Lightweight image super-resolution based multi-order gated aggregation network.
Neural networks : the official journal of the International Neural Network Society(2023)
摘要
Recently, Transformer-based models are taken much focus on solving the task of image super-resolution (SR) due to their ability to achieve better performance. However, these models combined huge computational cost during the computing self-attention mechanism. To solve this problem, we proposed a multi-order gated aggregation super-resolution network (MogaSRN) for low-level vision based on the concept of the MogaNet that is developed for high-level vision. The concept of the MogaSRN model is based on spatial multi-order context aggregation and adaptive channel-wise reallocation with the aid of the multi-layer perceptron (MLP). In contrast to the MogaNet model, in which the resolution of each stage decreased by a factor of 2, the resolution of the MogaSRN is stayed fixed during the deep features extraction. Moreover, the structure of the MogaSRN model is built based on balancing the performance and the model complexity. We evaluated our model based on five benchmark datasets concluding that the MogaSRN model can achieve significant improvements compared to the state-of-the-art. Moreover, our model shows the good visual quality and accuracy of the reconstruction. Finally, our model has 3.7 × faster runtime at the scale of × 4 compared to LWSwinIR with better performance.
更多查看译文
关键词
Convolution neural network,Spatial aggregation,Adaptive channel aggregation,Multi-order gated aggregation,Lightweight image super-resolution
AI 理解论文
溯源树
样例
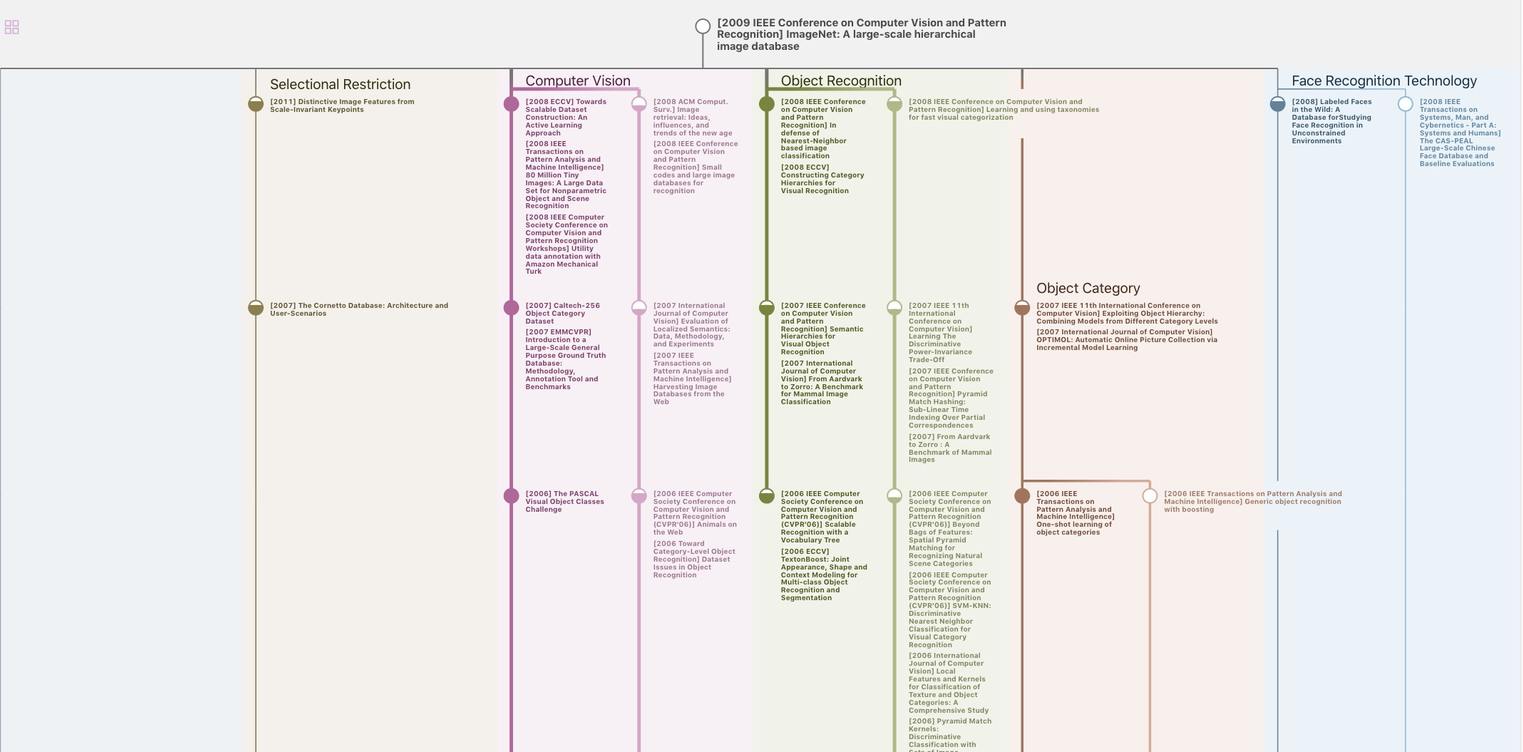
生成溯源树,研究论文发展脉络
Chat Paper
正在生成论文摘要