Differentially Private Nonlinear Causal Discovery from Numerical Data.
AAAI(2023)
摘要
Recently, several methods such as private ANM, EM-PC and Priv-PC have been proposed to perform differentially private causal discovery in various scenarios including bivariate, multivariate Gaussian and categorical cases. However, there is little effort on how to conduct private nonlinear causal discovery from numerical data. This work tries to challenge this problem. To this end, we propose a method to infer nonlinear causal relations from observed numerical data by using regression-based conditional independence test (RCIT) that consists of kernel ridge regression (KRR) and Hilbert-Schmidt independence criterion (HSIC) with permutation approximation. Sensitivity analysis for RCIT is given and a private constraint-based causal discovery framework with differential privacy guarantee is developed. Extensive simulations and real-world experiments for both conditional independence test and causal discovery are conducted, which show that our method is effective in handling nonlinear numerical cases and easy to implement. The source code of our method and data are available.
更多查看译文
关键词
private nonlinear causal discovery,data
AI 理解论文
溯源树
样例
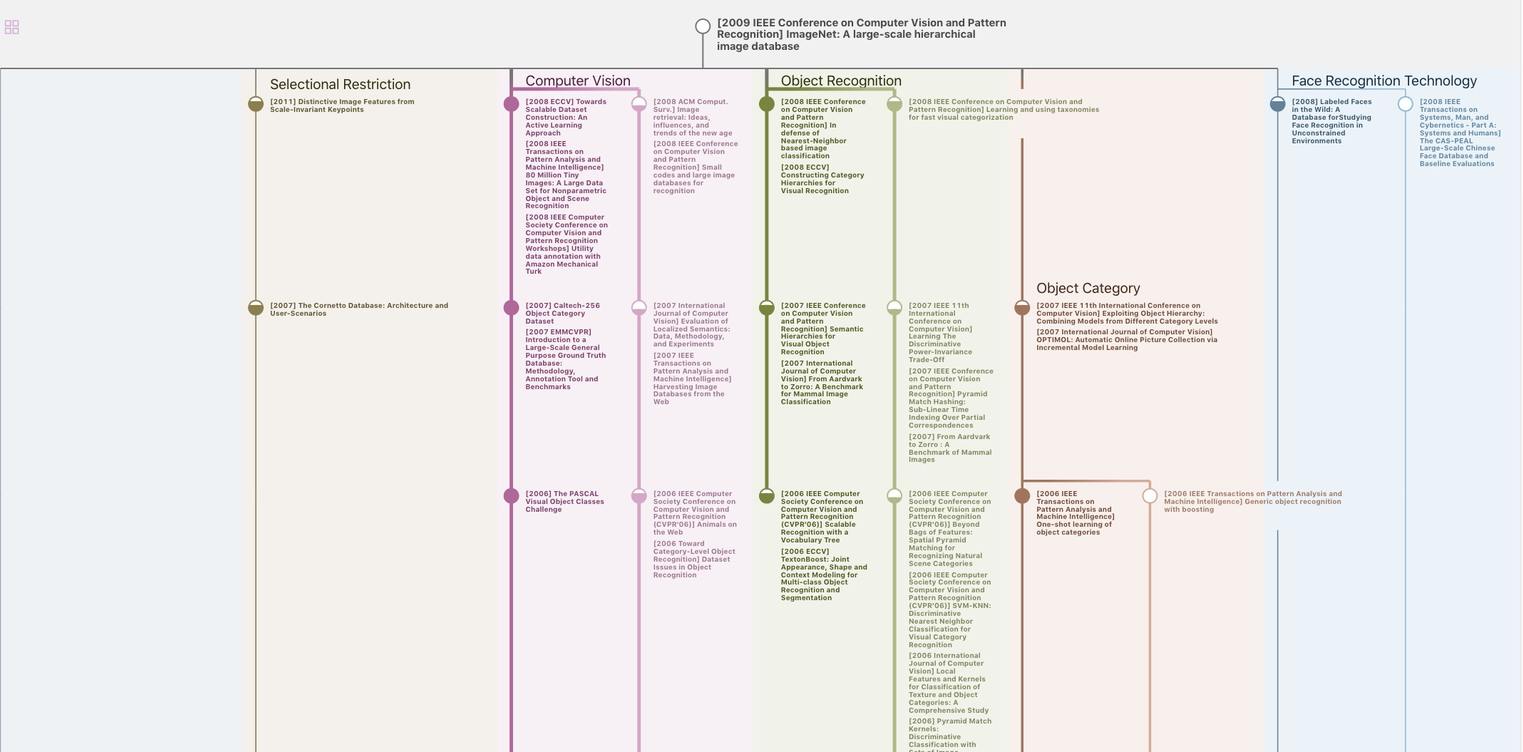
生成溯源树,研究论文发展脉络
Chat Paper
正在生成论文摘要