ANN Inference enabled by Variability Mitigation using 2T-1R Bit Cell-based Design Space Analysis.
ISCAS(2023)
摘要
Resistive RAM (RRAM) devices are compact and easy to fabricate with electrical inputs-based switching. Conductive-Bridge RRAM (CBRAM) is being developed to meet retention, endurance and reliability specifications by GlobalFoundries for typical 1-bit per cell digital storage. However, filamentary growth and rupture produce variability, and the resistance states can often span multiple orders. We explore whether digital-storage-focused CBRAM can support analog current readout-based Multiply-and-Accumulate operations in artificial neural network (ANN) applications. We explore the 2T-1R bit cell to tune the mean HRS/LRS ratio and to control the variability in HRS and LRS readouts. We use experimental CBRAM data and GlobalFoundries' 22FDX platform and demonstrate > 2 x reduction in HRS and LRS logscale variability and >10 x higher HRS/LRS ratio for the 2T-1R bit cell. The strategy is successfully tested for two datasets - the simpler MNIST and the more complex FMNIST using system-level modeling of non-idealities like weight quantization, HRS/LRS ratio, and variability in the readout of each bit-cell. Such bit-cell design principles have general utility in exploiting variability-prone characteristics of emerging memories for excellent application-level performance.
更多查看译文
关键词
inference, bit cell design, 2T-1R, RRAM, variability
AI 理解论文
溯源树
样例
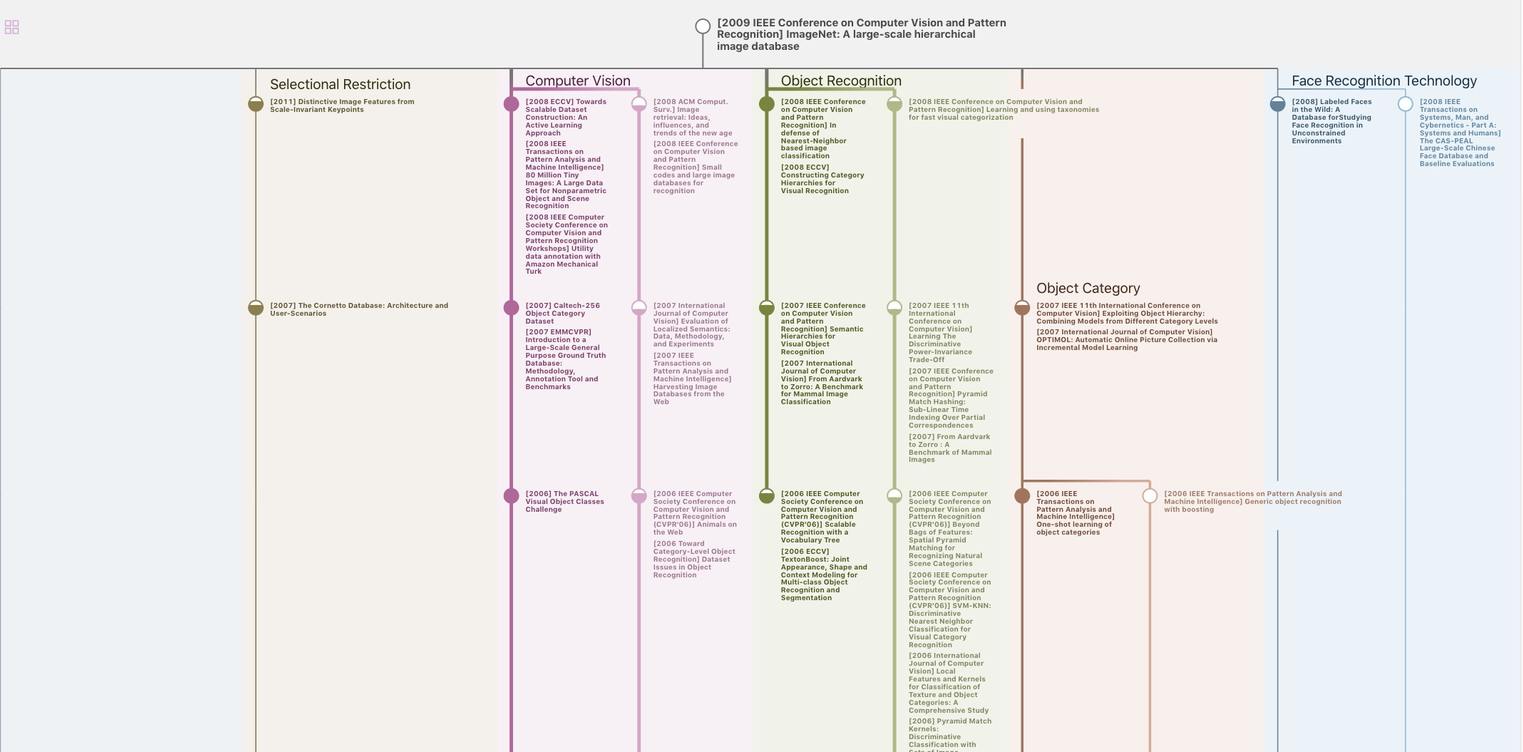
生成溯源树,研究论文发展脉络
Chat Paper
正在生成论文摘要