Balanced Classification: A Unified Framework for Long-Tailed Object Detection
IEEE TRANSACTIONS ON MULTIMEDIA(2024)
摘要
Conventional detectors suffer from performance degradation when dealing with long-tailed data due to a classification bias towards the majority head categories. In this article, we contend that the learning bias originates from two factors: 1) the unequal competition arising from the imbalanced distribution of foreground categories, and 2) the lack of sample diversity in tail categories. To tackle these issues, we introduce a unified framework called BAlanced CLassification (BACL), which enables adaptive rectification of inequalities caused by disparities in category distribution and dynamic intensification of sample diversities in a synchronized manner. Specifically, a novel foreground classification balance loss (FCBL) is developed to ameliorate the domination of head categories and shift attention to difficult-to-differentiate categories by introducing pairwise class-aware margins and auto-adjusted weight terms, respectively. This loss prevents the over-suppression of tail categories in the context of unequal competition. Moreover, we propose a dynamic feature hallucination module (FHM), which enhances the representation of tail categories in the feature space by synthesizing hallucinated samples to introduce additional data variances. In this divide-and-conquer approach, BACL sets a new state-of-the-art on the challenging LVIS benchmark with a decoupled training pipeline, surpassing vanilla Faster R-CNN with ResNet-50-FPN by 5.8% AP and 16.1% AP for overall and tail categories. Extensive experiments demonstrate that BACL consistently achieves performance improvements across various datasets with different backbones and architectures.
更多查看译文
关键词
Tail,Detectors,Training,Object detection,Feature extraction,Head,Task analysis,Long-tailed object detection,Long-short-term indicators pair,Foreground classification balance loss,Feature hallucination module
AI 理解论文
溯源树
样例
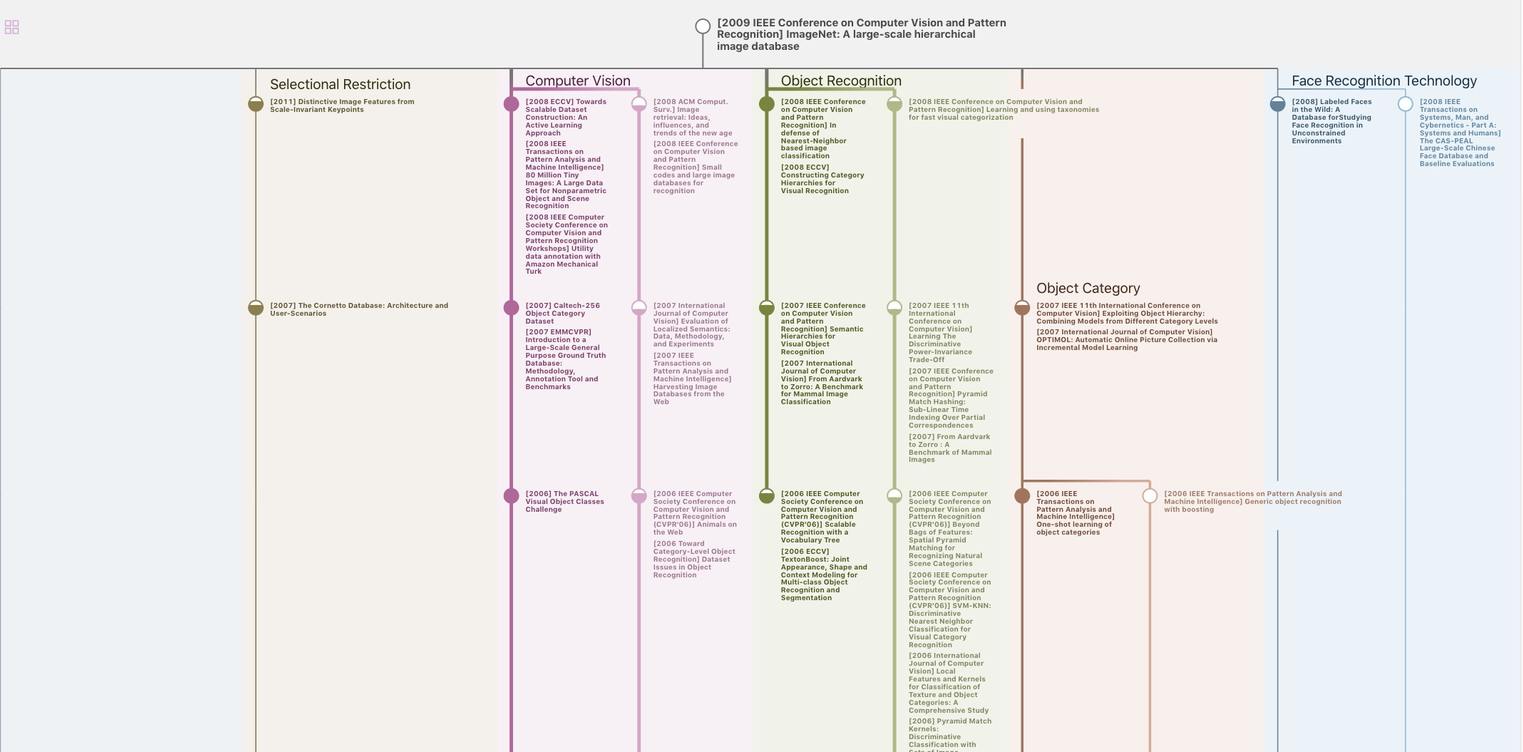
生成溯源树,研究论文发展脉络
Chat Paper
正在生成论文摘要