Composed Image Retrieval via Cross Relation Network With Hierarchical Aggregation Transformer
IEEE transactions on image processing : a publication of the IEEE Signal Processing Society(2023)
摘要
Composing Text and Image to Image Retrieval (CTI-IR) aims at finding the target image, which matches the query image visually along with the query text semantically. However, existing works ignore the fact that the reference text usually serves multiple functions, e.g., modification and auxiliary. To address this issue, we put forth a unified solution, namely Hierarchical Aggregation Transformer incorporated with Cross Relation Network (CRN). CRN unifies modification and relevance manner in a single framework. This configuration shows broader applicability, enabling us to model both modification and auxiliary text or their combination in triplet relationships simultaneously. Specifically, CRN includes: 1) Cross Relation Network comprehensively captures the relationships of various composed retrieval scenarios caused by two different query text types, allowing a unified retrieval model to designate adaptive combination strategies for flexible applicability; 2) Hierarchical Aggregation Transformer aggregates top-down features with Multi-layer Perceptron (MLP) to overcome the limitations of edge information loss in a window-based multi-stage Transformer. Extensive experiments demonstrate the superiority of the proposed CRN over all three fashion-domain datasets. Code is available at github.com/yan9qu/crn.
更多查看译文
关键词
Cross Relation,Image Retrieval,Transformer
AI 理解论文
溯源树
样例
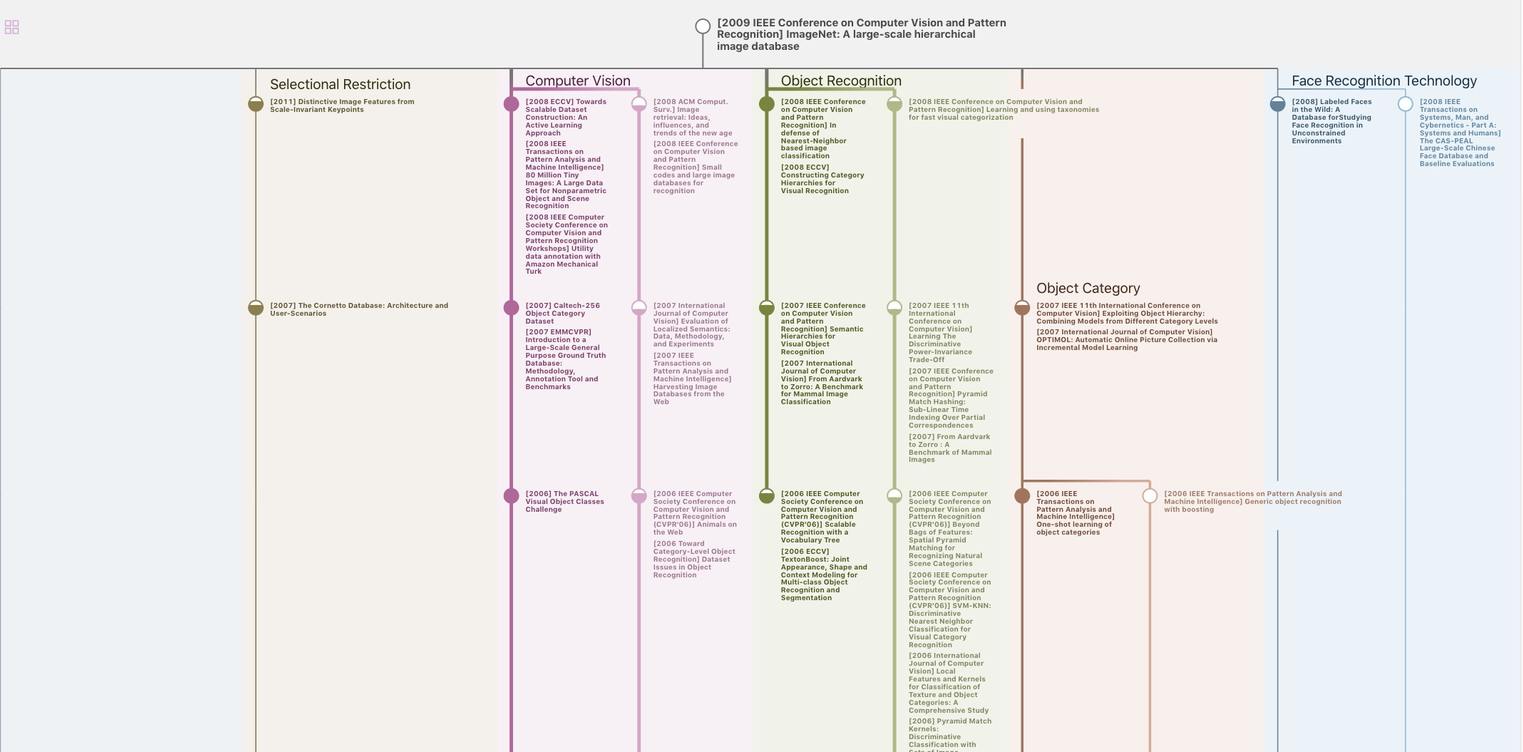
生成溯源树,研究论文发展脉络
Chat Paper
正在生成论文摘要