Federated Learning-Based In-Network Traffic Analysis on IoT Edge
2023 IFIP NETWORKING CONFERENCE, IFIP NETWORKING(2023)
摘要
The rise of IoT-connected devices has led to an increase in collected data for service and traffic analysis, but also to emerging threats and attacks. In-network machine learning-based attack detection has proven effective in fast response, but scaling to distributed IoT edge devices risks increasing communication overheads and raising data privacy concerns. To address these concerns, we present FLIP4, a distributed in-network attack detection framework based on federated tree models. FLIP4 maintains data privacy by enabling distributed machine learning training while keeping data local on IoT edge, and provides in-network inference within the programmable data plane on edge gateway for timely attack labeling and mitigation. Evaluation results show that FLIP4 can accurately detect attacks while maintaining source data privacy and enabling lightweight deployment on IoT edge.
更多查看译文
关键词
In-Network Computing,Federated Learning,Security,Internet of Things,P4
AI 理解论文
溯源树
样例
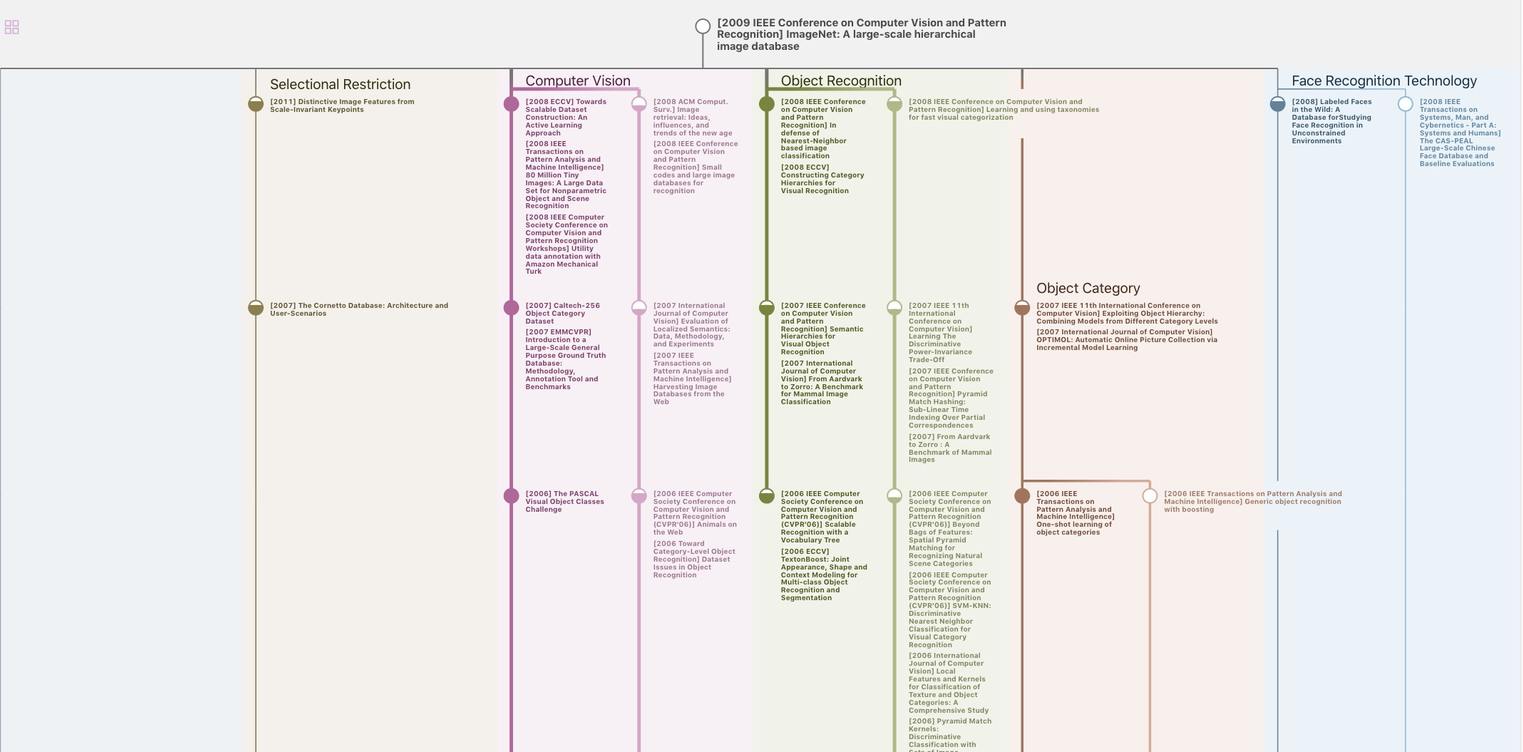
生成溯源树,研究论文发展脉络
Chat Paper
正在生成论文摘要