Fetal weight estimation based on deep neural network: a retrospective observational study
BMC pregnancy and childbirth(2023)
摘要
Background Improving the accuracy of estimated fetal weight (EFW) calculation can contribute to decision-making for obstetricians and decrease perinatal complications. This study aimed to develop a deep neural network (DNN) model for EFW based on obstetric electronic health records. Methods This study retrospectively analyzed the electronic health records of pregnant women with live births delivery at the obstetrics department of International Peace Maternity & Child Health Hospital between January 2016 and December 2018. The DNN model was evaluated using Hadlock’s formula and multiple linear regression. Results A total of 34824 live births (23922 primiparas) from 49896 pregnant women were analyzed. The root-mean-square error of DNN model was 189.64 g (95% CI 187.95 g—191.16 g), and the mean absolute percentage error was 5.79% (95%CI: 5.70%—5.81%), significantly lower compared to Hadlock’s formula (240.36 g and 6.46%, respectively). By combining with previously unreported factors, such as birth weight of prior pregnancies, a concise and effective DNN model was built based on only 10 parameters. Accuracy rate of a new model increased from 76.08% to 83.87%, with root-mean-square error of only 243.80 g. Conclusions Proposed DNN model for EFW calculation is more accurate than previous approaches in this area and be adopted for better decision making related to fetal monitoring.
更多查看译文
关键词
Obstetrics and gynecology, Fetal monitoring, Fetal weight, Computer neural networks, Decision making
AI 理解论文
溯源树
样例
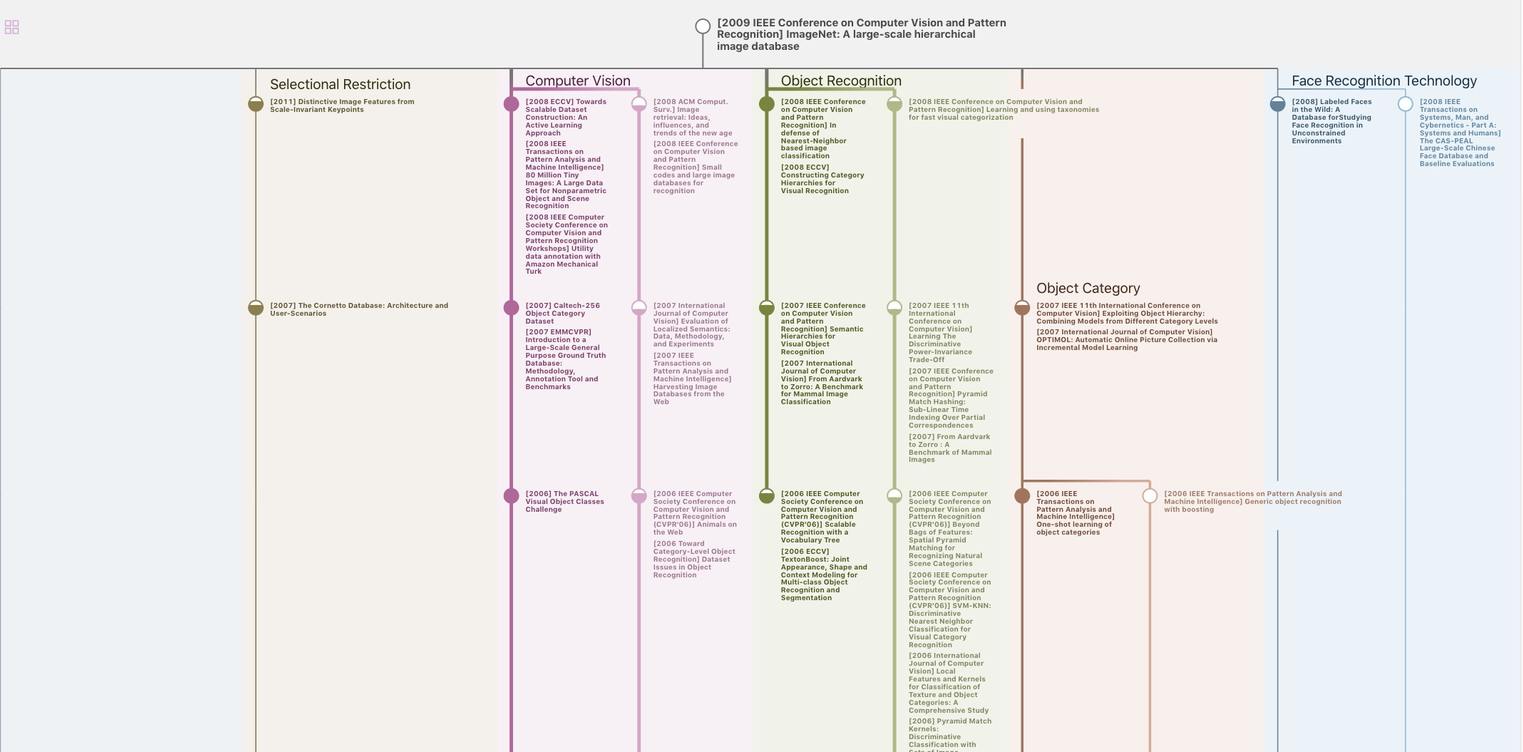
生成溯源树,研究论文发展脉络
Chat Paper
正在生成论文摘要