Enhancing LiDAR Point Cloud Segmentation with Synthetic Data.
MED(2023)
摘要
LiDAR point-cloud segmentation is a crucial issue for autonomous cars applications. The standard method for segmenting large-scale point clouds is to project 3D point cloud onto a 2D LiDAR image and apply convolutions to it. In this paper, we also follow this method and we want to detect and classify occurrences of road-objects, namely cars, cyclists, and pedestrians. To achieve this goal, we adapted the SqueezeSeg deep neural network.To address the challenge of obtaining labeled data for training autonomous driving systems, we used the CARLA autonomous driving simulator to generate a synthetic dataset in a simulation environment. The proposed network is initially trained on real-world LiDAR point-cloud data acquired from the KITTI dataset. Then, we created a synthetic dataset using the CARLA autonomous driving simulator in order to obtain more data and determine its impact on the validation accuracy of real-world data. To compare our current work to earlier work, we employ the same method. Our synthetic dataset has additional classes, such as cyclists and pedestrians, and when combined with real-world data, it significantly improves validation accuracy for each class, surpassing previous work. This demonstrates the effectiveness of our approach in detecting and classifying road-objects using LiDAR point-clouds, which is essential for the safe operation of autonomous vehicles.
更多查看译文
关键词
Semantic Segmentation,LiDAR Point Cloud Segmentation,Spherical Projection,CARLA Simulator,CNN,Conditional Random Field
AI 理解论文
溯源树
样例
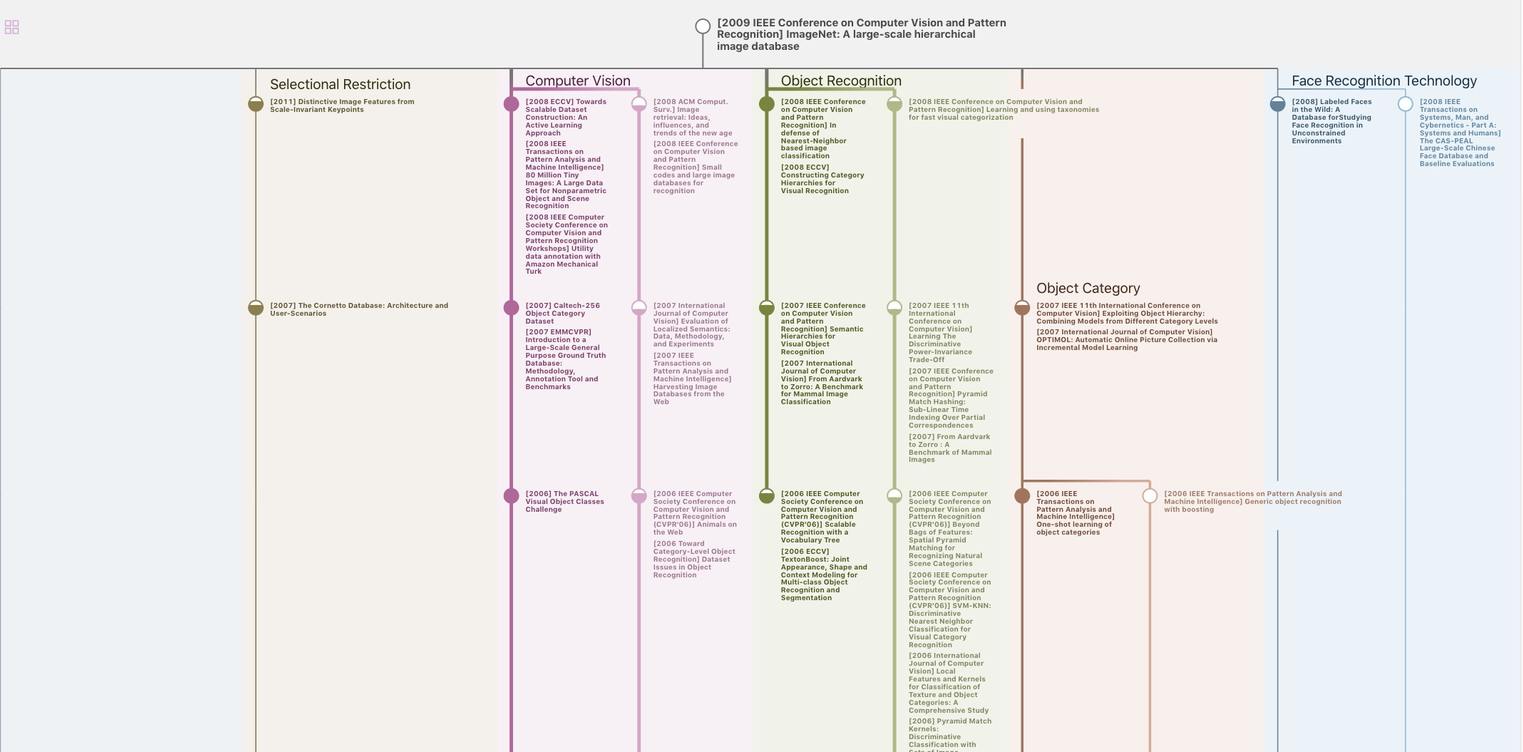
生成溯源树,研究论文发展脉络
Chat Paper
正在生成论文摘要