A new NIALM system design based on neural network architecture and adaptive springy particle swarm optimization algorithm
Energy Efficiency(2023)
摘要
A recent research interest in demand-side management technology of smart grids is to know what appliances are operating in a home, by giving only the power signals on the main feeder at the entrance of the home. Obtaining knowledge about power signals is possible by replacing old electro-mechanical electricity meters with automatic sensing. This paper presents an intelligent non-intrusive appliance load monitoring (NIALM) design for disaggregating appliances in a power system. A new loads disaggregation methodology is presented based on the self-recurrent radial basis function neural network (SRRBFNN) model that uses an adaptive springy particle swarm optimization (ASPSO) algorithm to optimize the parameters of the SRRBFNN model. In the proposed method, the SRRBFNN identifies appliance loads, determining if they are turned ON or OFF, based on the analysis of load signatures. A feature vector is built via an optimized MinMax Steady-State (MMSS) algorithm and a step-changes identifying unit. The step-changes identifying unit is an event detector capable of detecting the step-changes of input signals whenever an appliance is turned ON or OFF. Compared with the classical PSO (CPSO), the ASPSO shows a superior performance since it utilizes an adaptive procedure through two additional units. The two units follow an updating and a screening mechanism, intending to improve the CPSO performance after trapping on a local optimum. The experimental results indicate that the proposed methodology for solving the NIALM based on ASPSO and SRRBFNN significantly improves the loads’ classification accuracy.
更多查看译文
关键词
Non-intrusive appliance load monitoring system (NIALM), Self-recurrent radial basis function neural network (SRRBFNN), Adaptive springy partial swarm optimization (ASPSO), Load signatures
AI 理解论文
溯源树
样例
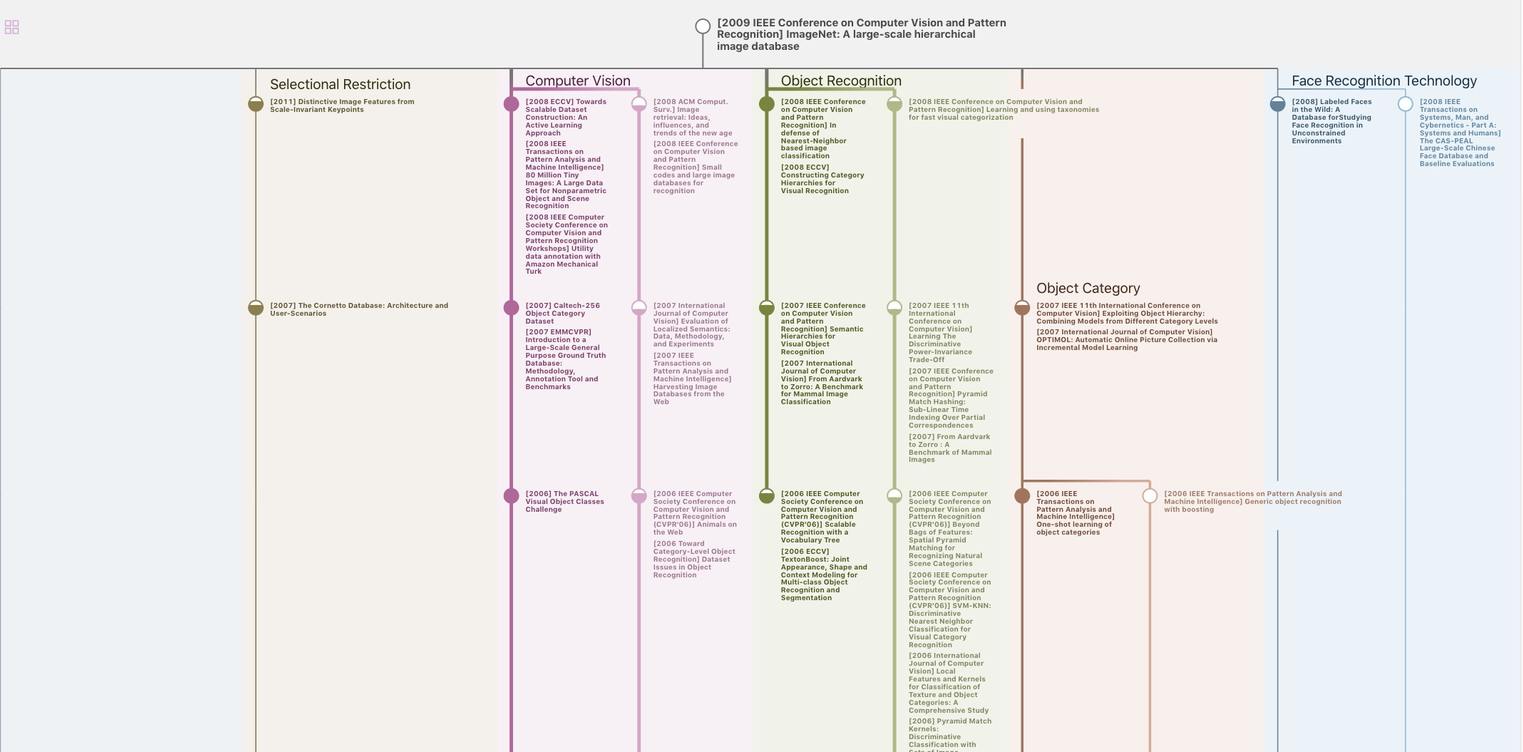
生成溯源树,研究论文发展脉络
Chat Paper
正在生成论文摘要