Surveying Federated Learning Approaches Through a Multi-Criteria Categorization
Multimedia tools and applications(2023)
摘要
In recent years, more and more attention has been paid to the privacy issues associated with storing user data in a centralized manner. In fact, strict laws have been introduced to regulate the use of personal data, making it more difficult to find enough data for training artificial intelligence models. As this new challenge arises, Federated Learning (FL) has been introduced to leverage user data without jeopardizing their privacy. To make FL possible, it was necessary to face several technical and algorithmic challenges. As a result, many different approaches have been proposed in recent years. In this survey, we begin by describing the FL approach and delving into both its advantages and challenges. Furthermore, a thorough analysis of various literature approaches is provided through the exploration of three key aspects characterizing them. Firstly, different network topologies proposed over the years are discussed in detail, with a focus on highlighting the benefits and drawbacks of each. Secondly, attention is given to the data distribution aspect, particularly focusing on the feature and sample spaces of the data, which may differ among participants. Lastly, potential security threats associated with FL are outlined, and the countermeasures proposed in the literature to address them are described in detail. By employing these analytical criteria, comparisons are made among the different approaches to provide a multi-criteria categorization of FL methodologies.
更多查看译文
关键词
Machine Learning,Federated Learning,Data Privacy
AI 理解论文
溯源树
样例
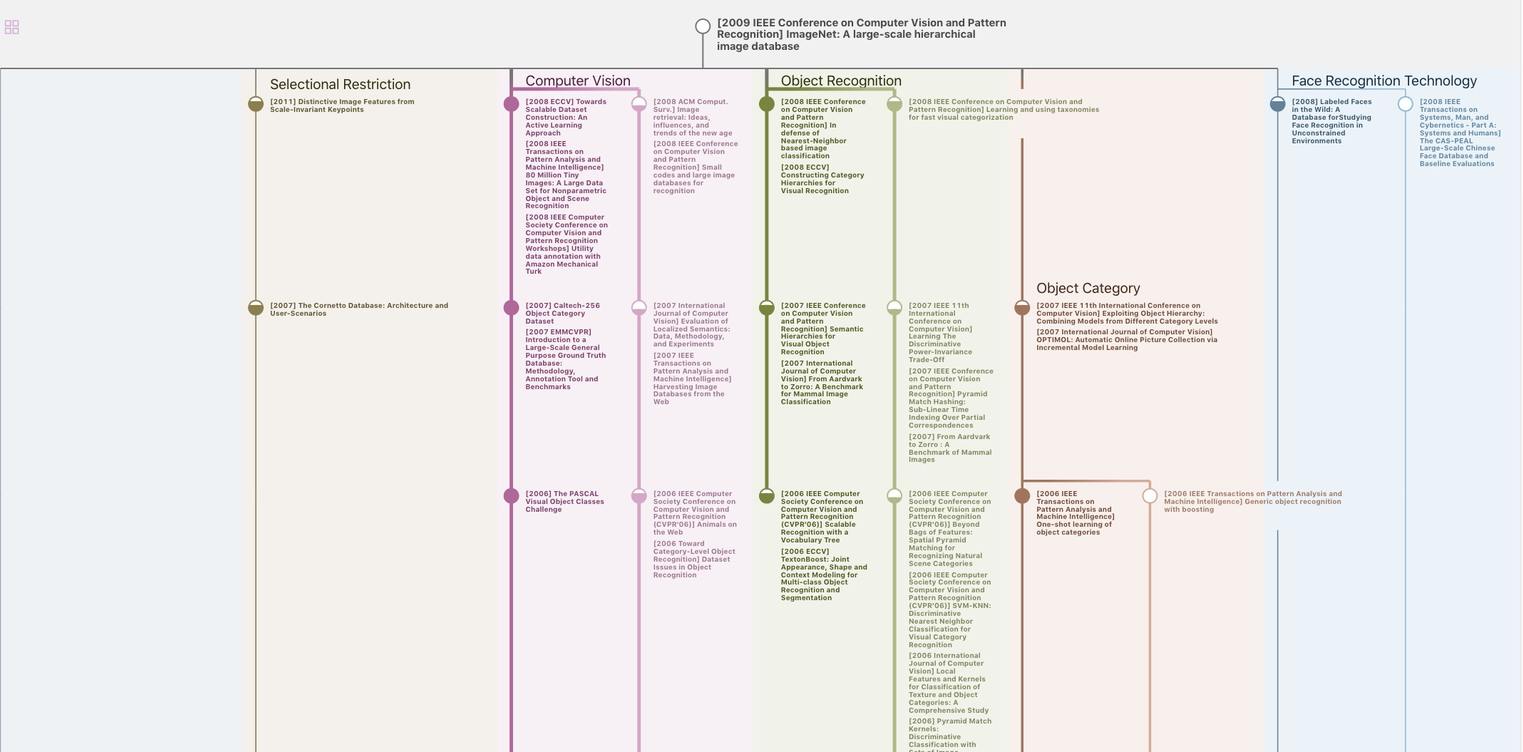
生成溯源树,研究论文发展脉络
Chat Paper
正在生成论文摘要