DeepGemini: Verifying Dependency Fairness for Deep Neural Network.
AAAI(2023)
摘要
Deep neural networks (DNNs) have been widely adopted in many decision-making industrial applications. Their fairness issues, i.e., whether there exist unintended biases in the DNN, receive much attention and become critical concerns, which can directly cause negative impacts in our daily life and potentially undermine the fairness of our society, especially with their increasing deployment at an unprecedented speed. Recently, some early attempts have been made to provide fairness assurance of DNNs, such as fairness testing, which aims at finding discriminatory samples empirically, and fairness certification, which develops sound but not complete analysis to certify the fairness of DNNs. Nevertheless, how to formally compute discriminatory samples and fairness scores (i.e., the percentage of fair input space), is still largely uninvestigated. In this paper, we propose DeepGemini , a novel fairness formal analysis technique for DNNs, which contains two key components: discriminatory sample discovery and fairness score computation. To uncover discriminatory samples, we encode the fairness of DNNs as safety properties and search for discriminatory samples by means of state-of-the-art verification techniques for DNNs. This reduction enables us to be the first to formally compute discriminatory samples. To compute the fairness score, we develop counterexample guided fairness analysis, which utilizes four heuristics to efficiently approximate a lower bound of fairness score. Extensive experimental evaluations demonstrate the effectiveness and efficiency of DeepGemini on commonly-used benchmarks, and DeepGemini outperforms state-of-the-art DNN fairness certification approaches in terms of both efficiency and scalability.
更多查看译文
AI 理解论文
溯源树
样例
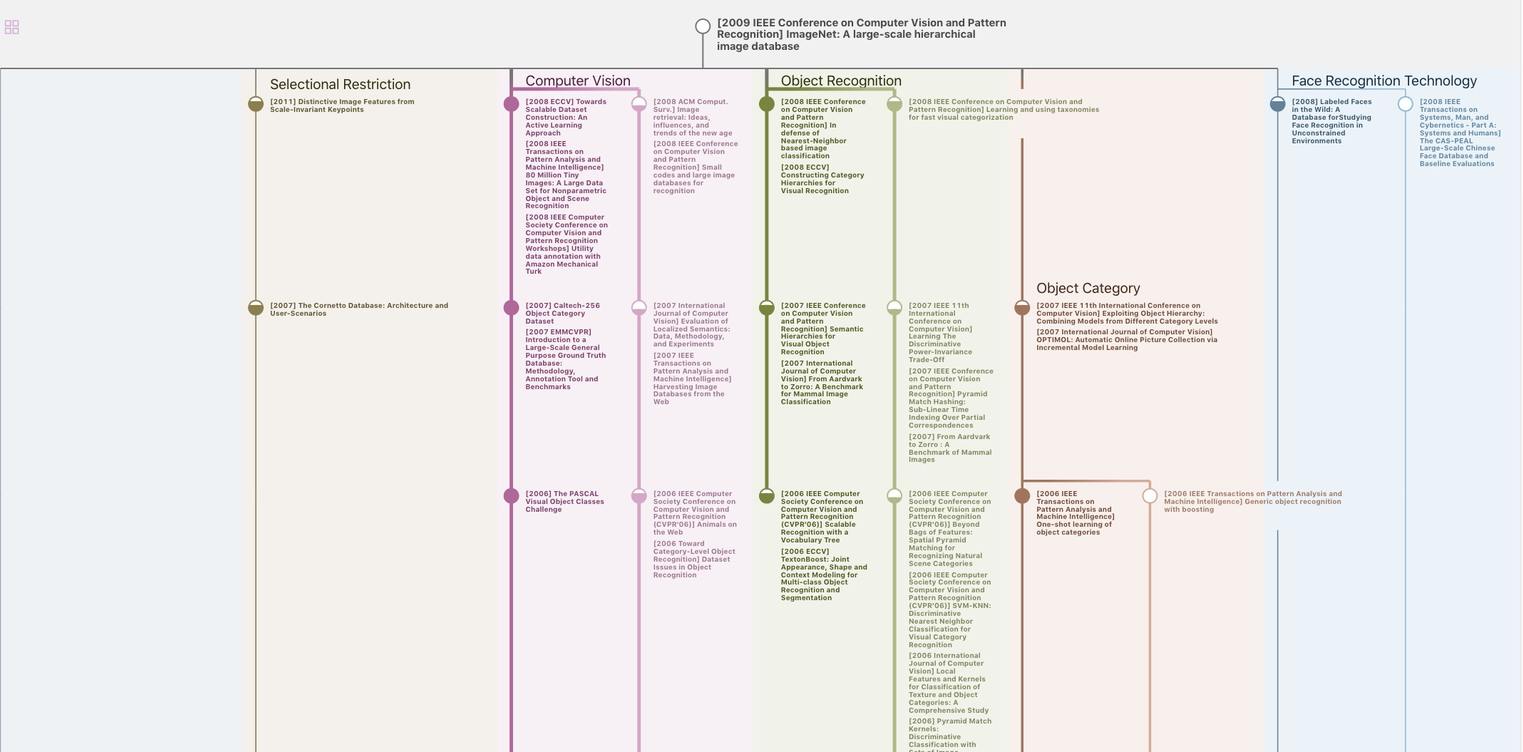
生成溯源树,研究论文发展脉络
Chat Paper
正在生成论文摘要