FSTC: Dynamic Category Adaptation for Encrypted Network Traffic Classification.
IFIP Networking(2023)
摘要
With the advancement in security and privacy on the Internet, network traffic has become increasingly difficult to classify. Current deep learning (DL)-based encrypted network traffic classification approaches rely on protocol-specific features (e.g., TLS headers) and/or assume that the classification categories (i.e., applications) remain constant over time. However, both the encryption protocols and applications continue to evolve. Therefore, DL models must be retrained from scratch for newer encryption protocols or applications, which makes existing approaches intractable in practice. In this paper, we propose novel Transfer Learning (TL) approaches for introducing new traffic classes to DL models without retraining them from scratch. We also propose a framework named FSTC, which leverages Active Learning (AL) to achieve human-assisted TL for new traffic classes and minimizes the labeled data needed for encrypted network traffic classification. We evaluate our TL and AL approaches using protocol-agnostic features from the publicly available ISCXVPN2016 and QUIC datasets. To the best of our knowledge, neither proposal has been explored before in the existing literature.
更多查看译文
关键词
Encrypted Traffic Classification,Deep Learning,Active Learning,Transfer Learning,Web Traffic
AI 理解论文
溯源树
样例
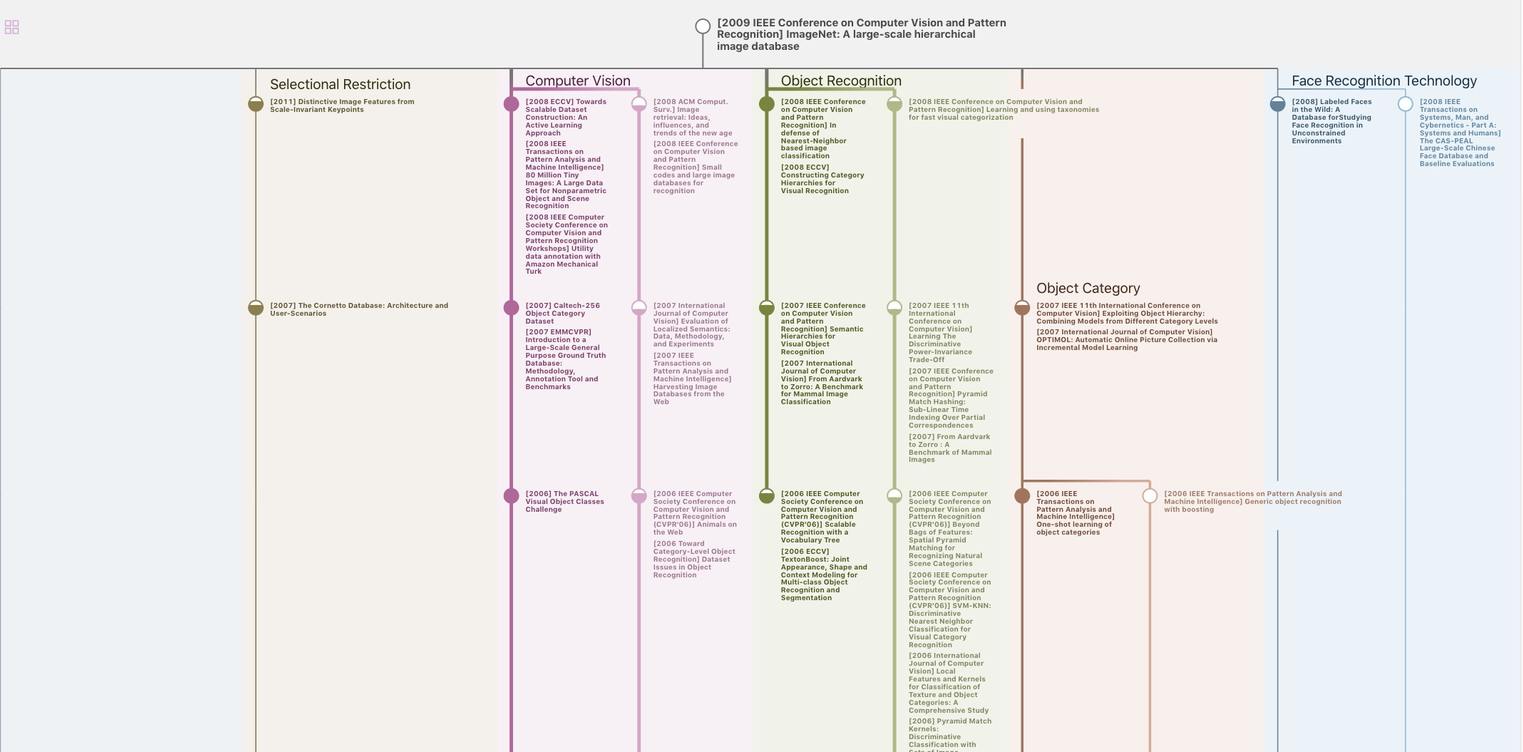
生成溯源树,研究论文发展脉络
Chat Paper
正在生成论文摘要