Task-Adaptive Feature Disentanglement and Hallucination for Few-Shot Classification
IEEE Transactions on Circuits and Systems for Video Technology(2023)
摘要
Few-shot classification is a challenging task of computer vision and is critical to the data-sparse scenario like rare disease diagnosis. Feature augmentation is a straightforward way to alleviate the data-sparse issue in few-shot classification. However, mimicking the original feature distribution from a small amount of data is challenging. Existing augmentation-based methods are task-agnostic: the augmented feature is not with optimal intra-class diversity and inter-class discriminability concerning a certain task. To address this drawback, we propose a novel Task-adaptive Feature Disentanglement and Hallucination framework, dubbed TaFDH. Concretely, we first perceive the task information to disentangle the original feature into two components: class-irrelevant and class-specific features. Then more class-irrelevant features are decoded from a learned variational distribution, fused with the class-specific feature to get the augmented features. Finally, a generalized prior distribution over a quadratic classifier is meta-learned, which can be fast adapted to the class-specific posterior, thus further alleviating the inadequacy and uncertainty of feature hallucination via the nature of Bayesian inference. In this way, we construct a more discriminable embedding space with reasonable intra-class diversity instead of simply restoring the original embedding space, which can lead to a more precise decision boundary. We obtain the augmented features equipped with enhanced inter-class discriminability by highlighting the most discriminable part while boosting the intra-class diversity by fusing with the diverse generated class-irrelevant parts. Experiments on five multi-grained few-shot classification datasets demonstrate the superiority of our method.
更多查看译文
关键词
Few-shot classification, meta learning, task adaptation, feature augmentation, variational inference, Bayesian inference
AI 理解论文
溯源树
样例
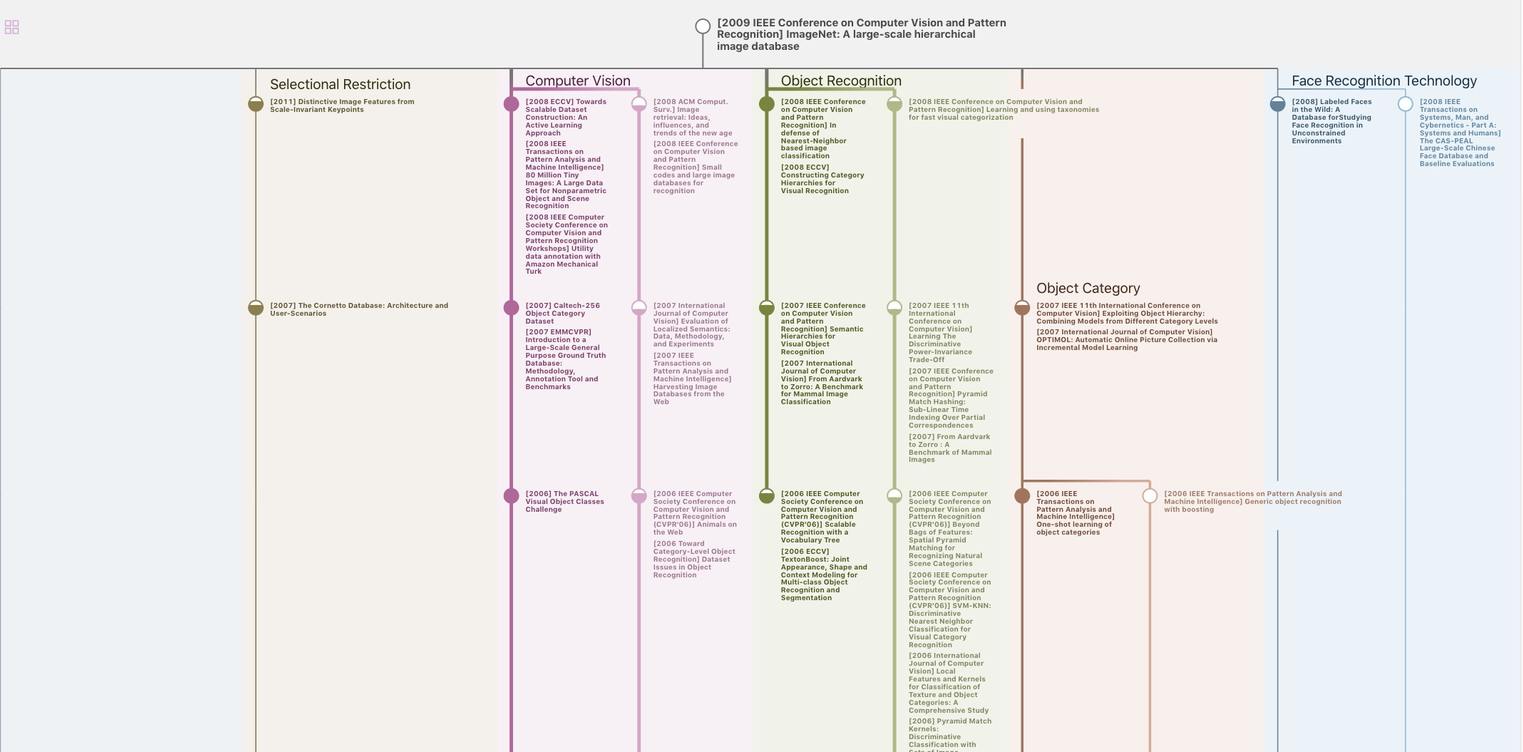
生成溯源树,研究论文发展脉络
Chat Paper
正在生成论文摘要