Automatic feature selection using enhanced dynamic Crow Search Algorithm
International Journal of Information Technology(2023)
摘要
Data clustering is an unsupervised machine-learning technique that segregates similar data points. Most real-life datasets have redundant and irrelevant features, which deteriorate the clustering accuracy. Feature selection selects the most relevant subset of features, which improves clustering accuracy and reduces execution time. Different swarm and evolutionary-based algorithms are known to give an optimal solution for complex optimization problems like clustering and feature selection. The present work employs a wrapper-based feature selection technique. It incorporates an evolutionary search-based algorithm for simultaneous clustering and feature selection. An Enhanced Dynamic Cluster Search Algorithm (EDCSA) that wraps the K-means method to pick optimal features for clustering has been proposed. The results are compared with other works using various cluster validity indices. The results show that the EDCSA performs better at selecting relevant features, improving overall accuracy.
更多查看译文
关键词
Feature selection,Clustering,Machine-learning,Meta-Heuristic algorithm
AI 理解论文
溯源树
样例
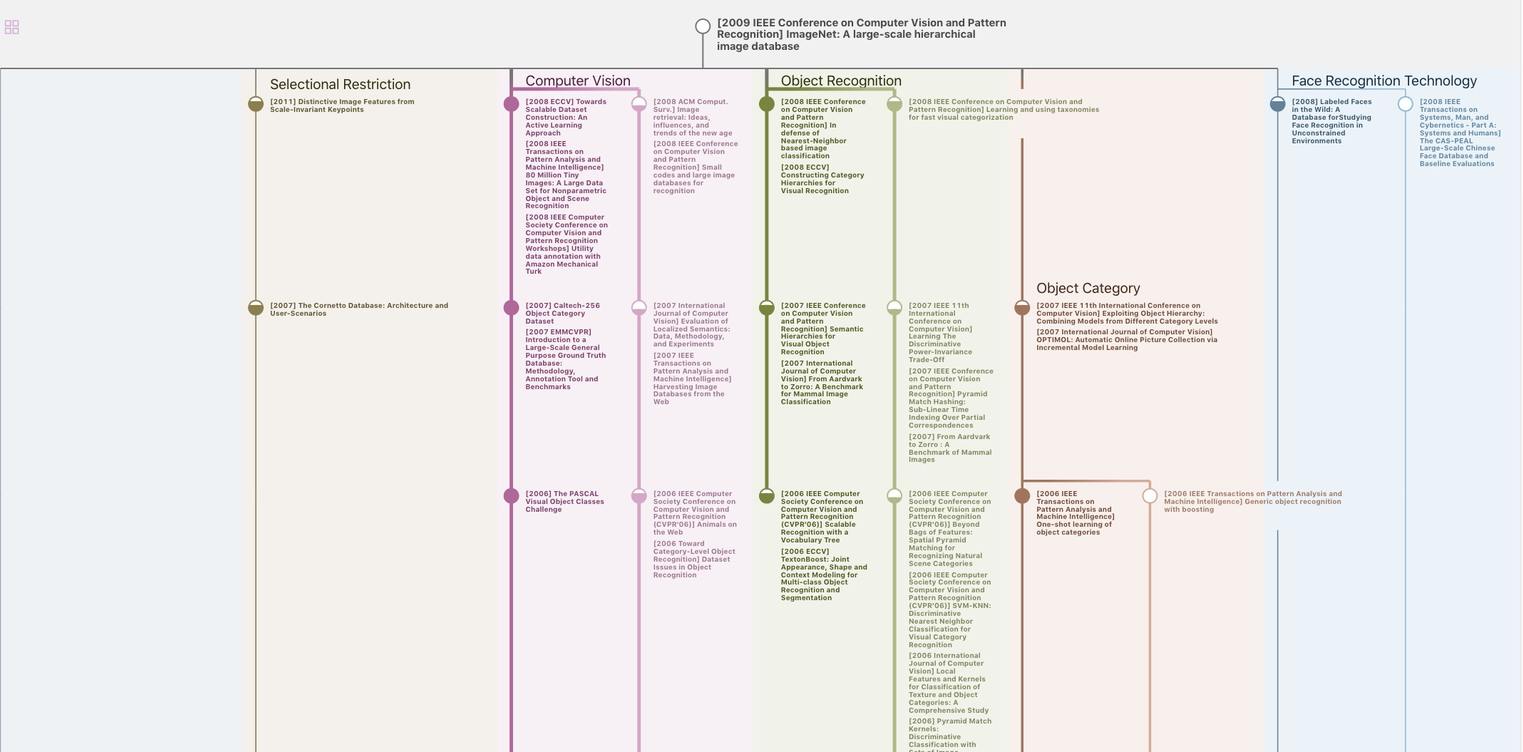
生成溯源树,研究论文发展脉络
Chat Paper
正在生成论文摘要