NCOD: Near-Optimum Video Compression for Object Detection.
ISCAS(2023)
摘要
With the emergence of technologies like smart cities, Internet of things (IoT), and 5G, the amount of produced visual data at the edges and remote nodes has exploded. Since for a considerable portion of the captured video the target is a machine learning task, rather than a human audience, transmission of videos in such applications requires efficient video compression tailored for machine vision. However, existing compression solutions are optimized for human vision. This paper presents a methodology to optimize an existing video compression standard, HEVC, for a machine vision task, Object Detection (OD). To this end, (1) a dataset of compressed videos, including several compression-ratios and their corresponding OD performance is collected to enable modeling, (2) A trade-off point (knee-point) between bitrate and OD performance is defined, that finds the point after which no major improvements will be achieved, (3) a set of features were extracted and studied to model this point, via a practical machine learning method. The resulting solution can predict the knee-point with MAE=1.28, resulting in a.Recall of only 0.012 and bitrate reduction of 86.56%, compared to OD with very high-quality video.
更多查看译文
关键词
Video coding,Video coding for machine (VCM),CRF,Object Detection,JND
AI 理解论文
溯源树
样例
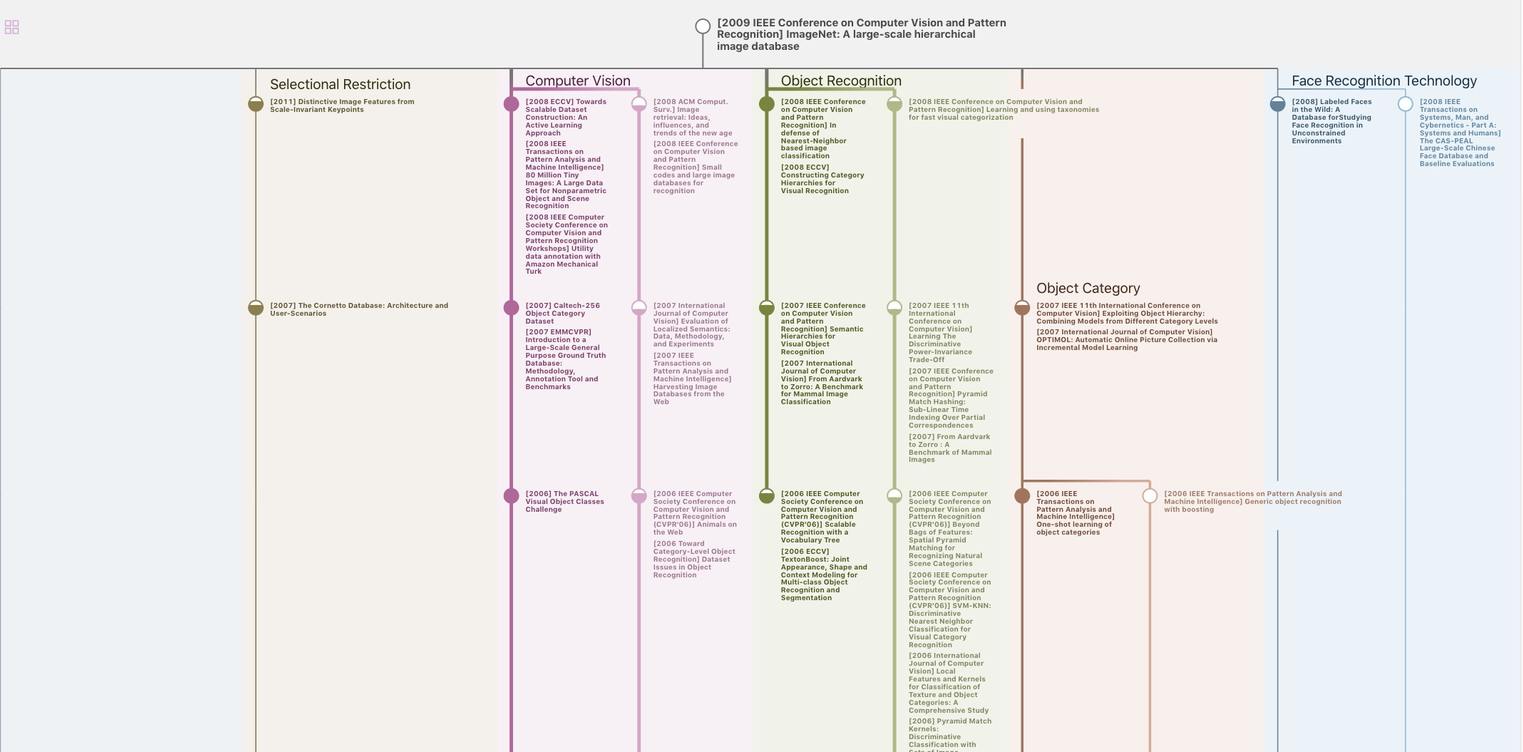
生成溯源树,研究论文发展脉络
Chat Paper
正在生成论文摘要