Structural Transfer Learning in NL-to-Bash Semantic Parsers
CoRR(2023)
摘要
Large-scale pre-training has made progress in many fields of natural language processing, though little is understood about the design of pre-training datasets. We propose a methodology for obtaining a quantitative understanding of structural overlap between machine translation tasks. We apply our methodology to the natural language to Bash semantic parsing task (NLBash) and show that it is largely reducible to lexical alignment. We also find that there is strong structural overlap between NLBash and natural language to SQL. Additionally, we perform a study varying compute expended during pre-training on the English to German machine translation task and find that more compute expended during pre-training does not always correspond semantic representations with stronger transfer to NLBash.
更多查看译文
关键词
structural transfer learning,nl-to-bash
AI 理解论文
溯源树
样例
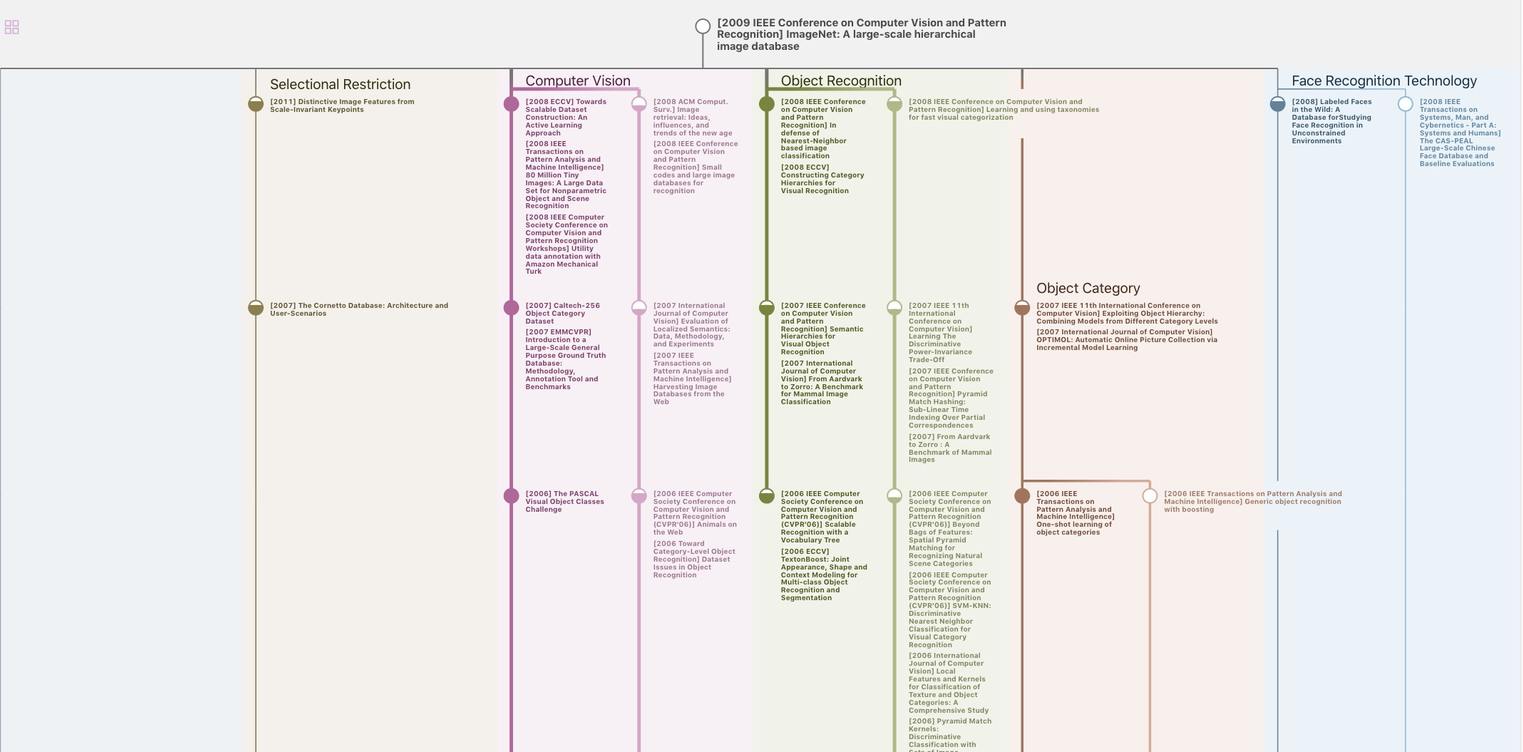
生成溯源树,研究论文发展脉络
Chat Paper
正在生成论文摘要