Deep Learning-Based Modulation Classification for OFDM Systems Without Symbol-Level Synchronization
2023 IEEE International Conference on Acoustics, Speech, and Signal Processing Workshops (ICASSPW)(2023)
摘要
Deep learning (DL)-based modulation classification of incoherently received orthogonal frequency division multiplexing (OFDM) signals is studied. We propose a novel preprocessing algorithm to build features characterizing the modulation of OFDM signals, which are insensitive to synchronization error. With obtained features, pilot subcarrier indices used for CFO correction may also be estimated. The features obtained with the proposed algorithm are classified with a convolutional neural network (CNN)-based classifier. We have evaluated classification performance with simulated and hardware-generated data. Using these features, the modulation classifier outperforms existing DL-based classifiers which assume symbol-level synchronization with up to 25% classification accuracy performance gain.
更多查看译文
关键词
Modulation Classification, Deep Learning, OFDM
AI 理解论文
溯源树
样例
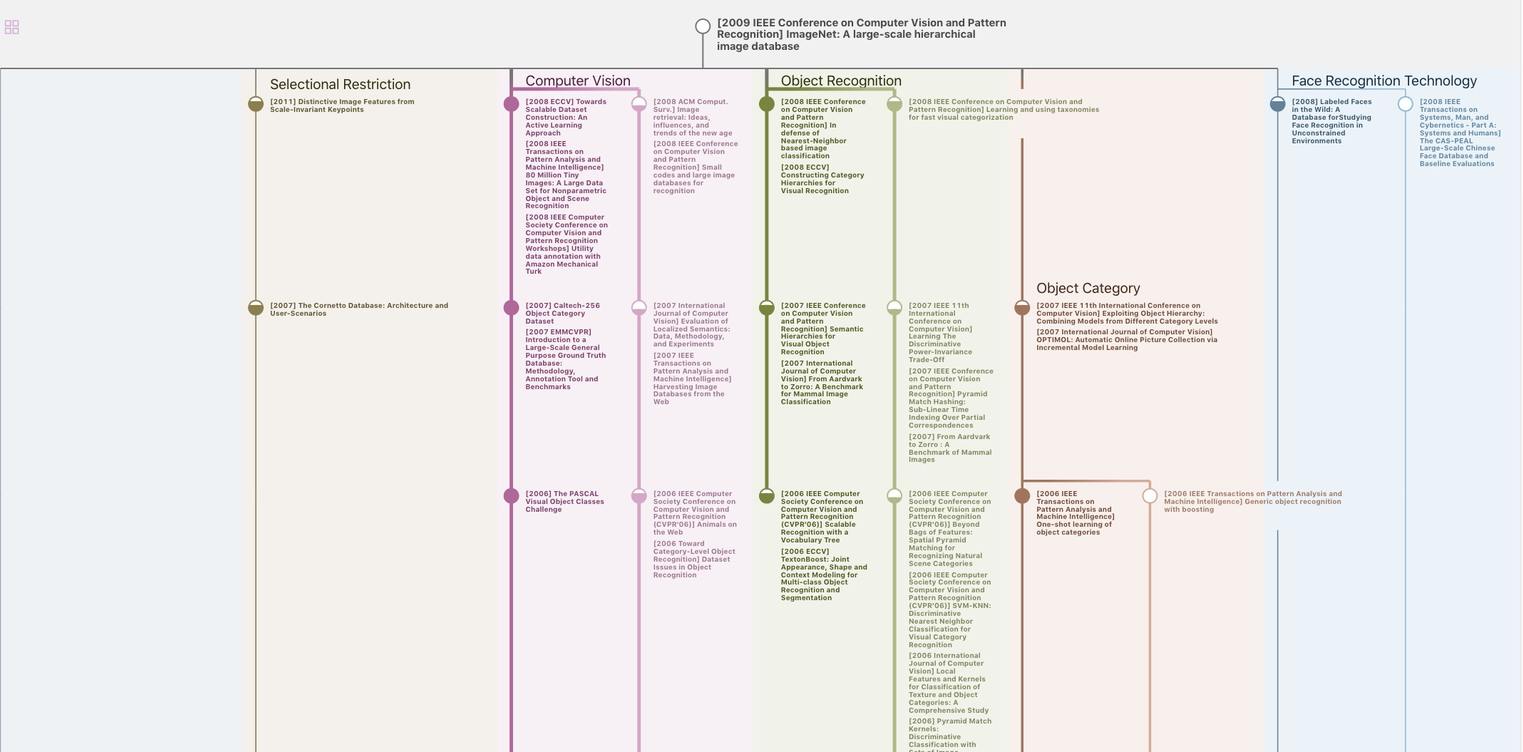
生成溯源树,研究论文发展脉络
Chat Paper
正在生成论文摘要