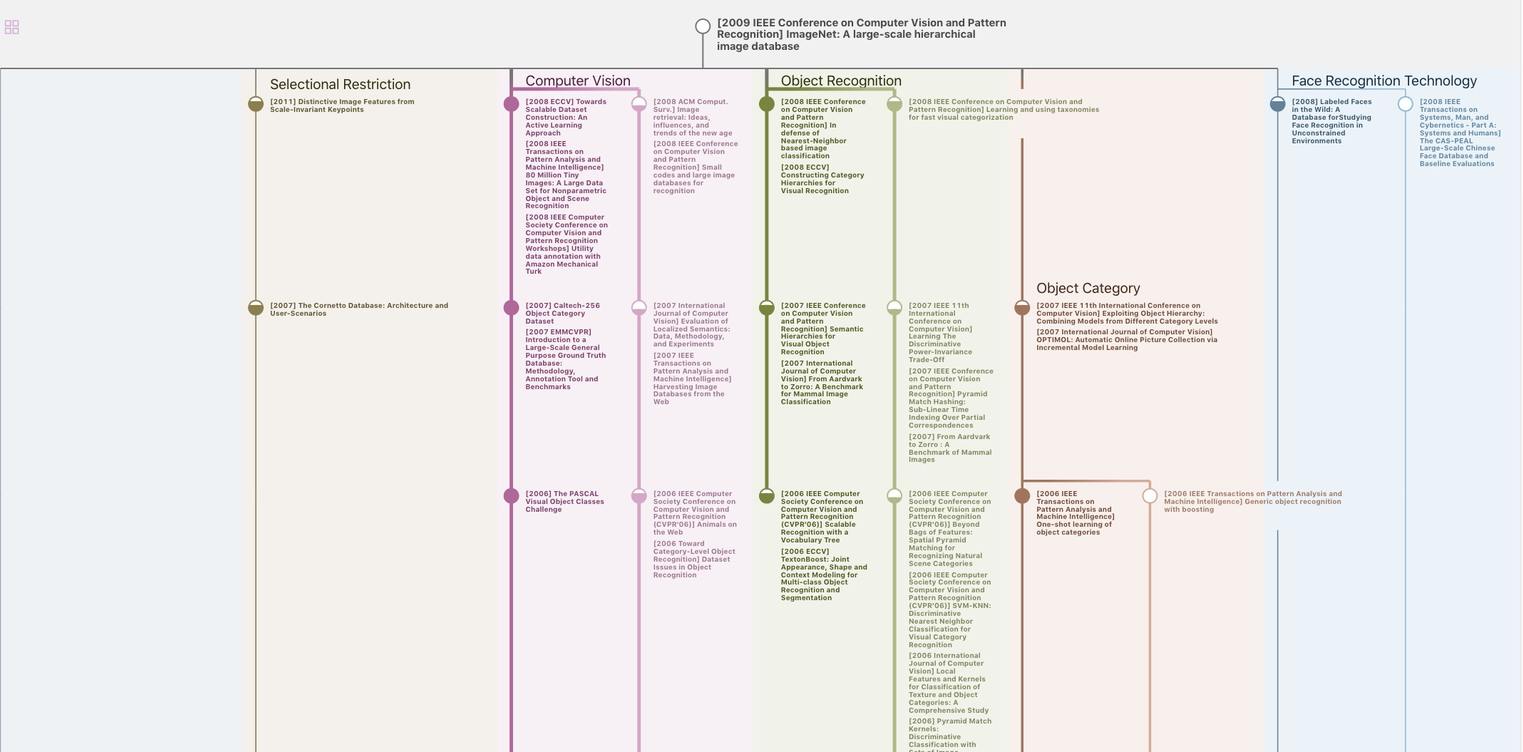
生成溯源树,研究论文发展脉络
Chat Paper
正在生成论文摘要