Likelihood-ratio-based confidence intervals for neural networks
CoRR(2023)
摘要
This paper introduces a first implementation of a novel likelihood-ratio-based approach for constructing confidence intervals for neural networks. Our method, called DeepLR, offers several qualitative advantages: most notably, the ability to construct asymmetric intervals that expand in regions with a limited amount of data, and the inherent incorporation of factors such as the amount of training time, network architecture, and regularization techniques. While acknowledging that the current implementation of the method is prohibitively expensive for many deep-learning applications, the high cost may already be justified in specific fields like medical predictions or astrophysics, where a reliable uncertainty estimate for a single prediction is essential. This work highlights the significant potential of a likelihood-ratio-based uncertainty estimate and establishes a promising avenue for future research.
更多查看译文
关键词
confidence intervals,neural networks,likelihood-ratio-based
AI 理解论文
溯源树
样例
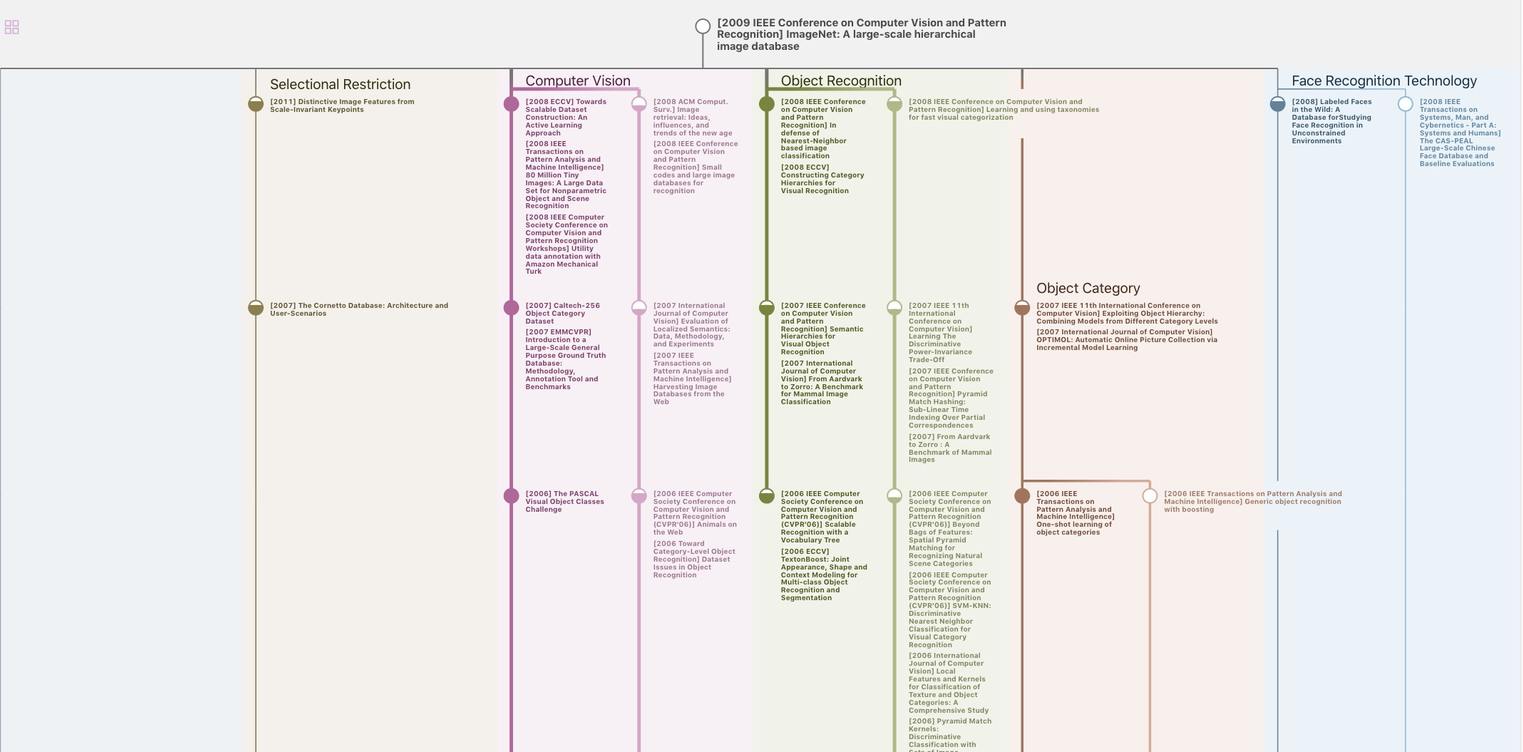
生成溯源树,研究论文发展脉络
Chat Paper
正在生成论文摘要