RL-based path planning for controller performance validation.
MED(2023)
摘要
Autonomous vehicles (AVs) will be part of everyday life in the near future. In order to accelerate this process, many subsystems need to be optimised and validated. One of the most important subsystem of AVs is the steering controller. It's task is to keep the vehicle on track, which is the reason, why many steering controllers have been designed for a large variety of applications. However, the validation of such controllers is a labour-intensive task, which is why in this paper, an Artificial Intelligence (AI) is trained to find an edge case path that brings the steering controller to its limits. This path is a sufficient substitute for a large set of paths and enables fast validation of steering controllers. This contribution describes the development of a reinforcement learning (RL) based path planner using the PPO-Algorithm to train a so called agent. Comparing the resulting key feature maps shows that the agent adapts to each controllers characteristics during the learning process. The result is demonstrated for three different state of the art path tracking controllers. For each controller the agent finds a path that leads to the controllers failure within seconds.
更多查看译文
关键词
artificial intelligence,autonomous vehicles,controller performance validation,edge case path,path tracking controllers,PPO-algorithm,reinforcement learning based path planner,RL-based path planning,steering controller design
AI 理解论文
溯源树
样例
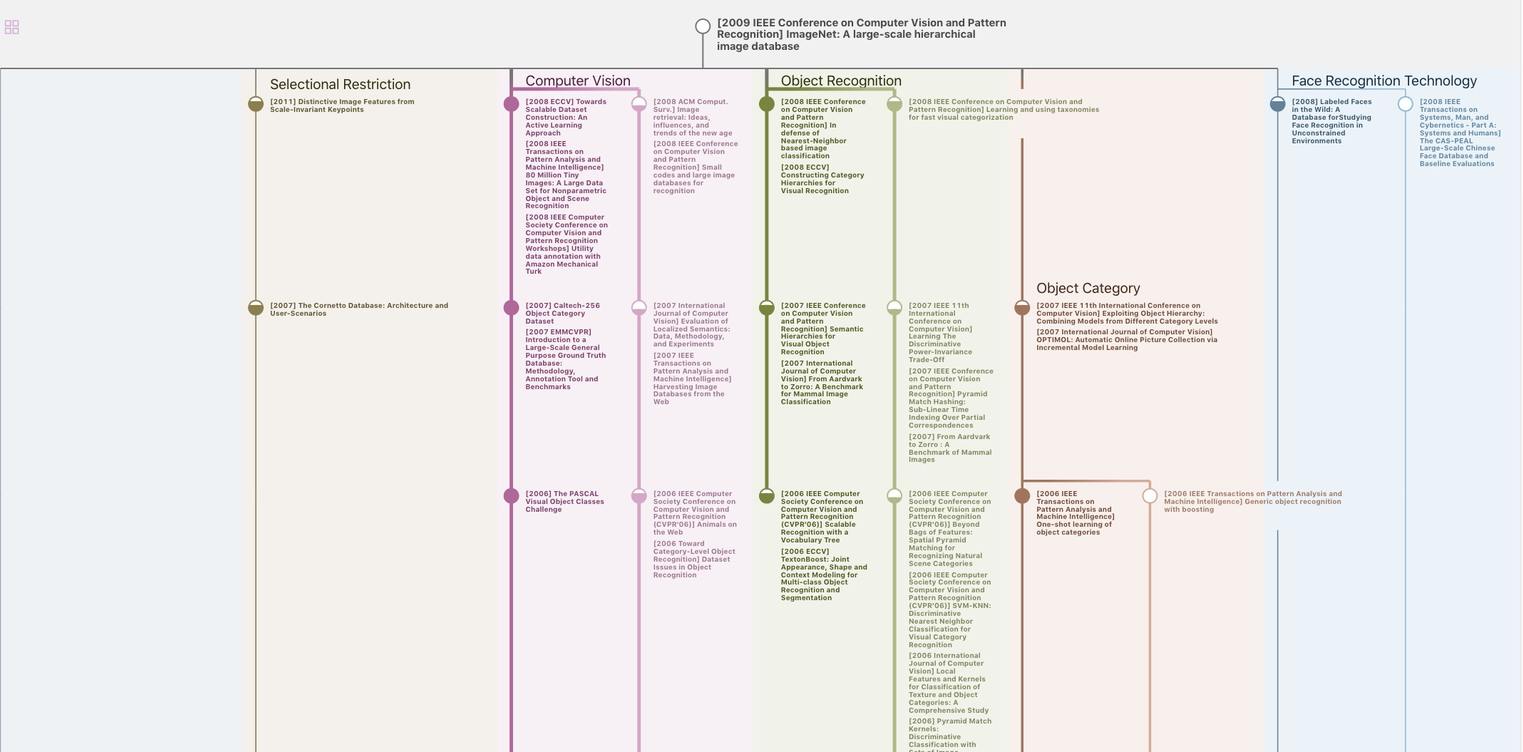
生成溯源树,研究论文发展脉络
Chat Paper
正在生成论文摘要