Anti-drifting Feature Selection via Deep Reinforcement Learning (Student Abstract).
AAAI(2023)
摘要
Feature selection (FS) is a crucial procedure in machine learning pipelines for its significant benefits in removing data redundancy and mitigating model overfitting. Since concept drift is a widespread phenomenon in streaming data and could severely affect model performance, effective FS on concept drifting data streams is imminent. However, existing state-of-the-art FS algorithms fail to adjust their selection strategy adaptively when the effective feature subset changes, making them unsuitable for drifting streams. In this paper, we propose a dynamic FS method that selects effective features on concept drifting data streams via deep reinforcement learning. Specifically, we present two novel designs: (i) a skip-mode reinforcement learning environment that shrinks action space size for high-dimensional FS tasks; (ii) a curiosity mechanism that generates intrinsic rewards to address the long-horizon exploration problem. The experiment results show that our proposed method outperforms other FS methods and can dynamically adapt to concept drifts.
更多查看译文
关键词
deep reinforcement learning,reinforcement learning,selection,anti-drifting
AI 理解论文
溯源树
样例
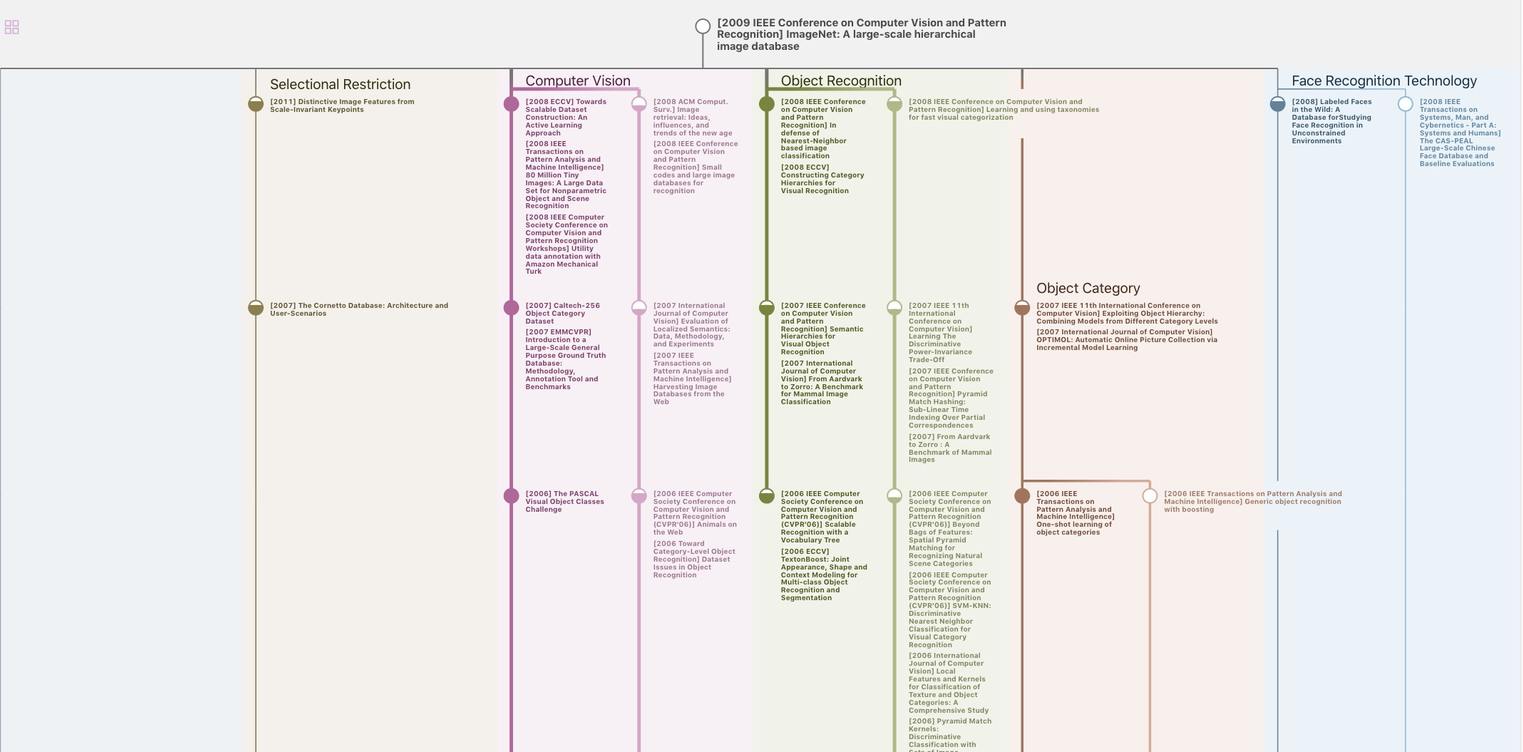
生成溯源树,研究论文发展脉络
Chat Paper
正在生成论文摘要