Resource-efficient Face Detector Using 1.5-bit Frame-to-frame Delta Quantization for Image Based Always-on Wake-up Application
ISCAS(2023)
摘要
A resource-efficient neural-network-based face detector using 1.5-bit frame-to-frame delta quantization with diagonal spatial feature extraction method is proposed in this paper, which is designed for resource-limited always-on camera sensors. The proposed architecture completes analog-domain frame difference for motion sensing, which triggers digital-domain feature extraction. Based on the sparse and effective features, a lightweight convolutional neural network is devised as a classifier. A self-recorded dataset of 313 videos for humans of different appearances, light intensity and backgrounds is used to validate the performance of the proposed method. Simulation results show that the proposed method achieves 93.6% accuracy using only a
$\boldsymbol{50\times 50}$
pixel array, which is higher than the prior discontinuous temporal change quantization method. Meanwhile, the conservatively estimated power consumption of the proposed method can be reduced by
$\mathbf{14 \times}$
compared to the state-of-the-art work.
更多查看译文
关键词
face detector,low power,frame-to-frame delta quantization,feature extraction,lightweight neural network
AI 理解论文
溯源树
样例
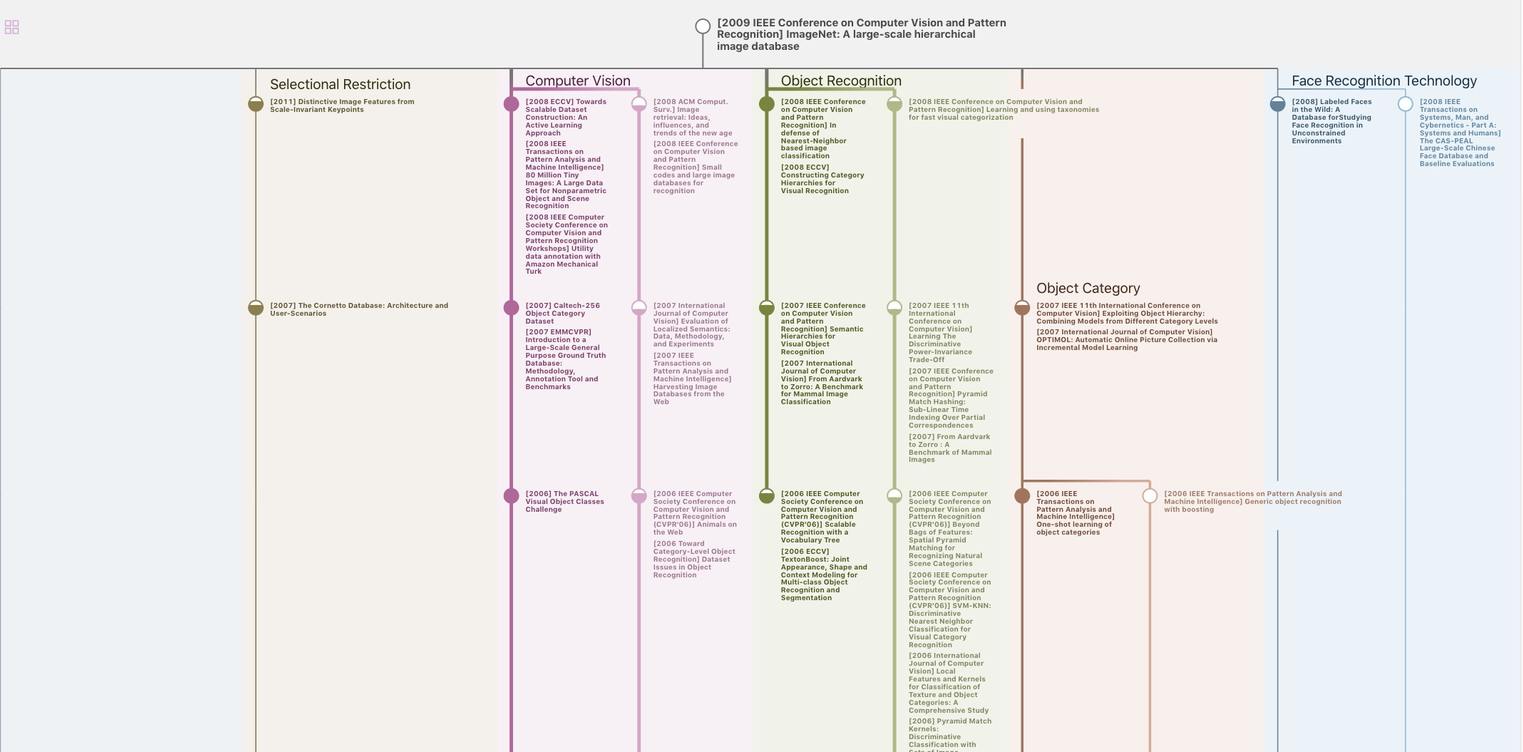
生成溯源树,研究论文发展脉络
Chat Paper
正在生成论文摘要