Active Learning With Co-Auxiliary Learning and Multi-Level Diversity for Image Classification
IEEE Transactions on Circuits and Systems for Video Technology(2023)
摘要
Due to the fact that it is expensive and time-consuming to annotate a large amount of data, the available labeled data to train a deep neural network is usually scarce, resulting in the poor performance of the deep classification network in most cases. In this paper, we propose a novel active learning method to train a competitive deep classification neural network by labeling a limited amount of diverse images. The proposed method advances most of the active learning methods in two aspects. First, active learning is enhanced with a co-auxiliary learning strategy. We use an auxiliary network to provide diverse pseudo-labels for the primary network. The auxiliary network is also adopted to remove a part of redundancy information from the candidate pool of active learning when image predictions of the primary network and the auxiliary network are the same. Meanwhile, the primary network can also provide pseudo-labels to improve the performance of the auxiliary network. Second, we further remove the redundancy within the query samples with multi-level diversity selection. The multi-level diversity strategy not only considers the feature-level diversity but also the class-level diversity with the predictions of the primary network, and it can select both representative and uncertain samples effectively and efficiently from the large-scale data. The extensive experiments on four large-scale datasets show that the proposed method outperforms the state-of-the-art methods.
更多查看译文
关键词
Active learning, auxiliary learning, diversity, image classification
AI 理解论文
溯源树
样例
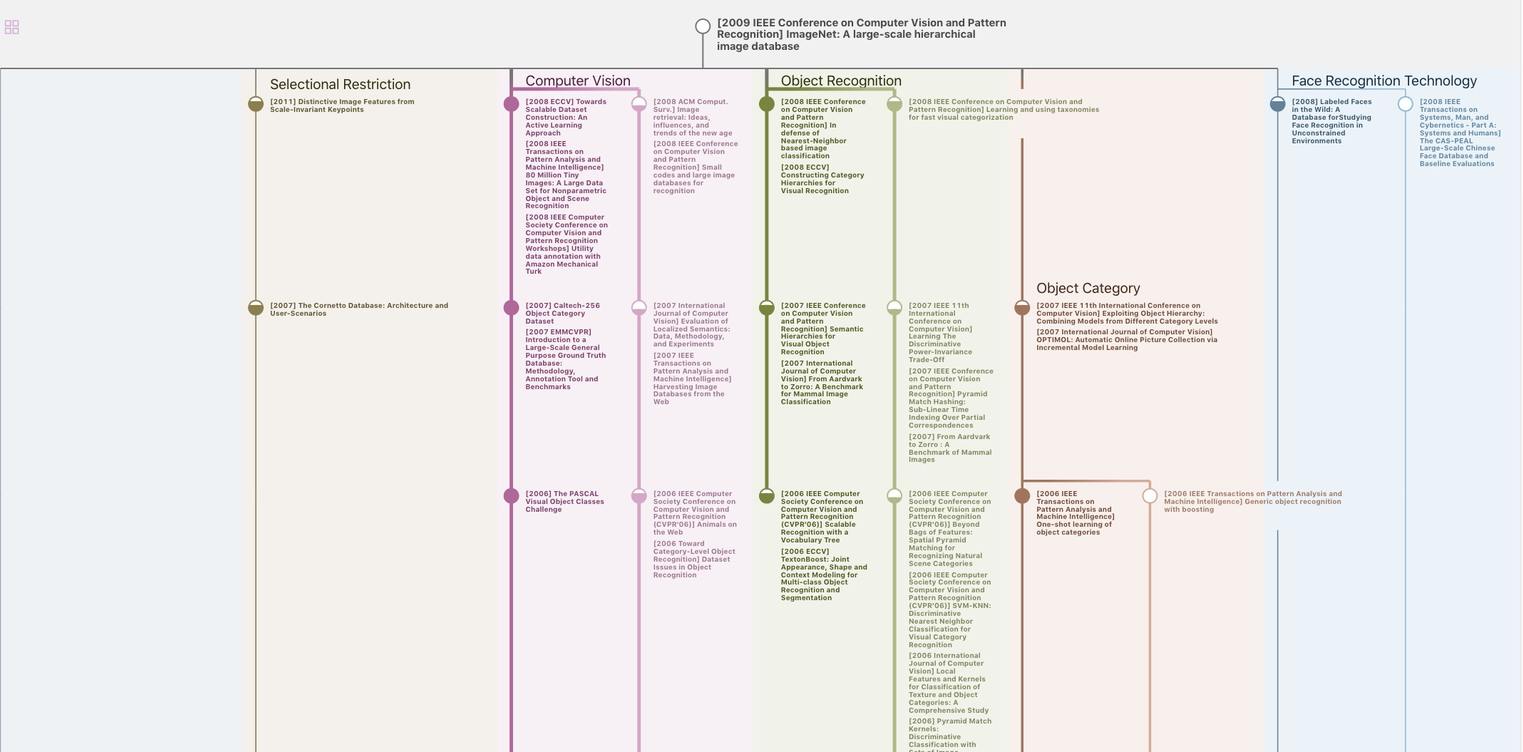
生成溯源树,研究论文发展脉络
Chat Paper
正在生成论文摘要