Unleashing the Potential of Systems Modeling and Simulation in Supporting Policy-Making and Resource Allocation for Suicide Prevention
Crisis(2023)
摘要
Free AccessUnleashing the Potential of Systems Modeling and Simulation in Supporting Policy-Making and Resource Allocation for Suicide PreventionMaria Michail and Katrina WittMaria MichailMaria Michail, School of Psychology, Institute for Mental Health, University of Birmingham, Edgbaston B15 2TT, Birmingham, UK, [email protected]School of Psychology, Institute for Mental Health, University of Birmingham, UKSearch for more papers by this author and Katrina WittOrygen, Parkville, VIC, AustraliaCentre for Youth Mental Health, The University of Melbourne, Parkville, VIC, AustraliaSearch for more papers by this authorPublished Online:August 04, 2023https://doi.org/10.1027/0227-5910/a000905PDF ToolsAdd to favoritesDownload CitationsTrack Citations ShareShare onFacebookTwitterLinkedInReddit SectionsMoreOver 700,000 people die by suicide every year (World Health Organization [WHO], 2021). Despite increasing investment in suicide prevention activities from many governments worldwide, rates have continued to rise over the past 30 years (Yip et al., 2022). This is because suicide is complex and addressing this complexity is our Achilles heel. There are several factors that contribute to the complexity behind suicide. First and foremost, suicide is rarely the outcome of a single factor. Many suicide deaths are associated with a gradual and increasing build-up of vulnerability associated with multiple, interacting, and interrelated biological, psychological, socioeconomic, cultural, and political factors (Hawton et al., 2012). Put simply, there are many and different pathways that could lead to suicide. This equifinality means that mapping the causal processes and interactions that underlie suicidal behavior, at least within the context of the methodological approaches currently favored by the field (e.g., meta-analyses, regression models), can be very challenging (Franklin et al., 2017).Second, a multifaceted public health approach to suicide prevention requires planning and coordination between complex systems including health, social care, education, the justice system, and the voluntary sector (WHO, 2012). Each of these systems is characterized by multiple, interrelated entities including financial and human resources, organizational structures, funding models, and at the core of all, those with lived and living experience of self-harm/suicidal behavior, whose needs can change rapidly over time. When a system entails so many moving parts, it can be difficult to know when and how to intervene, what interventions to offer, for how long, and with what intensity to generate the most significant reductions in suicide rates (Occhipinti, Skinner, Iorfino et al., 2021). We therefore often resort to the deployment of comprehensive and multisectoral suicide prevention strategies (WHO, 2018), with the assumption that investing in more interventions or more policies across all levels (universal, selective, targeted) will simply confer better results (Occhipinti, Skinner, Iorfino et al., 2021; Occhipinti et al., 2022). The question remains, however, as to whether this is the most efficient and effective way of informing decision-making in suicide prevention policy and planning.The Role of Systems Thinking and Systems ModelingUnderstanding the dynamic complexity of the etiological factors underlying suicidal behavior within a complex and multifaceted public health framework requires a shift in our thinking, attitudes, and response. Systems thinking offers the potential for a more nuanced approach to suicide prevention by recognizing, mapping, and understanding the complex and dynamic interactions (i.e., interconnectedness) between variables within a system (Meadows, 2008). By “system” we mean “a set of elements or parts that is coherently organized and interconnected in a pattern or structure that produces a characteristic set of behaviours, often classified as its function or purpose” (Meadows, 2008, p. 11). For a system thinker, “a system isn’t just any old collection of things … a system is more than the sum of its parts” (Meadows, 2008, p. 11). One way of quantifying multiple, nonlinear, interacting components within a system is by using system dynamics modeling (SDM). SDM is a computer-assisted method that helps frame, test, and simulate the causal processes and interactions that underlie complex systems or behaviors to inform policy-making (Homer et al., 2006). SDM is an iterative process consisting of problem definition and model