Hierarchical Federated Learning in Wireless Networks: Pruning Tackles Bandwidth Scarcity and System Heterogeneity
IEEE Transactions on Wireless Communications(2023)
摘要
While a practical wireless network has many tiers where end users do not
directly communicate with the central server, the users' devices have limited
computation and battery powers, and the serving base station (BS) has a fixed
bandwidth. Owing to these practical constraints and system models, this paper
leverages model pruning and proposes a pruning-enabled hierarchical federated
learning (PHFL) in heterogeneous networks (HetNets). We first derive an upper
bound of the convergence rate that clearly demonstrates the impact of the model
pruning and wireless communications between the clients and the associated BS.
Then we jointly optimize the model pruning ratio, central processing unit (CPU)
frequency and transmission power of the clients in order to minimize the
controllable terms of the convergence bound under strict delay and energy
constraints. However, since the original problem is not convex, we perform
successive convex approximation (SCA) and jointly optimize the parameters for
the relaxed convex problem. Through extensive simulation, we validate the
effectiveness of our proposed PHFL algorithm in terms of test accuracy, wall
clock time, energy consumption and bandwidth requirement.
更多查看译文
关键词
Heterogeneous network,hierarchical federated learning,model pruning,resource management
AI 理解论文
溯源树
样例
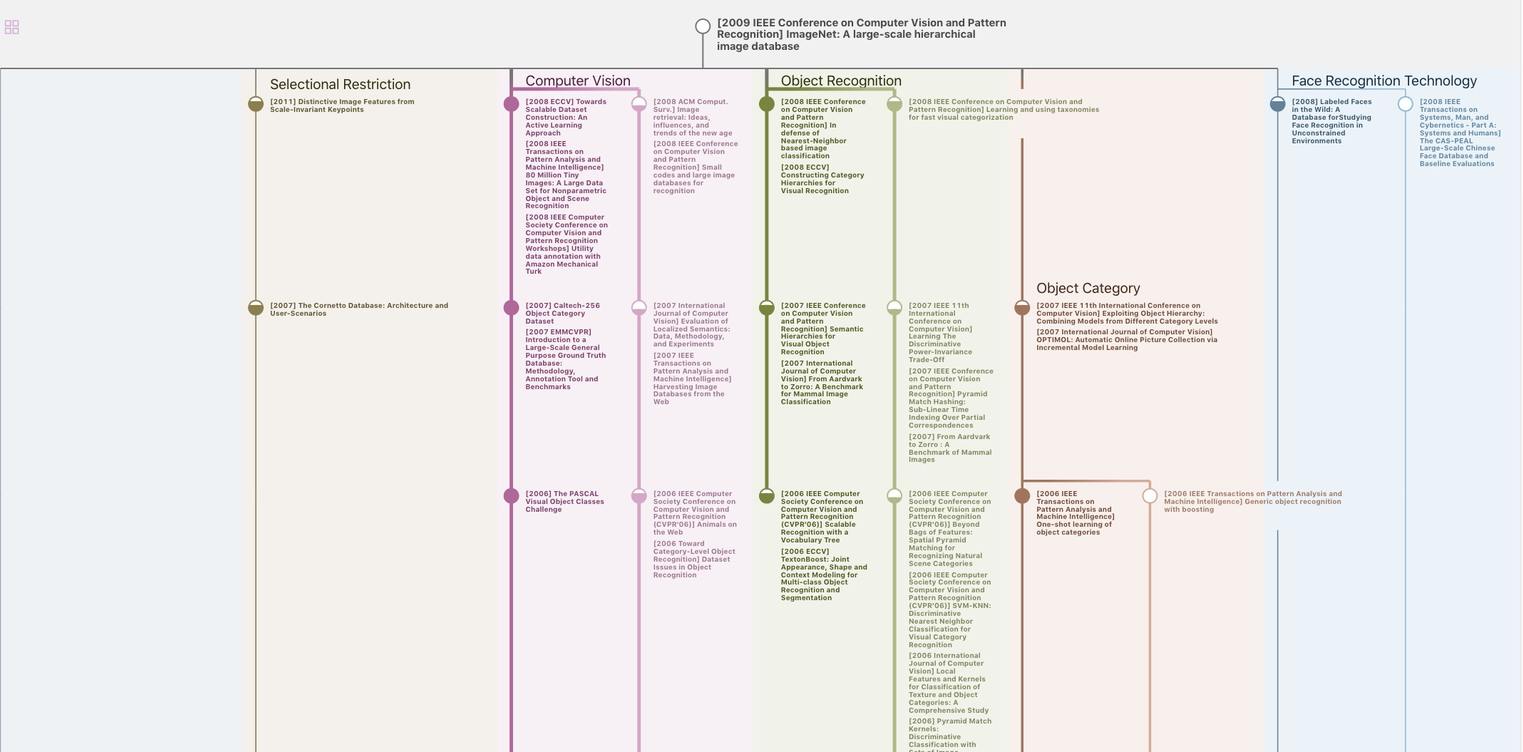
生成溯源树,研究论文发展脉络
Chat Paper
正在生成论文摘要