Poster: Quantifying Signal Quality Using Autoencoder for Robust RF-based Respiration Monitoring.
CHASE(2023)
摘要
While radio frequency (RF) based respiration monitoring for at-home health screening is receiving increasing attention, robustness remains an open challenge. In recent work, deep learning (DL) methods have been demonstrated effective in dealing with non-linear issues from multi-path interference to motion disturbance, thus improving the accuracy of RF-based respiration monitoring. However, such DL methods usually require large amounts of training data with intensive manual labeling efforts, and frequently not openly available. We propose RF-Q for robust RF-based respiration monitoring, using self-supervised learning with an autoencoder (AE) neural network to quantify the quality of respiratory signal based on the residual between the original and reconstructed signals. We demonstrate that, by simply quantifying the signal quality with AE for weighted estimation we can boost the end-to-end (e2e) respiration monitoring accuracy by an improvement ratio of 2.75 compared to a baseline.
更多查看译文
关键词
Vital signs monitoring,RF sensing,signal quality assessment,signal reconstruction,unsupervised learning,autoencoder (AE)
AI 理解论文
溯源树
样例
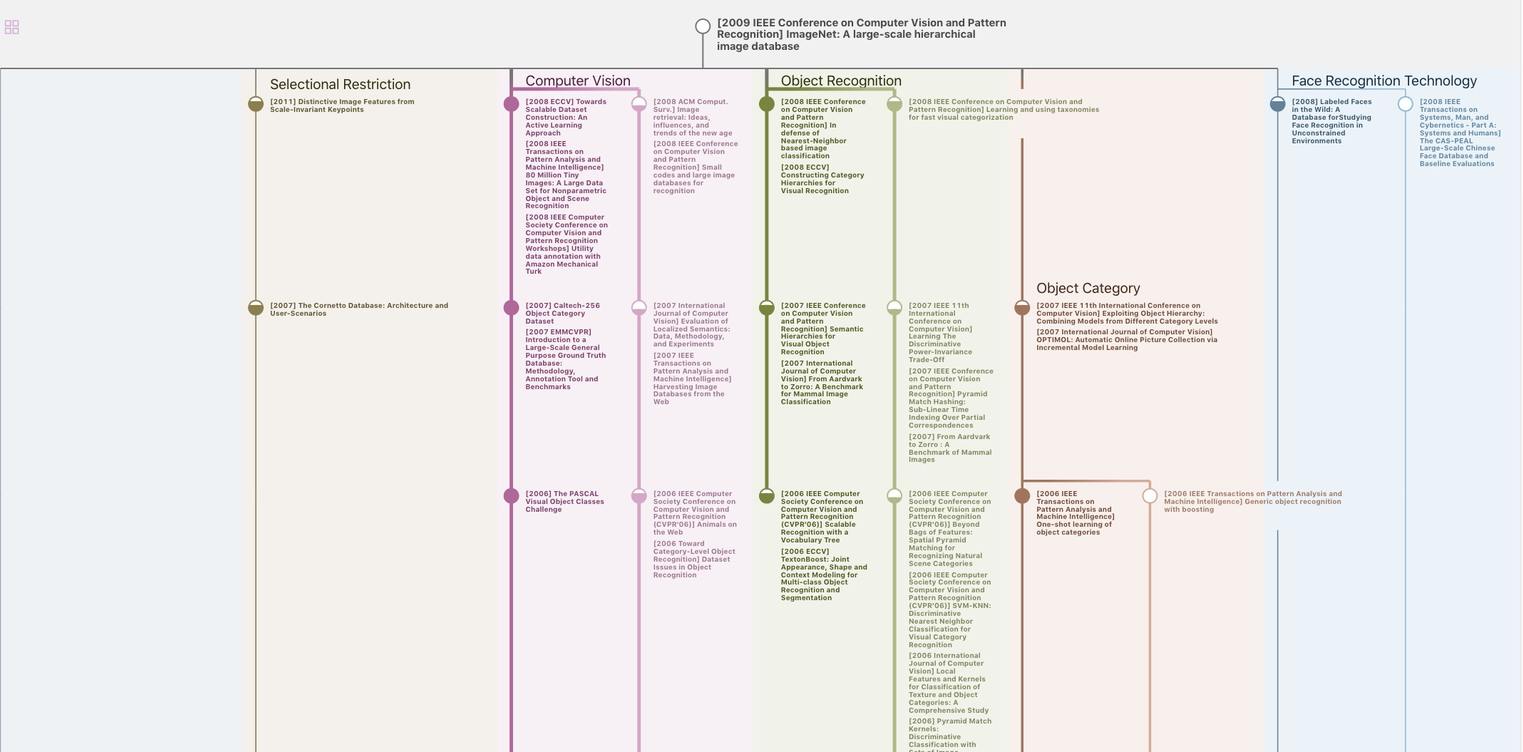
生成溯源树,研究论文发展脉络
Chat Paper
正在生成论文摘要