conceptualization, model formulation, model testing, and implementation (Forrester & Senge, 1980). SDM provides researchers, policy-makers, and others with a virtual environment in which the likely impacts of different interventions or policies on desired outcomes can be tested over time to understand with what intensity and for how long investments are required to sustain the effects of any given intervention (Atkinson et al., 2015).Over the past seven decades, SDM has been extensively used in many sectors including healthcare (Marshall et al., 2015), transport (Liu et al., 2015), and hydrology (Jeong & Adamowski, 2016). However, the adoption of SDM in the field of mental health, and more specifically suicide prevention, has been slower (Chung et al., 2022; Occhipinti, Skinner, Doraiswamy et al., 2021; Page et al., 2017, 2018; Rogers & Joiner, 2019; Skinner et al., 2021). This is beginning to change, however. In Australia, for example, SDM has been used as an analytical tool to shape regional (Page, Atkinson, Campos et al., 2018; Skinner et al., 2021) and national (Page et al., 2017) plans for suicide prevention. SDM has also been used to help us understand trajectories of suicidal thoughts and behaviors among adults (Rogers & Joiner, 2019) and adolescents (Chung et al., 2022). The potential of SDM in addressing the increasing suicide rates among young people has been recently highlighted (Occhipinti, Skinner, Iorfino et al., 2021).In view of this, this editorial seeks to (1) highlight the merit of adopting SDM to inform suicide prevention research, practice, and policy; (2) critically explore some of the caveats concerning the use of SDM for suicide prevention; and (3) identify future directions to maximize the potential of SDM.Benefits of Utilizing SDM for Suicide PreventionSDM addresses the limitations of current methodological approaches in suicide prevention (e.g., regression models), which primarily focus on identifying and analyzing suicide risk and protective factors independently without accounting for their interdependence as they operate across multiple levels (e.g., individual, social, health system; Page, Atkinson, Heffernan et al., 2018). By contrast, SDM, underpinned by the mathematical theory of nonlinear dynamics (Homer et al., 2006), can model multiple interacting and interrelated suicide risk factors (dynamic complexity) to understand likely trajectories of suicidal behavior while considering the complexity of health systems and the influence of factors such as capacity, access, and workforce (Atkinson et al., 2015, 2018). SDM allows us to simulate the impacts of alternative interventions or policies and thereby determine what works, for which groups of people, how, for how long, and why (Atkinson et al., 2020; Occhipinti et al., 2021). This is particularly appealing to policy-makers as they can experiment with, and optimize, different “what-if” scenarios to understand how interventions work within the system before implementing decisions in the real world (Atkinson et al., 2020).SDM is guided by a participatory approach to model building, evaluation, and implementation (Freebairn et al., 2022; Lee et al., 2022; Zimmerman et al., 2016). As such, it is an interdisciplinary methodological approach highly suitable (if not necessary) when addressing complex phenomena. SDM brings together a diverse group of stakeholders across different disciplines and types of expertise (e.g., those with lived and living experience of self-harm/suicidal behavior, carers, healthcare professionals, data scientists, service commissioners, and policy-makers) to collaboratively identify local needs and priorities. Stakeholders can offer unique knowledge of how the system operates, weaknesses in the system that could be used as leverage points, and how the system could be optimized through different policies, interventions, or service planning decisions (Freebairn et al., 2022; Lee et al., 2022; Occhipinti et al., 2021). Stakeholder insight into contextual barriers and facilitators for the translation of interventions into practice can guide the development of locally relevant implementation strategies (Zimmerman et al., 2016). A participatory modeling approach allows for transparency in how models are conceptualized, developed, and evaluated, thus increasing model credibility and confidence in the findings (Lee et al., 2022). Novel frameworks guiding the documentation of stakeholder engagement and evaluation of participatory modeling methods for SDM in youth mental health and suicide prevention are now available (Lee et al., 2022). Such frameworks are highly transferable