Improving Dino-Based Self-Supervised Speaker Verification with Progressive Cluster-Aware Training
2023 IEEE International Conference on Acoustics, Speech, and Signal Processing Workshops (ICASSPW)(2023)
摘要
Self-supervised contrastive learning has recently emerged as one of the promising approaches in speaker verification task, due to its independence from labeled data. Among them, the DINO-based self-supervised framework, trained without exploiting negative pairs, is very popular and achieves excellent performance in the speaker verification task. However, limited by the duration of utterance, there exist many overlaps which may mislead the model to pay attention to irrelevant information. To tackle this problem, we propose a cluster-aware (CA) training strategy to make the model crop positive segments from several utterances in the same cluster rather than from a single utterance. Besides, in the clustering stage, we also investigate strategies of fixed number clustering as well as progressive clustering. With these strategies, our CA-DINO achieves the state-of-the-art result on Vox-O test set. Finally, we explore the effect of fine-tuning CA-DINO with a small amount of labeled data. Our proposed model with only 10% labeled data outperforms the fully supervised system trained on all data.
更多查看译文
关键词
speaker verification, self-supervised, dino, cluster-aware, progressive clustering
AI 理解论文
溯源树
样例
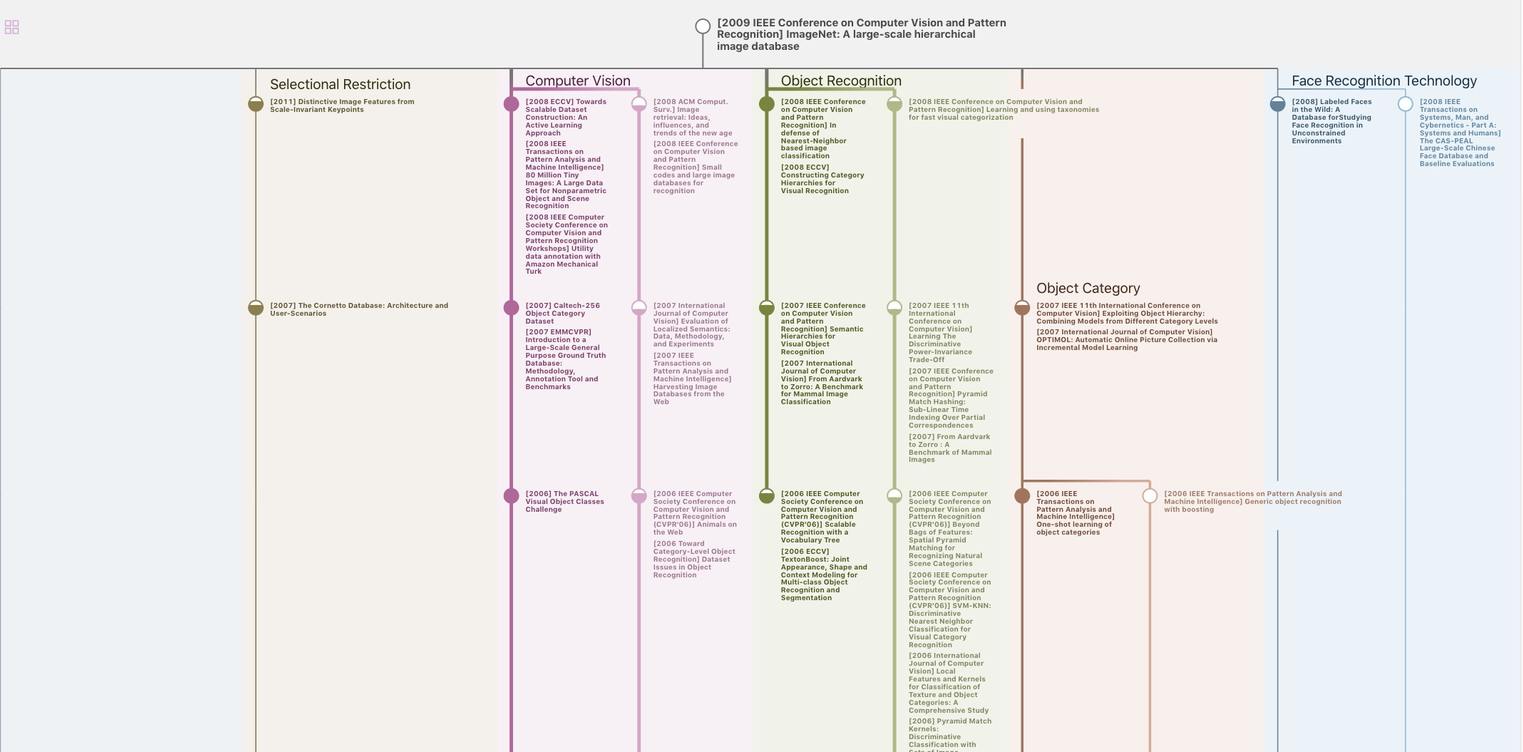
生成溯源树,研究论文发展脉络
Chat Paper
正在生成论文摘要