Asynchronous Silent Programmable Matter: Line Formation
Lecture Notes in Computer Science Stabilization, Safety, and Security of Distributed Systems(2023)
关键词
Programmable Matter
AI 理解论文
溯源树
样例
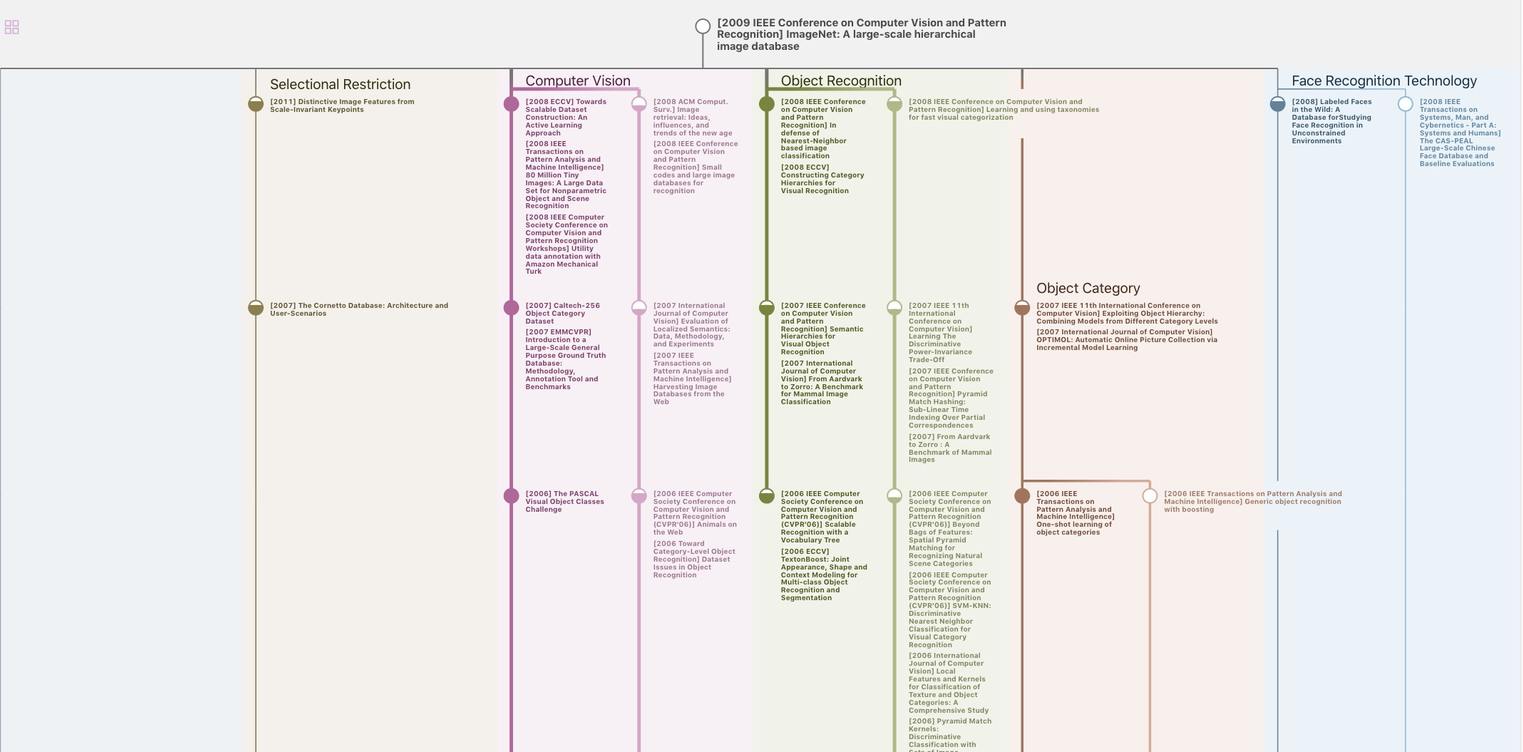
生成溯源树,研究论文发展脉络
Chat Paper
正在生成论文摘要
Lecture Notes in Computer Science Stabilization, Safety, and Security of Distributed Systems(2023)