and allow for the systematic assessment of the feasibility, value, impact, and sustainability of participatory modeling methods for developing system dynamics models (Lee et al., 2022). There is a need to use approaches that span disciplinary boundaries and facilitate the meaningful involvement of those with lived and living experience in the design and rollout of suicide prevention research; yet we continue to work in silos, and suicide prevention is often considered an issue for specialist mental health services (Michail et al., 2020). Through a participatory modeling approach, SDM offers a paradigm shift by “democratizing expertise” (Nowotny, 2003) and empowering the meaningful engagement of those with experiential knowledge of self-harm and suicide.One of the unique advantages of SDM is the fact that it is grounded in the principles of implementation science. It therefore maximizes opportunities for policy adoption and uptake in the real world (Atkinson et al., 2020). There have been consistent calls to close the gap between research, practice, and policy in the field of suicide prevention (Reifels et al., 2022). Despite available guidance for the implementation of national suicide prevention strategies (WHO, 2021), there remains a lack of planning for the systematic uptake and translation of such strategies in the real world (Reifels et al., 2022). The participatory approach inherent to system dynamics model development, evaluation, and refinement has the unique potential to advance the uptake of evidence-based interventions by working with stakeholders to identify contextual determinants that can impact on policy adoption before rollout (Freebairn et al., 2016, 2022; Zimmerman et al., 2016).Challenges in Adopting SDM for Suicide PreventionThe wide-scale adoption of SDM in suicide prevention remains slow due to several factors. SDM models have been criticized for failing to represent reality and, therefore, making unsubstantiated predictions about the future (Featherston & Doolan, 2012). SDM models require large numbers of data not only to build the initial model, but also for parameter calibration (i.e., finding a unique set of values that best describe the system behavior). Collecting the necessary data to inform model calibration can be time-consuming. Additionally, the quality of the available data can affect the accuracy of the final model. The appropriateness and robustness of modeling assumptions has been raised as a concern in predictive modeling (Glozier et al., 2023; Ioannidis et al., 2020) even among supporters of SDM. Sterman (2002), for example, highlighted the importance of thorough model testing, not just in relation to parameter sensitivity, but also to evaluate the robustness of underlying assumptions. Assumptions and parameter estimates may also need to be revised and models re-calibrated and re-validated as new data become available (Glozier et al., 2023). This is particularly relevant to the field of suicide prevention (Glozier et al., 2023; Ioannidis et al., 2020).SDM models are typically complex, representing multiple different pathways toward a common endpoint (Featherston & Doolan, 2012). Although this reflects the real-world complexity, and modeling this dynamic complexity is a key advantage of SDM over traditional methods (Homer & Hirsch, 2006), it can be difficult to identify the optimal point(s) within the system to intervene where an SDM model is overly complex. In part due to this complexity, SDM models can also lack transparency from the perspective of end-users. Others, on the other hand, have criticized SDM for being reductionist (Keys, 1990), that is, failing to account for individual-level differences in behaviors and experiences.SDM models are also computationally intensive and often require significant technical expertise in mathematics and computer programming to develop. Many organizations may not have access to staff with the necessary expertise and skill set to build, validate, and calibrate these complex models. Additionally, many decision-makers in our field might be unfamiliar with the complexity of the methods used in SDM, making it challenging to communicate the results of the models, and impacting the likelihood of the use of these models for advocacy.Future DirectionsNovel interdisciplinary methodological approaches are needed to unpack the complexity behind suicide, improve suicide prevention and intervention efforts, and guide effective policy action whilst avoiding unintended consequences. The application of systems modeling to suicide prevention has the potential to address this call and offer policy-makers