A Semidefinite Relaxation Based Branch-and-Bound Method for Tight Neural Network Verification.
AAAI(2023)
摘要
We introduce a novel method based on semidefinite program (SDP) for the tight and efficient verification of neural networks. The proposed SDP relaxation advances the present SoA in SDP-based neural network verification by adding a set of linear constraints based on eigenvectors. We extend this novel SDP relaxation by combining it with a branch-and-bound method that can provably close the relaxation gap up to zero. We show formally that the proposed approach leads to a provably tighter solution than the present state of the art. We report experimental results showing that the proposed method outperforms baselines in terms of verified accuracy while retaining an acceptable computational overhead.
更多查看译文
关键词
neural network,branch-and-bound
AI 理解论文
溯源树
样例
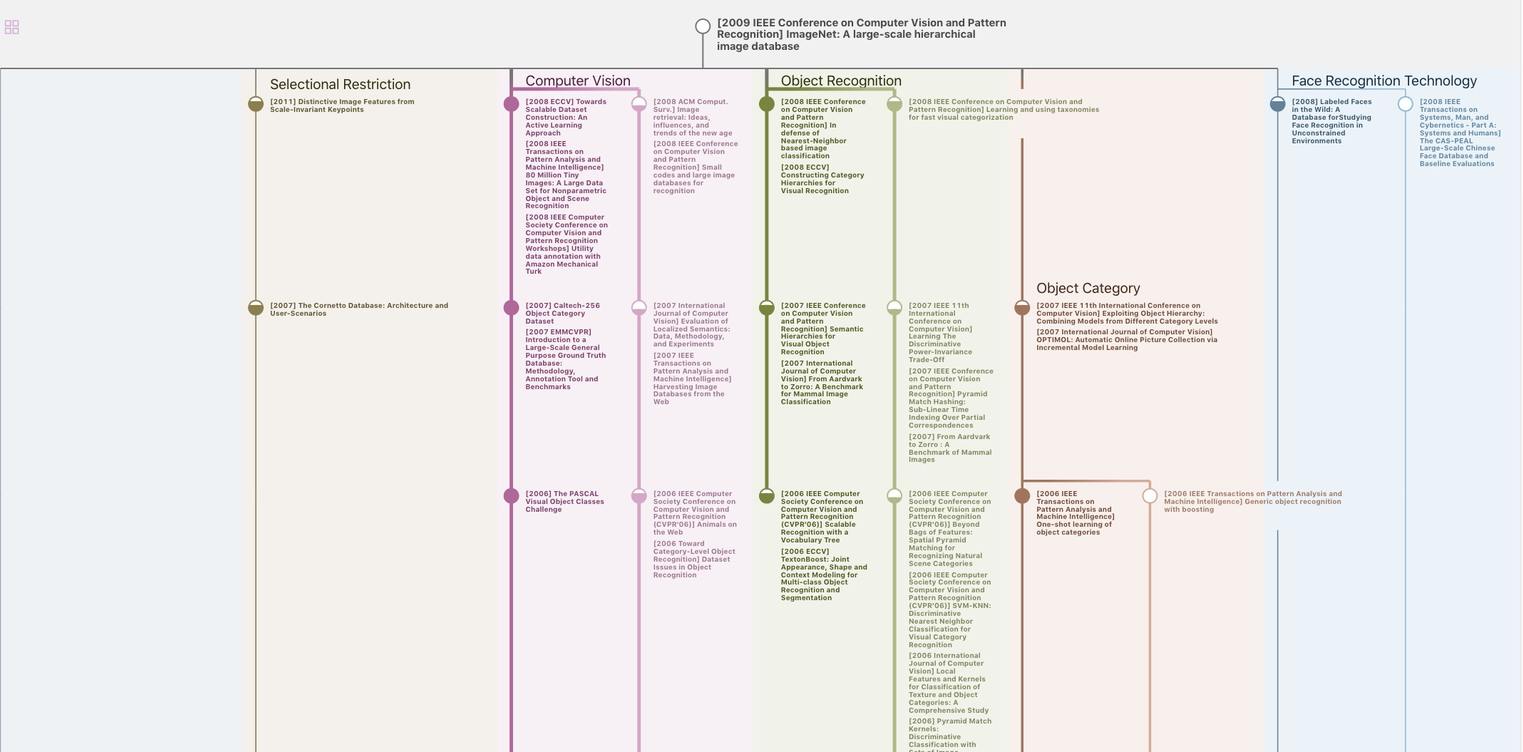
生成溯源树,研究论文发展脉络
Chat Paper
正在生成论文摘要