Predicting drug-disease associations through similarity network fusion and multi-view feature projection representation.
IEEE journal of biomedical and health informatics(2023)
摘要
Predicting drug-disease associations (DDAs) through computational methods has become a prevalent trend in drug development because of their high efficiency and low cost. Existing methods usually focus on constructing heterogeneous networks by collecting multiple data resources to improve prediction ability. However, potential association possibilities of numerous unconfirmed drug-related or disease-related pairs are not sufficiently considered. In this paper, we propose a novel computational model to predict new DDAs. First, a heterogeneous network is constructed, including four types of nodes (drugs, targets, cell lines, diseases) and three types of edges (associations, association scores, similarities). Second, an updating and merging-based similarity network fusion method, termed UM-SF, is presented to fuse various similarity networks with diverse weights. Finally, an intermediate layer-mediated multi-view feature projection representation method, termed IM-FP, is proposed to calculate the predicted DDA scores. This method uses multiple association scores to construct multi-view drug features, then projects them into disease space through the intermediate layer, where an intermediate layer similarity constraint is designed to learn the projection matrices. Results of comparative experiments reveal the effectiveness of our innovations. Comparisons with other state-of-the-art models by the 10-fold cross-validation experiment indicate our model's advantage on AUROC and AUPR metrics. Moreover, our proposed model successfully predicted 107 novel high-ranked DDAs.
更多查看译文
关键词
similarity network fusion,associations,drug-disease,multi-view
AI 理解论文
溯源树
样例
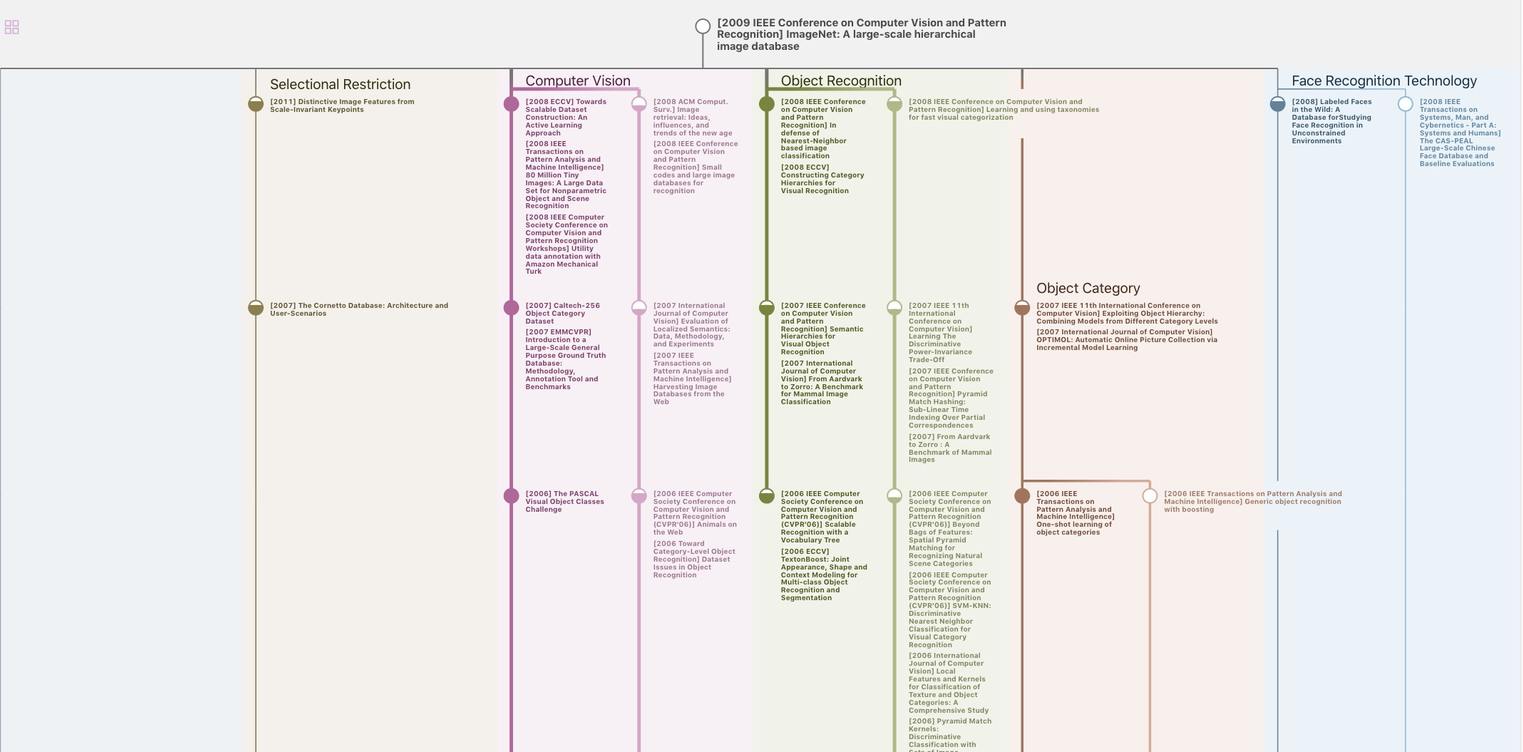
生成溯源树,研究论文发展脉络
Chat Paper
正在生成论文摘要