a robust decision support tool to inform strategic planning and resource allocation for suicide prevention. However, there are a number of considerations if we are to unleash the full potential of SDM for suicide prevention. Indeed, before embarking on SDM we recommend researchers determine whether SDM approaches are appropriate to address their research question(s). To assist with this, the International Society for Pharmacoeconomics and Outcomes Research taskforce has developed the SIMULATE checklist – System, Interactions, Multilevel, Understanding, Loops, Agents, Time, Emergence (Marshall et al., 2015).This eight-point checklist helps researchers decide whether dynamic simulation modeling is appropriate to their research and identify those key elements (e.g., feedback loops, nonlinear interacting components within a system) that require complex simulation modeling.During the model building stage, the focus should be on the most important variables and relationships to identify those strategies likely to deliver the greatest benefit for suicide prevention to reduce model complexity. This process should be conducted in partnership with multiple stakeholders, including service commissioners and policy-makers who have the potential to change systems, healthcare providers, community leaders, educators, nongovernmental organizations, those with lived and living experience of suicide, as well as mathematicians and computer programmers who bring their expertise to help build, validate, and calibrate model. This will also help to ensure that decision-makers feel invested in the process and are provided with the knowledge and support they need to use the insights generated by the SDM to advocate for change. We acknowledge that bringing together stakeholders from diverse backgrounds, with different (and often competing) epistemological perspectives and priorities can be challenging. Managing power dynamics, successfully incorporating the technical aspects of research (e.g., modeling) and the experiential knowledge of those with lived and living experience are only some of the challenges researchers face when seeking to meaningfully involve a diverse group of stakeholders in their research (Bryson et al., 2004; Crompton et al, 2018). However, stakeholders matter (Bryson et al., 2004). Working collaboratively with stakeholders to co-produce protocols and procedures that would enable their meaningful engagement in suicide prevention research is necessary (Freebairn et al., 2022).Next, and before predictions can be made, time should be spent in validating the SDM model using reliable and routinely collected historic data wherever available, for example, data on suicide deaths as recorded by a national agency, in order to ensure the model can reproduce effects on this outcome(s). All too often, suicide prevention initiatives are implemented with inadequate evaluation of their impacts on concrete outcomes, such as per capita rates of self-harm and suicide (Kalafat, 2002). Therefore, it can be challenging to obtain the necessary data to inform the inputs to use for SDM in this field. There is therefore a need for a considerable investment in funding to enhance collaboration between researchers and grassroots and other nongovernment organizations in order to ensure that all novel interventions developed for suicide prevention can be robustly implemented and evaluated to bridge this knowledge gap (Platt & Niederkrotenthaler, 2020). Where certain assumptions have to be made, due to, for example, a lack of relevant and good-quality data, these assumptions should be thoroughly documented in publicly available data supplements. Examples of good practice in the field of suicide prevention can be used as a guide (Occhipinti et al., 2021; Skinner et al., 2021). Predictions made from SDM should be appropriately nuanced and thoroughly evaluated to determine whether the model accurately represents system behavior (Barlas, 1996). Model validation is a key step in the model development process and necessary to enhance confidence in the robustness of the model and future predictions in any field (Barlas, 1996), including that of suicide prevention (Glozier et al., 2023).To further foster public trust and confidence in SDM, reporting quality tools should be developed. Adherence to similar standards has considerably improved transparency in the reporting of evidence syntheses, randomized controlled trials, health economics, and other fields.To enhance the value of SDM and address concerns that this approach fails to account for individual-level differences in behaviors and experiences (Keys, 1990), researchers could combine insights generated at the macro-level by SDM with those generated at the micro-level by agent-based models. Agent-based models are computational models that have the capacity to model complex individual-level behaviors and interactions to help us understand how systems can be influenced by individual human behavior and social dynamics (Cassidy et al., 2019).ConclusionSystems thinking encourages us to reconsider how we understand and conceptualize suicide and suicidal behavior. Adopting a whole-system approach to suicide prevention requires the use of analytical tools that span disciplinary boundaries and facilitate the meaningful involvement of stakeholders. SDM has the potential to provide researchers with a conduit through which they can unpack the dynamic complexity underlying suicidal behavior; and policy-makers with a robust tool for an evidence-informed approach to suicide prevention. When conducted in an open and transparent way, SDM and related simulation modeling approaches are likely to be of great benefit to public health research (Carey et al., 2015), including suicide prevention.Katrina WittMaria MichailReferencesAtkinson, J.-A., Page, A., Prodan, A., McDonnel, G., & Osgood, N. (2018). Systems modelling tools to support policy and planning. Lancet, 391(10126), 1158–1159. 10.1016/S0140-6736(18)30302-7 First citation in articleCrossref Medline, Google ScholarAtkinson, J.-A., Page, A., Wells, R., Milat, A., & Wilson, A. (2015). A modelling tool for policy analysis to support the design of efficient and effective policy responses for complex public health problems. Implementation Science, 10, Article 26. 10.1186/s13012-015-0221-5 First citation in articleCrossref Medline, Google ScholarAtkinson, J.-A., Skinner, A., Hackney, S., Mason, L., Heffernan, M., Currier, D., King, K., & Pirkis, J. (2020). Systems modelling and simulation to inform strategic decision making for suicide prevention in rural New South Wales (Australia). Australian & New Zealand Journal of Psychiatry, 54(9), 892–901. 10.1177/0004867420932639 First citation in articleCrossref Medline, Google ScholarBarlas, Y. (1996). Formal aspects of model validity and validation in system dynamics. System Dynamics Review, 12(3), 183–210. 10.1002/(SICI)1099-1727(199623)12:3<183::AID-SDR103>3.0.CO;2-4 First citation in articleCrossref, Google ScholarBryson, J. M. (2004). What to do when stakeholders matter. Public Management Review, 6(1), 21–53. 10.1080/14719030410001675722 First citation in articleCrossref, Google ScholarCarey, G., Malbon, E., Carey, N., Joyce, A., Crammond, B., & Carey, A. (2015). Systems science and systems thinking for public health: A systematic review of the field. BMJ Open, 5(12), Article e009002. 10.1136/bmjopen-2015-009002 First citation in articleCrossref, Google ScholarCassidy, R., Singh, N. S., Schiratti, P. R., Semwanga, A., Binyaruka, P., Sachingongu, N., Chama-Chiliba, C. M., Chalabi, Z., Borghi, J., & Blanchet, K. (2019). Mathematical modelling for health systems research: A systematic review of system dynamics and agent-based models. BMC Health Services Research, 19(1), Article 845. 10.1186/s12913-019-4627-7 First citation in articleCrossref, Google ScholarChung, S., Hovmand, P., McBride, A. M., & Joiner, T. (2022). Suicide attempts during adolescence: Testing the system dynamics of the interpersonal theory of suicide. Journal of Adolescence, 94(4), 628–641. 10.1002/jad.12051 First citation in articleCrossref Medline, Google ScholarCrompton, A., Waring, J., Roe, B., & O’Connor, R. (2018). Are we all on the same page? A qualitative study of the facilitation challenges associated with the implementation of deliberative priority-setting. Public Management Review, 20(11), 1623–1642. 10.1080/14719037.2017.1417463 First citation in articleCrossref, Google ScholarFeatherston, C. R., & Doolan, M. C. (2012, July 22–26). A critical review of the criticisms of system dynamics [Paper presentation]. 30th International Conference of the System Dynamics Society, St. Gallen, Switzerland. First citation in articleGoogle ScholarForrester, J. W., & Senge, P. M. (1980). Tests for building confidence in system dynamics models. In A. A. Legasto Jr.J. W. ForresterJ. M. Lyneis (Eds.), System dynamics. TIMS studies in the management sciences (pp. 209–228). North-Holland Publishing Company. First citation in articleGoogle ScholarFranklin, J. C., Ribeiro, J. D., Fox, K. R., Bentley, K. H., Kleiman, E. M., Huang X., Musacchio, K. M., Jaroszewski, A. C., Chang, B. P., & Nock, M. K. (2017). Risk factors for suicidal thoughts and behaviours: Meta-analysis of 50 years of research. Psychological Bulletin, 143(2), 187–232. 