Fault diagnosis study of mine drainage pump based on MED–WPD and RBFNN
Journal of the Brazilian Society of Mechanical Sciences and Engineering(2023)
摘要
Mine drainage pumps typically operate in the strong background noise environment of underground coal mines, and the vibration sensor is usually mounted outside the pump housing. This makes the large values noise and the high-frequency noise contained in the vibration signals. The energy of the early weak fault vibration signal gradually decays in a complex transmission path and is finally drowned out by strong background noise. Therefore, the extraction of fault features for mine drainage pumps is difficult. To solve this problem, a fault diagnosis method of mine drainage pumps based on minimum entropy deconvolution (MED)–wavelet packet decomposition (WPD) and radial basis function neural network (RBFNN) is proposed. First, the vibration signal is noise-reduced by the MED to reduce noise interference, and the fault signal characteristics are highlighted. Then, the noise-reduced signal is analyzed and processed by WPD, and each fault feature is extracted. Finally, the above fault characteristics are fused with the temperature and pressure signals. The RBFNN is trained by the above eigenvectors, and the test arrays are input into the trained RBFNN to obtain fault diagnosis results. The research result shows that the method has a high recognition accuracy, with a recognition accuracy of 94.44%, and the types of faults in mine drainage equipment can be effectively identified.
更多查看译文
关键词
Mine drainage pump,MED,WPD,RBFNN,Fault diagnosis
AI 理解论文
溯源树
样例
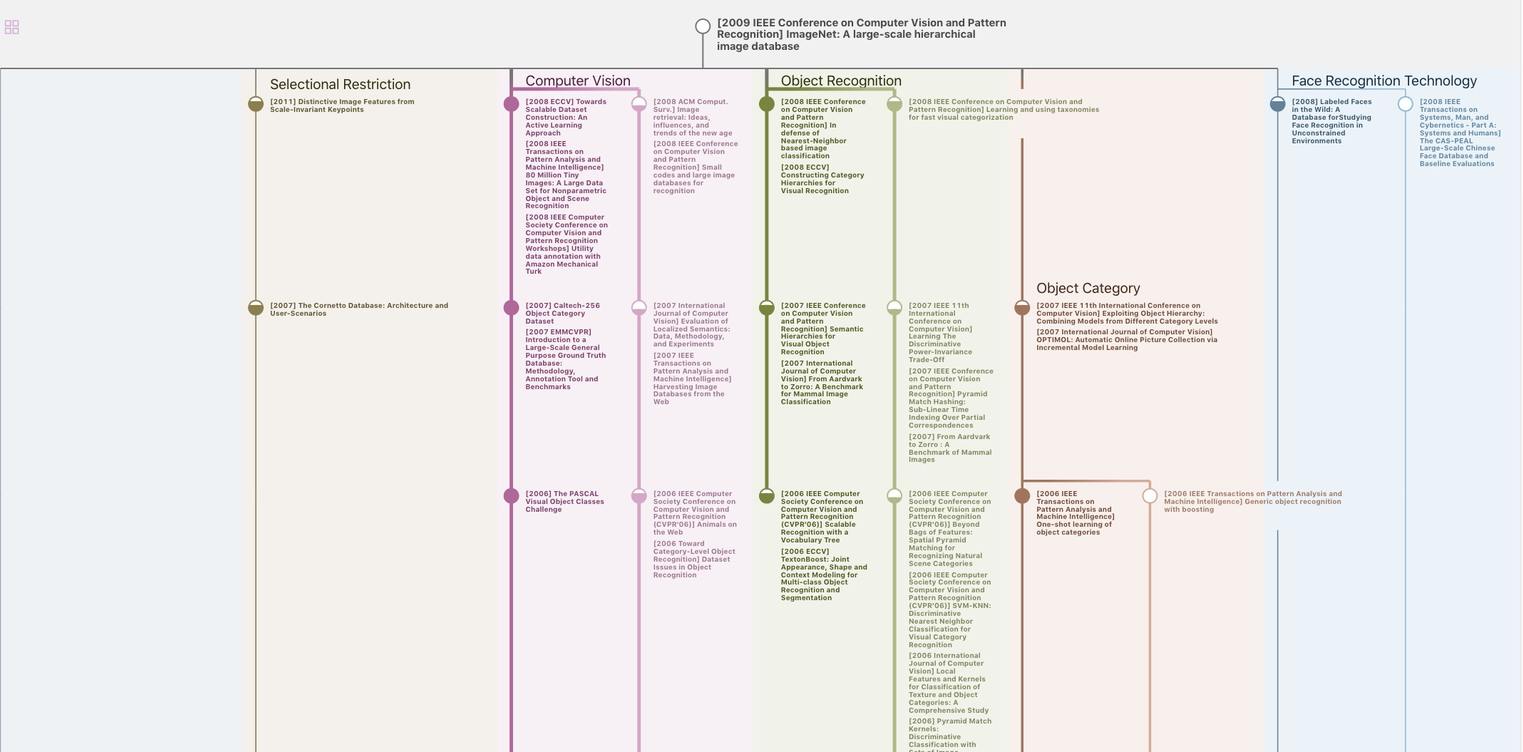
生成溯源树,研究论文发展脉络
Chat Paper
正在生成论文摘要