Automatic Feature Engineering for Time Series Classification: Evaluation and Discussion
IJCNN(2023)
摘要
Time Series Classification (TSC) has received much attention in the past two decades and is still a crucial and challenging problem in data science and knowledge engineering. Indeed, along with the increasing availability of time series data, many TSC algorithms have been suggested by the research community in the literature. Besides state-of-the-art methods based on similarity measures, intervals, shapelets, dictionaries, deep learning methods or hybrid ensemble methods, several tools for extracting unsupervised informative summary statistics, aka features, from time series have been designed in the recent years. Originally designed for descriptive analysis and visualization of time series with informative and interpretable features, very few of these feature engineering tools have been benchmarked for TSC problems and compared with state-of-the-art TSC algorithms in terms of predictive performance. In this article, we aim at filling this gap and propose a simple TSC process to evaluate the potential predictive performance of the feature sets obtained with existing feature engineering tools. Thus, we present an empirical study of 11 feature engineering tools branched with 9 supervised classifiers over 112 time series data sets. The analysis of the results of more than 10000 learning experiments indicate that feature-based methods perform as accurately as current state-of-the-art TSC algorithms, and thus should rightfully be considered further in the TSC literature.
更多查看译文
关键词
aka features,automatic feature engineering,data science,data visualization,deep learning methods,descriptive analysis,hybrid ensemble methods,knowledge engineering,supervised classifiers,time series classification,time series data sets,TSC algorithms,unsupervised informative summary statistics
AI 理解论文
溯源树
样例
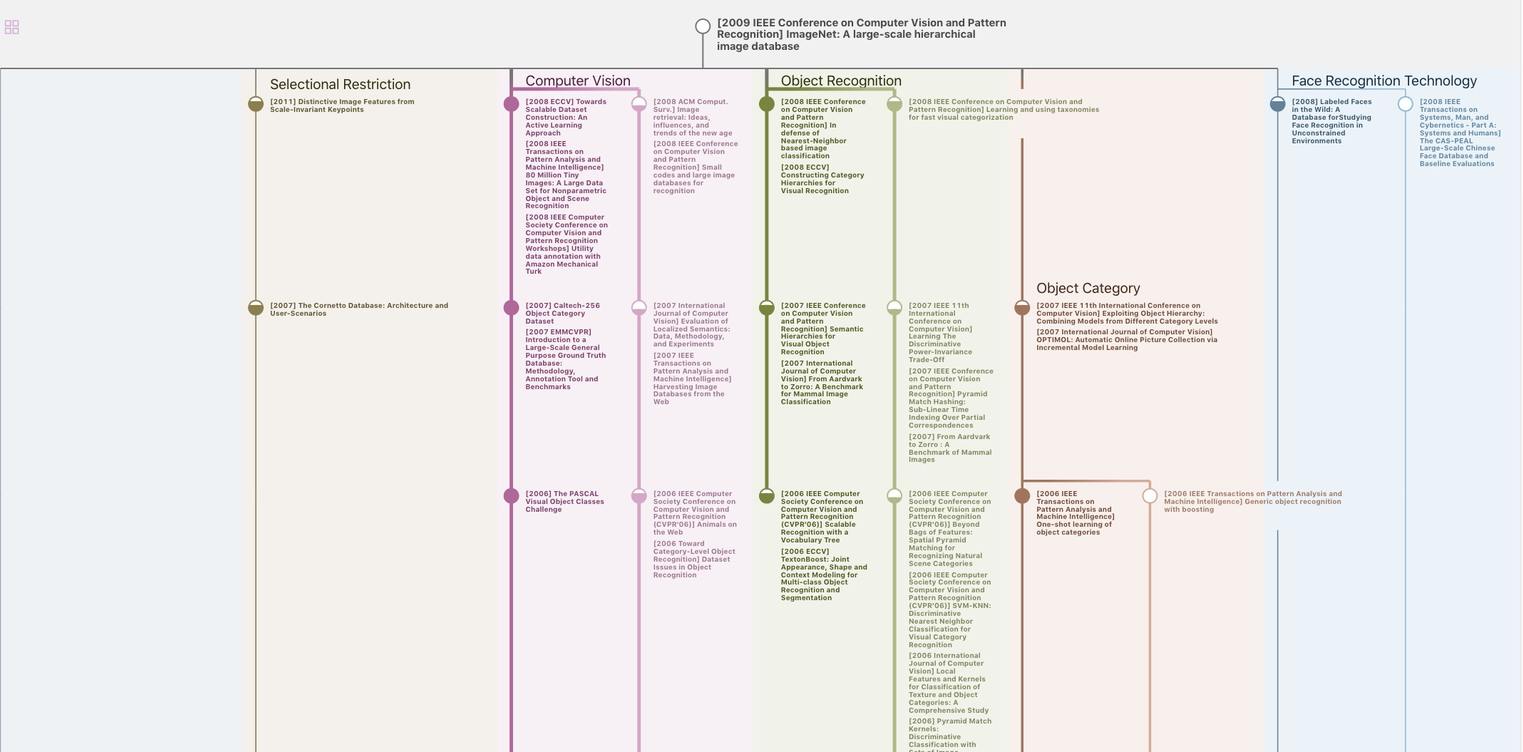
生成溯源树,研究论文发展脉络
Chat Paper
正在生成论文摘要