10.1037/bul0000084 First citation in articleCrossref Medline, Google ScholarFreebairn, L., Atkinson, J., Kelly, P., McDonnell, G., & Rychetnik, L. (2016). Simulation modelling as a tool for knowledge mobilisation in health policy settings: A case study protocol. Health Research Policy and Systems, 14, Article 71. 10.1186/s12961-016-0143-y First citation in articleCrossref Medline, Google ScholarFreebairn, L., Occhipinti, J., Huntley, S., & Hickie, I. B. (2022). Participatory methods for systems modelling of youth mental health: An implementation protocol. JIMR Research Protocol, 11(2), Article e32988. 10.2196/32988 First citation in articleCrossref, Google ScholarGlozier, N., Morris, R., & Schurer, S. (2023). What happened to the predicted COVID-19-induced suicide epidemic, and why? Australian & New Zealand Journal of Psychiatry, 57(1), 11–16. 10.1177/00048674221131500 First citation in articleCrossref Medline, Google ScholarHawton, K., Saunders, K. E. A., & O'Connor, R. (2012). Self-harm and suicide in adolescents. Lancet, 379(9834), 2373–2382. 10.1016/S0140-6736(12)60322-5 First citation in articleCrossref Medline, Google ScholarHomer, J. B., & Hirsch, G. B. (2006). System dynamics modeling for public health: Background and opportunities. American Journal of Public Health, 96(3), 452–458. 10.2105/AJPH.2005.062059 First citation in articleCrossref Medline, Google ScholarIoannidis, J. P., Cripps, S., & Tanner, M. A. (2020). Forecasting for COVID-19 has failed. International Journal of Forecasting, 38(2), 423–438. 10.1016/j.ijforecast.2020.08.004 First citation in articleCrossref Medline, Google ScholarJeong, H., & Adamowski, J. (2016). A system dynamics based socio-hydrological model for agricultural wastewater reuse at the watershed scale. Agricultural Water Management, 171, 89–107. 10.1016/j.agwat.2016.03.019 First citation in articleCrossref, Google ScholarKalafat, J. (2002). Issues in the evaluation of youth suicide prevention initiatives. In T. JoinerM. D. Rudd (Eds.), Suicide science: Expanding the boundaries (pp. 241–249). Kluwer Academic Publishers. First citation in articleCrossref, Google ScholarKeys, P. (1990). System dynamics as a systems-based problem-solving method. Systems Practice, 3(5), 479–493. 10.1007/BF01064156 First citation in articleCrossref, Google ScholarLee, G., Hickie I. B., Occhipinti, J., Song, Y., Camacho, S., Skinner, A., Lawson, K., Hockey, S., Hilber, A., & Freebairn, L. (2022). Participatory systems modelling for youth mental health: An evaluation study applying a comprehensive multi-scale framework. International Journal of Environmental Research and Public Health, 19(7), Article 4015. 10.3390/ijerph19074015 First citation in articleCrossref, Google ScholarLiu, H., Benoit, G., Liu, T., Liu, Y., & Guo, H. (2015). An integrated system dynamics model developed for managing lake water quality at the watershed scale. Journal of Environmental Management, 155, 11–23. 10.1016/j.jenvman.2015.02.046 First citation in articleCrossref Medline, Google ScholarMarshall, D. A., Burgos-Liz, L., IJzerman, M. J., Osgood, N. D., Padula, W. V., Higashi, M. K., Wong, P. K., Pasupathy, K. S., & Crown, W. (2015). Applying dynamic simulation modeling methods in healthcare delivery research—the SIMULATE checklist: Report of the ISPOR simulation modeling emerging good practices task force. Value in Health, 18(1), 5–16. 10.1016/j.jval.2014.12.001 First citation in articleCrossref Medline, Google ScholarMeadows, D. H. (2008). Thinking in systems: A primer. Earthscan. First citation in articleGoogle ScholarMichail, M., Mughal, M., & Robinson, J. (2020). Suicide prevention in young people: Optimising primary care. British Journal of General Practice, 70(692), 104–105. 10.3399/bjgp20X708329 First citation in articleCrossref Medline, Google ScholarNowotny, H. (2003). Democratising expertise and socially robust knowledge. Science and Public Policy, 30(3), 151–156. 10.3152/147154303781780461 First citation in articleCrossref, Google ScholarOcchipinti, J.-A., Rose, D., Skinner, A., Rock, D., Song, Y. J. C., Prodan, A., Rosenberg, S., Freebairn, L., Vacher, C., & Hickie, I. B. (2022). Sound decision making in uncertain times: Can systems modelling be useful for informing policy and planning for suicide prevention? International Journal of Environmental Research and Public Health, 19(3), Article 1468. 10.3390/ijerph19031468 First citation in articleCrossref, Google ScholarOcchipinti, J.-A., Skinner, A., Doraiswamy, P., Fox, C., Herrman, H., Saxena, S., London, E., Song, Y., & Hickie, I. B. (2021). Mental health: Build predictive models to steer policy. Nature, 597, 633–636. 