Detection of atrial fibrillation based on Stockwell transformation using convolutional neural networks
International Journal of Information Technology(2023)
摘要
Atrial Fibrillation (AF) is a pervasive cardiac cantering rhythm that is harmful and causes heart-related complications. AF causes an irregular heartbeat, which may show up at intervals known as proximal AF or for a long duration known as persistent AF. Such irregularities lead to the unhealthy functioning of the heart and cause various cardiac problems. So, the early detection of AF can help in planning efficient treatment, though automatic and early detection of AF is a challenging task. In this study presents a novel approach to detecting AF signals from electrocardiogram (ECG) signals that is based on the combination of a Stockwell Transformation (ST) technique with a 2-D convolutional neural network (CNN). These traditional AF detection methods are used for the isolation of ventricular and atrial activities. The proposed method uses the MIT-BIH database of AF and normal sinus rhythm (NSR) for training and testing. However, the proposed method analyses the time–frequency features of the ECG, and to improve the prior AF detection, a 2D-CNN model is trained and tested for the overall accuracy (Acc), sensitivity (Se), specificity (Sp), and positive predictive value (PPV) performance measures. The results indicated that the proposed method improved the overall performance significantly, providing better accuracy and precision compared to other existing models. The experimental results performed on the proposed approach provide significant improvements in Acc, Se, Sp, and PPV of 99.543%, 99.50%, 99.58%, and 99.57%, respectively. The proposed method can be used in the emergency healthcare department, and it helps cardiologists in the accurate detection of heart problems.
更多查看译文
关键词
Atrial fibrillation,Stockwell transformation,2D-CNN,Time–frequency features
AI 理解论文
溯源树
样例
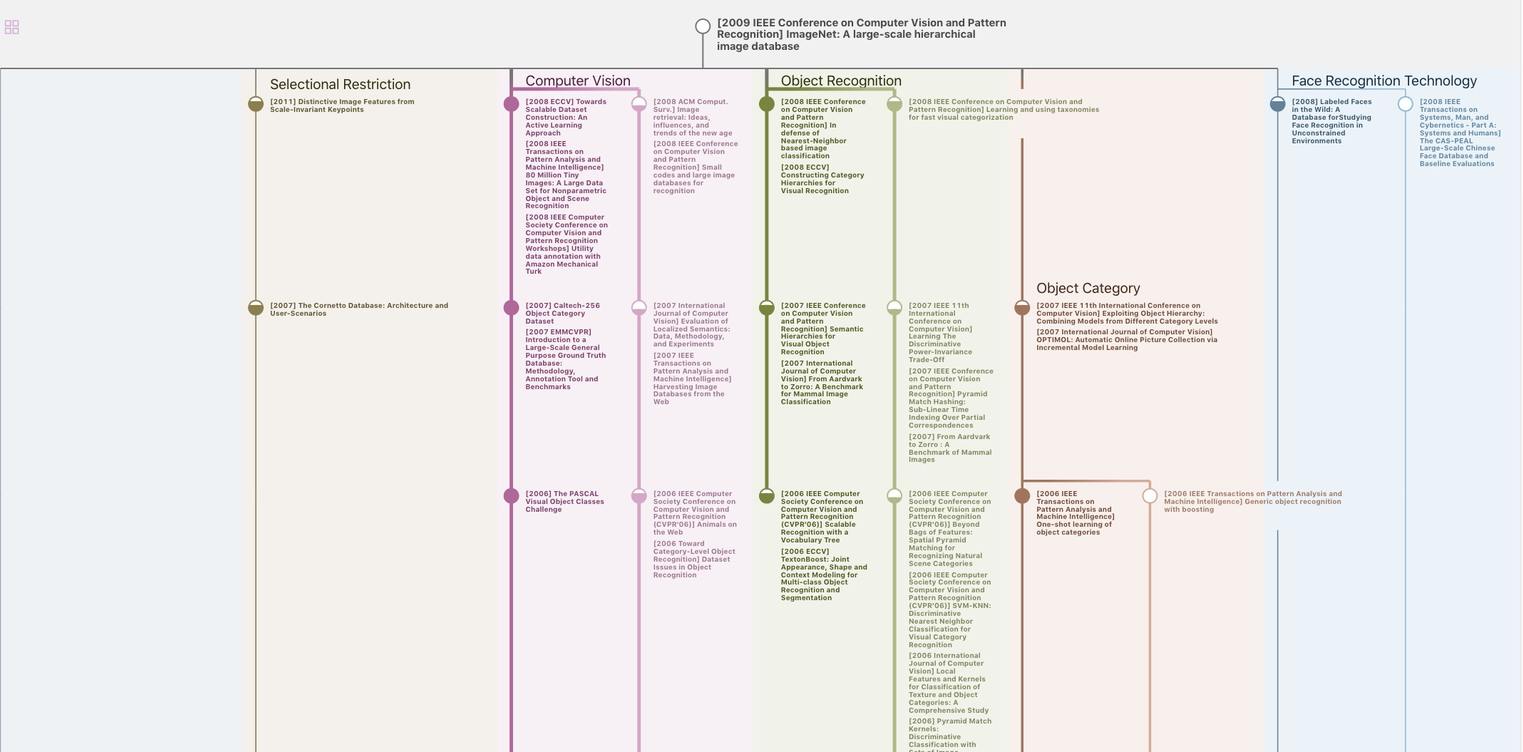
生成溯源树,研究论文发展脉络
Chat Paper
正在生成论文摘要