A Hybrid Continuous-Time Dynamic Graph Representation Learning Model by Exploring Both Temporal and Repetitive Information
ACM Transactions on Knowledge Discovery from Data(2023)
摘要
Recently, dynamic graph representation learning has attracted more and more attention from both academic and industrial communities due to its capabilities of capturing different real-world phenomena. For a dynamic graph represented as a sequence of timestamped events, there are two kinds of evolutionary essences: temporal and repetitive information. At present, the temporal information of interactions (e.g., timestamps) have been deeply explored. However, as another vital nature of dynamic graphs, the repetitive information of interactions between two nodes is neglected, which may lead to inaccurate node representation. To address this issue, we propose a novel continuous-time dynamic graph representation learning model, which consists of a node-level-memory based module, a historical high-order neighborhood based vertical aggregation module and a repetitive-topological information based horizontal aggregation module. In particular, to characterize the evolving pattern of the repetitive information of interactions between a pair of nodes, we put forward a repetitive-interaction based attention mechanism to integrate the two key attributes (i.e., the content and the number of interactions) of repetitive interactions at different moments, based on the insight that the repetitive behaviors of nodes are widespread and essential. We conduct extensive experiments including future link prediction tasks (for transductive and inductive learning) and dynamic node classification task, and results on three real-life dynamic graph datasets demonstrate that the proposed method significantly outperforms state-of-the-art baselines, for both observed nodes and new ones.
更多查看译文
关键词
Graph neural networks,continuous-time dynamic graph,representation learning
AI 理解论文
溯源树
样例
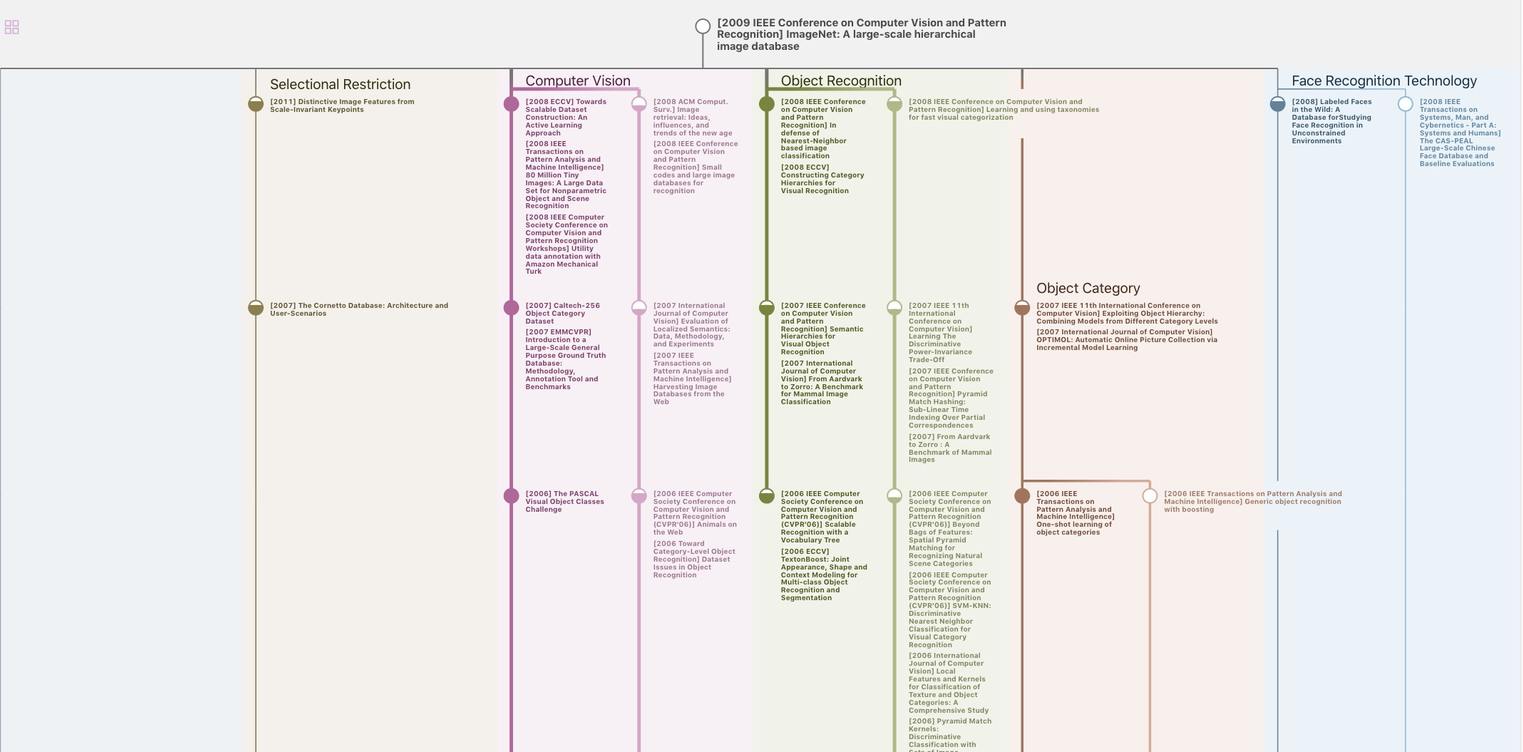
生成溯源树,研究论文发展脉络
Chat Paper
正在生成论文摘要