10.1038/d41586-021-02581-9 First citation in articleCrossref Medline, Google ScholarOcchipinti, J.-A., Skinner, A., Iorfino, F., Lawson, K., Sturgess, J., Burgess, W., Davenport, T., Hudson, D., & Hickie, I. (2021).Reducing youth suicide: Systems modelling and simulation to guide targeted investments across the determinants. BMC Medicine, 19(1), Article 61. 10.1186/s12916-021-01935-4 First citation in articleCrossref Medline, Google ScholarPage, A., Atkinson, J. A., Campos, W., Heffernan, M., Ferdousi, S., Power, A., McDonnell, G., Maranan, N., & Hickie, I. B. (2018). A decision support tool to inform local suicide prevention activity in Greater Western Sydney (Australia). Australian & New Zealand Journal of Psychiatry, 52(10), 983–993. 10.1177/0004867418767315 First citation in articleCrossref Medline, Google ScholarPage, A., Atkinson, J. A., Heffernan, M., McDonnell, G., & Hickie, I. B. (2017). A decision-support tool to inform Australian strategies for preventing suicide and suicidal behaviour. Public Health Research and Practice, 27(2), Article e2721717. 10.17061/phrp2721717 First citation in articleCrossref Medline, Google ScholarPage, A., Atkinson, J. A., Heffernan, M., McDonnell, G., Prodan, A., Osgood, N., & Hickie, I. B. (2018). Static metrics of impact for a dynamic problem: The need for smarter tools to guide suicide prevention planning and investment. Australian and New Zealand Journal of Psychiatry, 52(7), 660–667. 10.1177/0004867417752866 First citation in articleCrossref Medline, Google ScholarPlatt, S., & Niederkrotenthaler, T. (2020). Suicide prevention programs. Evidence base and best practice. Crisis, 41(Suppl 1), S99–S124. 10.1027/0227-5910/a000671 First citation in articleLink, Google ScholarReifels, L., Krishnamoorthy, S., Kõlves, K., & Francis, J. (2022). Implementation science in suicide prevention, Crisis 43(1), 1–7. 10.1027/0227-5910/a000846 First citation in articleLink, Google ScholarRogers, M. L., & Joiner, T. (2019). Exploring the temporal dynamics of the interpersonal theory of suicide constructs: A dynamic systems modeling approach. Journal of Consulting and Clinical Psychology, 87(1), 56–66. 10.1037/ccp0000373 First citation in articleCrossref Medline, Google ScholarSkinner, A., Occhipinti, J., Song, Y., & Hickie I., (2021). Regional suicide prevention planning: A dynamic simulation modelling analysis. British Journal of Psychiatry Open, 7(5), Article E157. 10.1192/bjo.2021.989 First citation in articleCrossref, Google ScholarSterman, J. D. (2002). All models are wrong: Reflections on becoming a systems scientist. System Dynamics Review 18(4), 501–531. 10.1002/sdr.261 First citation in articleCrossref, Google ScholarWorld Health Organization. (2012). Public health action for the prevention of suicide: A framework. https://apps.who.int/iris/handle/10665/75166 First citation in articleGoogle ScholarWorld Health Organization. (2018). National suicide prevention strategies: Progress, examples and indicators. https://www.who.int/publications/i/item/national-suicide-prevention-strategies-progress-examples-and-indicators First citation in articleGoogle ScholarWorld Health Organization. (2021). Suicide worldwide in 2019: Global health estimates. https://www.who.int/publications/i/item/9789240026643 First citation in articleGoogle ScholarYip, P. S. F., Zheng, Y., & Wong, C. (2022). Demographic and epidemiological decomposition analysis of global changes in suicide rates and numbers over the period 1990–2019. Injury Prevention, 28(2), 117–124. 10.1136/injuryprev-2021-044263 First citation in articleCrossref Medline, Google ScholarZimmerman, L., Lounsbury, D. W., Rosen, C. S., Kimerling, R., Trafton, J. A., & Lindley, S. E. (2016). Participatory system dynamics modelling: Increasing stakeholder engagement and precision to improve implementation planning in systems. Administration and Policy in Mental Health, 43(6), 834–849. 10.1007/s10488-016-0754-1 First citation in articleCrossref Medline, Google ScholarFiguresReferencesRelatedDetails Volume 44Issue 4July 2023ISSN: 0227-5910eISSN: 2151-2396 Published onlineAugust 4, 2023 InformationCrisis (2023), 44, pp. 261-266 https://doi.org/10.1027/0227-5910/a000905.© 2023Hogrefe PublishingPDF download Funding: While working on this editorial, Maria Michail was supported by a grant from the European Union H2020-EU.1.3.2. MSCA-IF-GF—Global Fellowships (Grant agreement ID: 101026065). Katrina Witt was supported by an Emerging Leader one Investigator Grant awarded by the Australian National Health and Medical Research Council (1177787).
