LRCN-based Noninvasive Blood Glucose Level Estimation.
ISCAS(2023)
摘要
Obtaining accurate assessments of blood glucose level (BGL) by any means that can be recorded easily in real-time has been a trending research focus area in recent years. Indeed, BGL indicators can help identify health risks associated with diabetes mellitus or provide efficient assistance and monitoring to patients diagnosed with diabetic conditions. However, the conventional methods of measuring blood glucose level require an invasive blood sampling technique to analyze the proportion of glucose in blood components. This method causes discomfort and relatively bulky equipment to remain available as portable systems. In this work, we proposed a system that uses long-term recurrent convolutional networks (LRCN) using non-invasive biomedical signal recording to estimate the blood glucose level. The proposed LRCN is combined with a robust feature extraction mechanism and can benefit from the temporal sensitivity of long short-term memory, to find a mathematical relationship between a photoplethysmogram (PPG) and blood glucose levels. The proposed system achieved 4.7 and 11.146 as mean absolute error (MAE) and root mean squared error (RMSE), respectively, on the predicted BGL values in comparison with standard BGL invasive blood sample recordings device values.
更多查看译文
关键词
BGL invasive blood sample recording device,blood components,blood glucose levels,invasive blood sampling technique,LRCN-based noninvasive blood glucose level estimation,mean absolute error,photoplethysmogram,root mean squared error
AI 理解论文
溯源树
样例
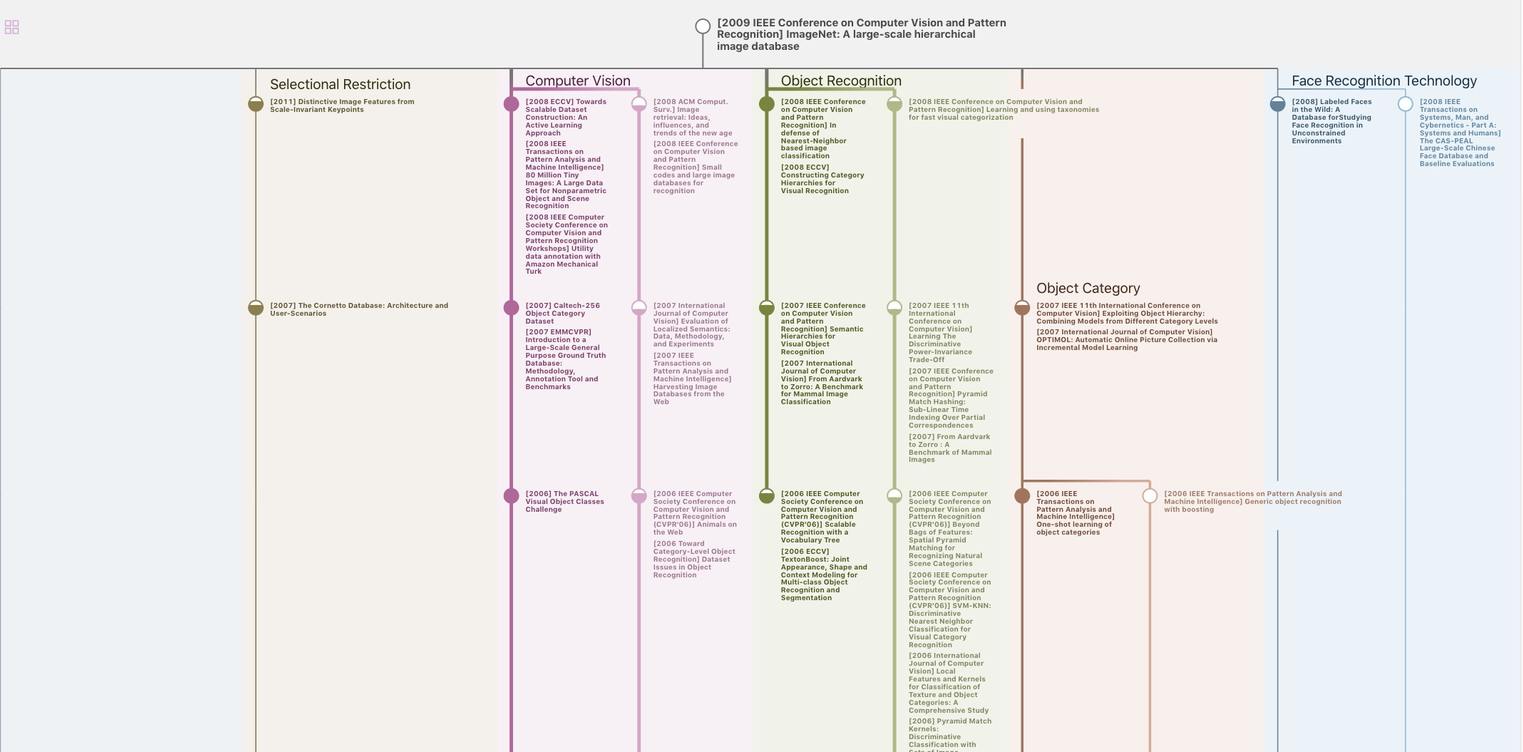
生成溯源树,研究论文发展脉络
Chat Paper
正在生成论文摘要