Explainable Graph Spectral Clustering of Text Documents.
CoRR(2023)
摘要
Spectral clustering methods are known for their ability to represent clusters of diverse shapes, densities etc. However, results of such algorithms, when applied e.g. to text documents, are hard to explain to the user, especially due to embedding in the spectral space which has no obvious relation to document contents. Therefore there is an urgent need to elaborate methods for explaining the outcome of the clustering. This paper presents a contribution towards this goal. We present a proposal of explanation of results of combinatorial Laplacian based graph spectral clustering. It is based on showing (approximate) equivalence of combinatorial Laplacian embedding, $K$-embedding (proposed in this paper) and term vector space embedding. Hence a bridge is constructed between the textual contents and the clustering results. We provide theoretical background for this approach. We performed experimental study showing that $K$-embedding approximates well Laplacian embedding under favourable block matrix conditions and show that approximation is good enough under other conditions.
更多查看译文
关键词
explainable graph spectral clustering,text
AI 理解论文
溯源树
样例
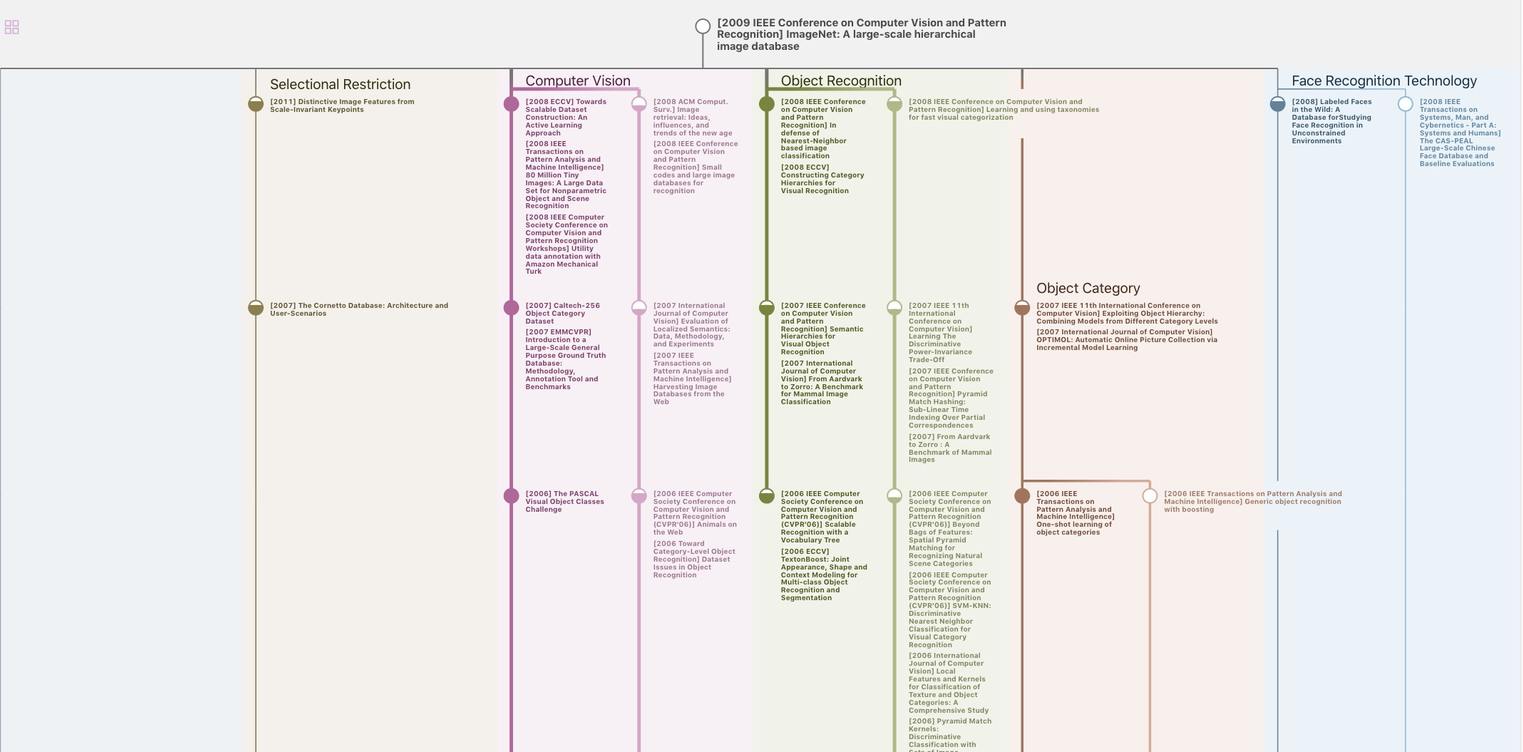
生成溯源树,研究论文发展脉络
Chat Paper
正在生成论文摘要