Stochastic Unit Commitment: Model Reduction via Learning
Current Sustainable/Renewable Energy Reports(2023)
摘要
Purpose of Review As weather-dependent renewable generation increases its share in the generation mix of most electric energy systems, a stochastic unit commitment becomes the natural day-ahead scheduling tool. However, such a tool is generally computationally intractable if a detailed uncertainty description is considered. Taking this into account, we proposed a learning method to make the stochastic unit commitment problem tractable. Recent Findings Recent advances in statistical learning and machine learning to address optimization problems can be advantageously applied to the rather intractable stochastic unit commitment problem. Considering these advances, we explore simple learning techniques to drastically reduce the size of a stochastic unit commitment problem without significantly altering its optimal solution. Summary The considered stochastic unit commitment problem is formulated as a two-stage stochastic programming problem. The first stage represents commitment decisions, while the second one represents the operation conditions under different scenarios. Taking into account historical solved instances (or proxies for them), we reduce the size (measured by numbers of constraints and variables) of the stochastic unit commitment problem by (i) fixing unchanged binary variables and by (ii) eliminating inactive inequality constraints. Our numerical results show that the reduced problem generally requires significantly less time to solve while obtaining high-quality solutions, which are very close to or indistinguishable from the one obtained by solving the original problem. We use an Illinois 200-bus system to illustrate and characterize the performance of the proposed problem-reduction method.
更多查看译文
关键词
Stochastic unit commitment,Learning,Stochastic programming,Mixed-integer linear programming
AI 理解论文
溯源树
样例
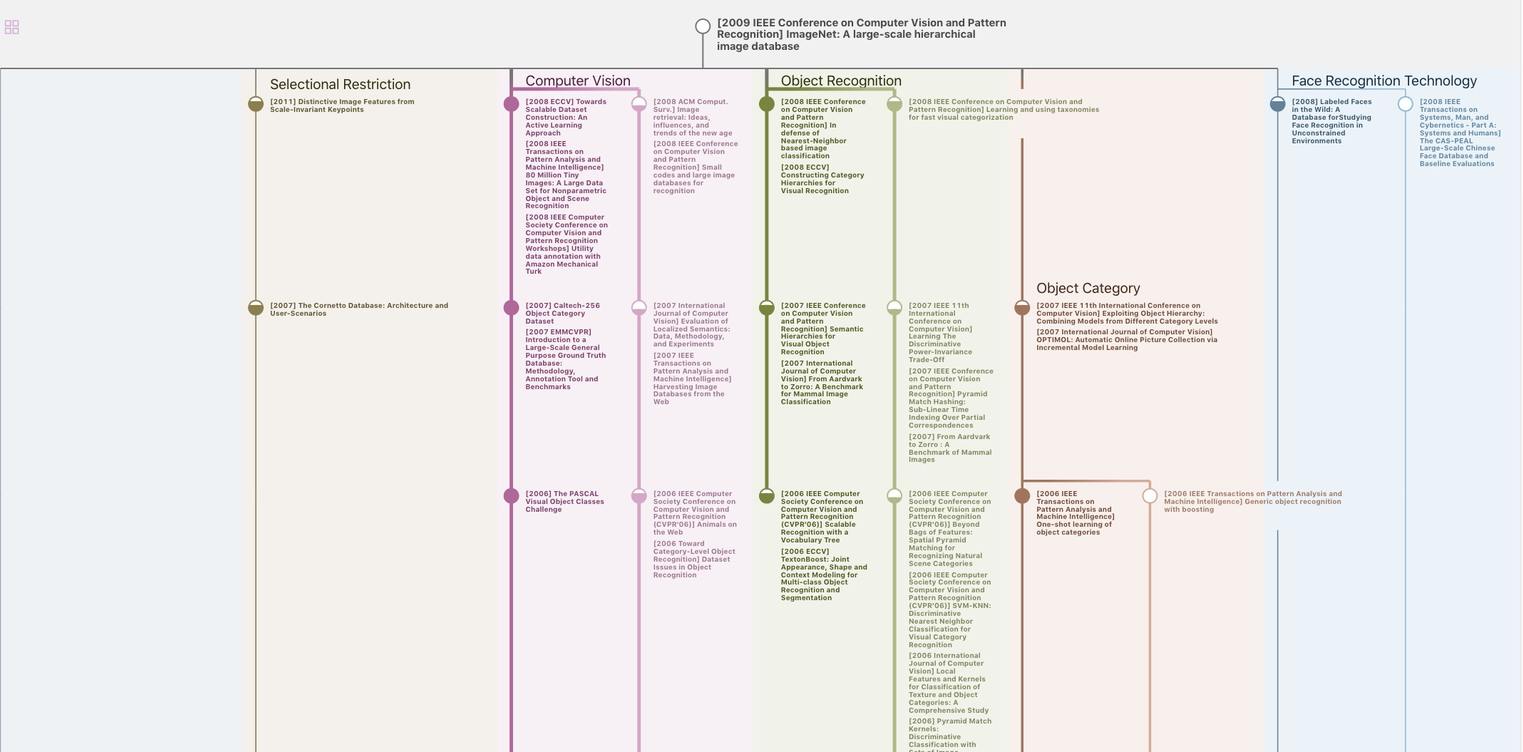
生成溯源树,研究论文发展脉络
Chat Paper
正在生成论文摘要