更多查看译文
关键词
suicide prevention,systems modeling,policy-making
AI 理解论文
溯源树
样例
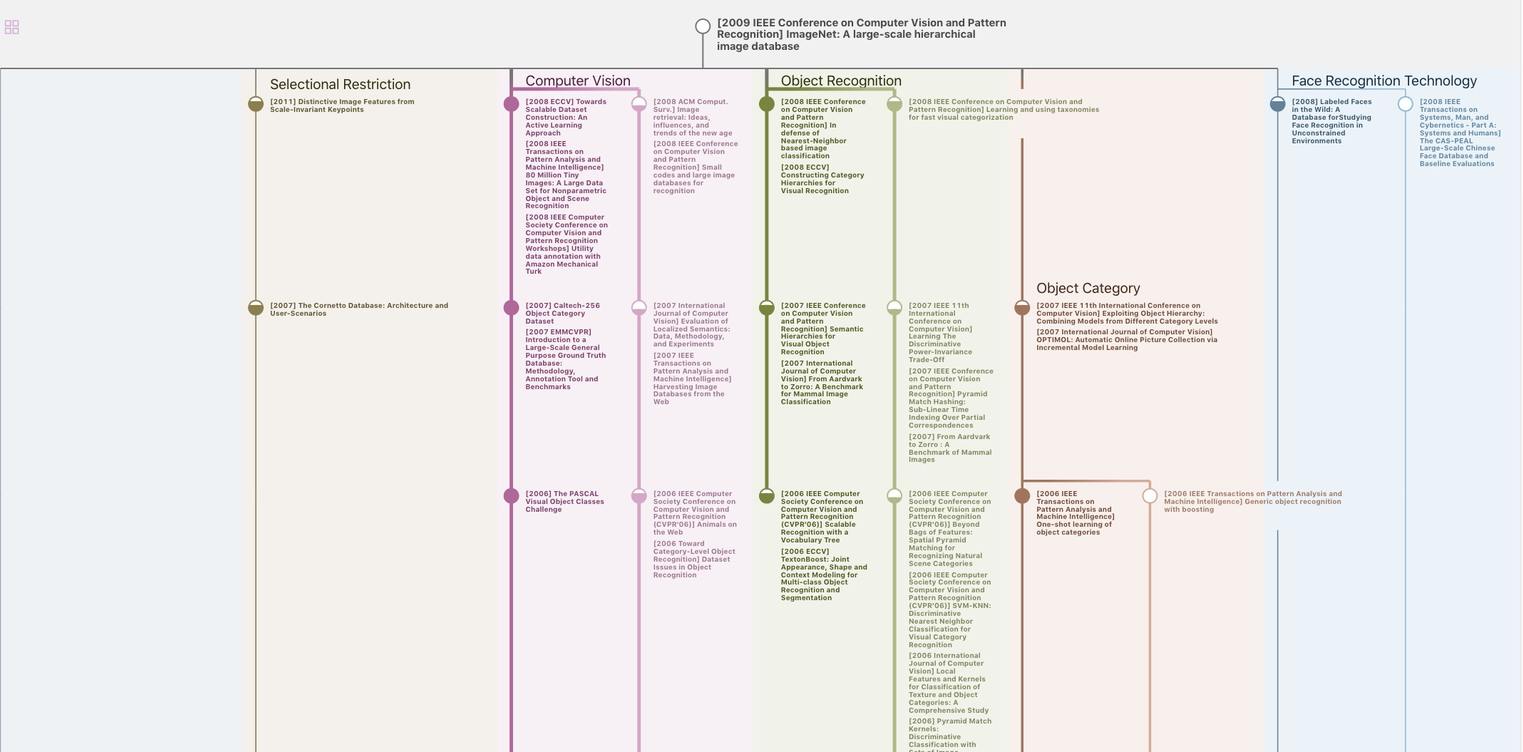
生成溯源树,研究论文发展脉络
Chat Paper
正在生成论文摘要