Lowbit Neural Network Quantization for Speaker Verification
2023 IEEE International Conference on Acoustics, Speech, and Signal Processing Workshops (ICASSPW)(2023)
摘要
With the continuous development of deep neural networks (DNN) in recent years, the performance of speaker verification systems has been significantly improved with the application of Deeper ResNet architectures. However, these deeper models occupy more storage space in application. In this paper, we adopt Alternate Direction Methods of Multipliers (ADMM) to realize low-bit quantization on the original ResNets. Our goal is to explore the maximal quantization compression without evident degradation in model performance. We implement different uniform quantization for each convolution layer to achieve mixed precision quantization of the entire model. Moreover, the impact of batch normalization layers in ADMM training and layer sensibility to quantization are explored. In our experiments, the 8 bit quantized ResNetl52 achieved comparable results to the full-precision one on Voxceleb 1, with only 45% of original model size. Besides, we find that shallow convolution layers are more sensitive to quantization. In addition, experimental results indicate that the model performance will be severely degraded if batch normalization layers are integrated into the convolution layer before the quantization training starts.
更多查看译文
关键词
speaker verification, neural network quantization, model compression, mixed precision quantization
AI 理解论文
溯源树
样例
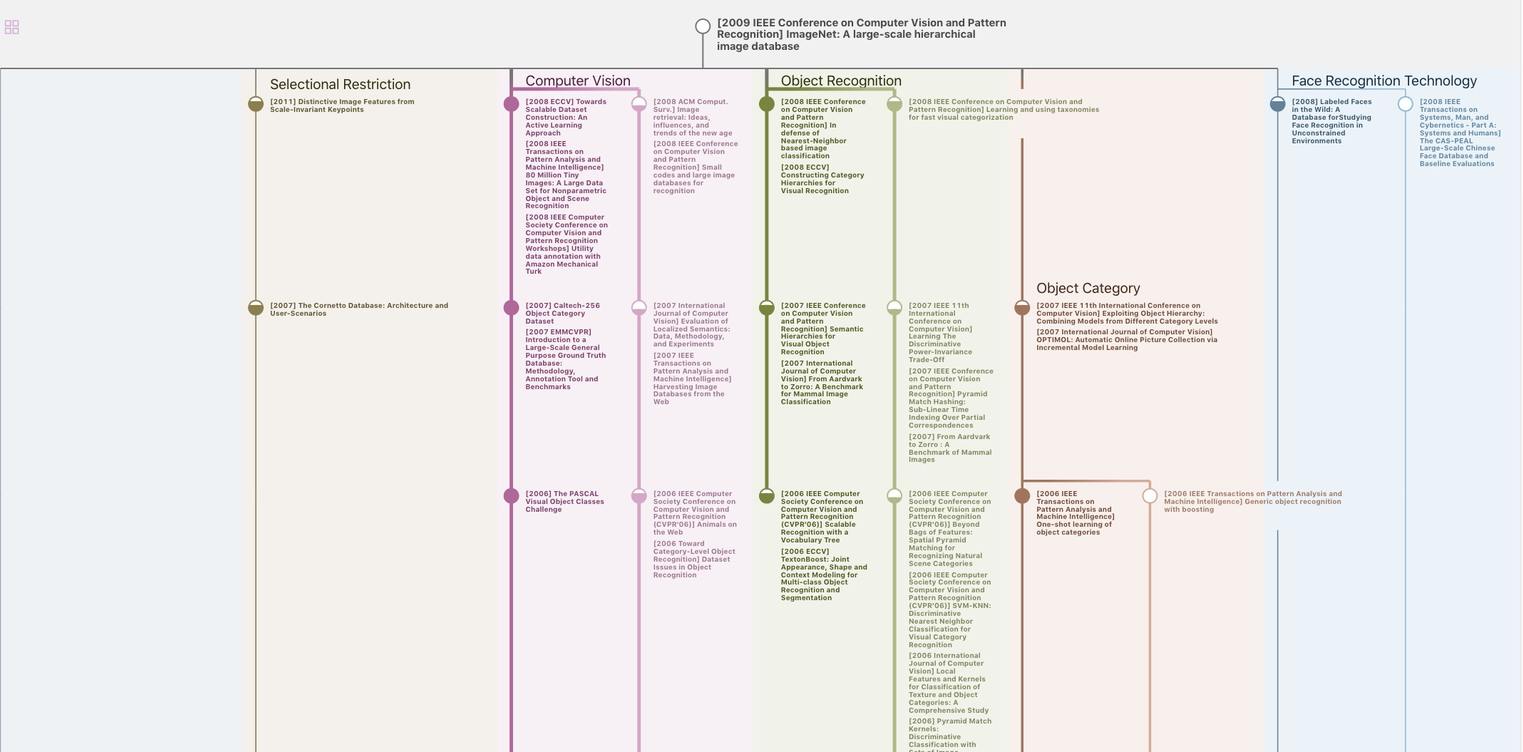
生成溯源树,研究论文发展脉络
Chat Paper
正在生成论文摘要